Sponsored by ORTECMay 10 2017
When Counting Statistics Dominates
For spectrometers measuring and counting individual events (Table 1), the precision for measuring the number of events is generally controlled by counting statistics. In the case of a small peak superimposed on a high background in the acquired spectrum, the precision of measuring the net peak counts is seriously degraded when there is a fluctuation in the background counts. Ultimately, it is this uncertainty in the background counts that establishes the detection limit for the peak.
Table 1. Typical Particles and Photons Associated with Counting Statistics
. |
. |
Ionized molecules |
Neutrons |
Molecules |
Electrons |
Ions (atomic or molecular) |
Beta particles |
Atomic nuclei |
Gamma rays |
Alpha particles |
X rays |
Protons |
IR to UV photons |
This article discusses the contribution of counting statistics to the uncertainty in identifying the net peak area, and in controlling detection limits. The method is applicable to spectrometers that count single events (Table 2). The results reveal the importance of maximizing the peak-to-background ratio, the event counting rate, and the counting time. The event counting rate and the counting time improve precision by increasing the count of measured counts.
Table 2. Spectrometers Dominated by Counting Statistics
- Time Digitizers used in Time-of-Flight Mass Spectrometry (TOF-MS)
- Time Digitizers or Multichannel Scalers (MCS) responding to single photons in LIDAR, or fluorescence and phosphorescence lifetime spectrometry
- Time-of-flight spectrometry with nuclear radiation (neutrons, alpha and beta particles, ions and nuclei)
- Pulse-amplitude or energy spectrometry with nuclear radiation (gamma-rays, X-rays, alpha particles, recoiling nuclei) using multichannel analyzers (MCA)
- Time spectrometry using a time-to-amplitude converter (TAC) followed by an MCA
|
Poisson Statistics Applies
The above applications normally fulfill the conditions that characterize the Poisson Distribution1, 2:
1) The events are randomly and evenly distributed over the sampling intervals
2) The probability of detecting an event during an infinitesimal time interval dt is ρdt, where ρ represents the expected counting rate
3) ρdt « 1
4) The probability of determining more than one event during the infinitesimal time interval dt is insignificant
If the events are counted over a finite time period (t), the Poisson Distribution (P(N)) explains the probability of recording N counts in a single measurement of duration (t).
.jpg) |
(1) |
If the measurement is repeated multiple times and the values of N are averaged, the average value of N approaches the mean of the distribution (µ) as the number of repeated measurements approaches infinity. Please note that the standard deviation of the Poisson Distribution is expressed as follows:
.jpg) |
(2) |
Substituting N for µ in Equation 2 reveals that the value of N from a single measurement is an adequately accurate estimate1 of µ. A more useful description of the precision of the measurement is obtained by multiplying the relative standard deviation, σN / N, by 100% to describe the percent standard deviation as
.jpg) |
(3) |
How the percent standard deviation improves with increasing counted number of events is shown in Table 3. Apparently, it is necessary to accumulate a large number of events to achieve a precision better than 1%.
Table 3. σN% for Selected Values of N
N |
σN% |
1
100
10,000
1,000,000 |
100.0%
10.0%
1.0%
0.1% |
Strictly speaking, Equations 1 through 3 accurately express the statistical distribution of events counted only if a) dead time losses are negligible, or b) if an ideal livetime clock is used to compensate for dead time losses3 – 8. Here, at least one of these conditions will be presumed to be met.
Optimum Precision for a Peak with Zero Background
Most of the spectrometers listed in Table 2 generate spectra consisting of widely separated peaks sitting on a slowly varying background. The position of the peak along the horizontal axis indicates the key parameter to be measured, including the mass of the particles in mass spectrometry, the flight time of the photons or particles in a time-of-flight spectrometer, or the energy of the photons or particles in pulse-height spectrometry. The centroid of the peak is normally used to mark the position of the peak and to yield an accurate measurement of the mass, flight time, or energy in the aforementioned examples.
The peak area measures the probability of determining events with that specific mass, flight time, or energy. A Gaussian peak shape is typically a good approximation to the actual shape of the peaks in the spectrum. As a result, it will be applied as a pragmatic model for examining the methods for extracting the optimum data from the spectrum.
The special case of zero background is useful to learn how to extract all the data from the peak in the more general case of non-zero background. Hence, the Gaussian peak as shown in Figure 1 is taken for consideration. The y axis denotes the number of events counted per unit interval on the x axis. Γ represents the Full Width at Half Maximum height (FWHM) of the peak. The dashed lines, located an equal distance to the right and left of the centroid, describe the peak area from which the counts will be extracted. ηP is the width of this region, and P represents the total number of counts defined by the area of this region. The total area of the peak (A) denotes the number of events counted in the entire peak.
.jpg)
Figure 1. Integrating the counts in a selected region of a Gaussian peak.
After summing the counts from entire area of the peak, the relative standard deviation in those counts is expressed as follows:
.jpg) |
(4) |
This is the best precision that can be obtained from the peak, because A represents the largest number of counts that can be extracted from the peak. The integration region of width ηP employs less than the total number of events counted in the peak, yielding a relative standard deviation as expressed below:
.jpg) |
(5) |
It is helpful to compare the precision derived using Equation 5 with the best precision that can be achieved by dividing Equation 5 by Equation 4.
.jpg) |
(6) |
Figure 2 shows the plot of Equation 6 versus the width of the integration region to the FWHM of the peak. The best precision that is achievable when the entire area of the peak is integrated is denoted by the horizontal dashed line in Figure 2. The precision degrades for narrower integration regions and the degradation escalates quickly when the integration region width approaches zero.
.jpg)
Figure 2. The figure of merit for precision in the peak counts versus the width of the integration region, ηP.
As can be seen in Figure 2, to achieve the best precision, the integration region width should be more than the double the FWHM of the peak. The condition, ηP = 2 Γ integrates 98% of the events counted in the peak. Wherever possible, it is advisable to have an even wider integration region to prevent errors caused by unintentional misalignment of the integration window relative to the centroid of the peak. Peak shifts due to changes in temperature or counting rate can cause misalignment. If the centroid of the peak is estimated calculated by the standard centroid algorithm9, an integration region wider than 2 Γ is important for preventing misalignment errors in the computed peak position. If the centroid is calculated using the entire area of the peak, the random error due to counting statistics is9, 13:
.jpg) |
(7) |
Where, σc represents the standard deviation in the x coordinate for the centroid of the peak. If it is not possible to use the entire peak to extract the centroid data, a least squares fit of the known peak shape to the central portion of the peak can be used for finding the peak position with reasonable accuracy2, 10, 11, 12. The same method can be applied to reduce misalignment errors when calculating the total area of the peak. For pedagogic simplicity, this article will presume a simple integration of the counts is used as shown in Figure 1. The random error controlling precision and detection limits is similar for both the methods, i.e., least squares fitting or simple integration.
Detection Limits with Zero Background
For a peak, detection limits are generally defined by setting a threshold that the counts in the peak must exceed to claim detection. The threshold is selected so that there is a 95% probability of the counts exceeding the threshold value if the peak is truly present in the spectrum. This defines the 95%-confidence limit, which implies that the probability of not detecting the peak is 5%, even though the peak is truly present.
A single count is the minimum that is detectable with zero background. Equation 1 is used to derive the value of µ for which there is a 95% probability of recording values higher than zero for N in a single measurement. This is identical to determining the value of µ for which there is a 5% probability of recording zero counts in a single measurement. Equation 8 expresses that probability, which is set equal to 0.05 to determine the value of µ corresponding to the detection limit.
.jpg) |
(8) |
The solution is
Therefore, a detection threshold set at N ≥ 1 yields a 95%-confidence detection limit at
The counts in the peak are normally related to the concentration C for the component of the material being measured by a calibration constant k.
This leads to the specification of the minimum detectable concentration for the case of zero background:
C denotes the concentration of the molecule with a mass ‘m’ in the original sample for mass spectrometry and represents the activity of the specific radioisotope for radioactive nuclide spectrometry.
If an initial attempt at the measurement yields detection limits that are not low enough, how to improve the detection limit? Increasing the counting time is the simplest solution. For the special case of zero background, when the counting time is doubled, the value of A will be doubled and the value of k will be decreased by a factor of 2 in Equation 11. This decreases CDL0 by a factor of 2 in Equation 12. In other words, detection limits are decreased by lowering the concentration required to yield an average of 3 counts. This can be achieved by a) increasing the counting time, and/or b) improving the yield of counts per unit concentration from the sample. Solution ‘b’ could help improve the efficiency of the measurement apparatus, or pre-concentration of the desired component in the sample.
A Gaussian Approximation for the Poisson Distribution
Moving to the general case of a peak sitting on a background requires subtraction of the background to detect the net peak counts. For this, it is necessary to develop the Gaussian approximation to a Poisson distribution in order to calculate the random error in the net peak counts. If the counts from two separate measurements are summed up:
and N1 and N2 are both defined by their own Poisson distributions, then N+ also has a Poisson distribution defined by1
.jpg) |
(14) |
Where,
µ+ is the sum of the means from the two distributions, as well as the mean for the distribution of the sum.
Using this relationship, the standard deviation of the sum can be easily calculated from:
.jpg) |
(16) |
However, the difference of two counts does not have such a simple relationship as the difference no longer follows a Poisson distribution1:
Here, one has to resort to the Gaussian approximation to a Poisson distribution. How the shape of the Poisson distribution changes with increasing mean value (µ) is shown in Figure 3. For values of µ nearing 1, the distribution is asymmetric. The distribution becomes more symmetric with increasing value of µ and begins to resemble a Gaussian shape. Poisson distributions for µ = 9 and µ = 36 to the Gaussian probability distribution are compared in Figure 4.
.jpg)
Figure 3. The Poisson probability distribution for various values of the mean, µ.
.jpg)
Figure 4. A comparison between the Poisson and Gaussian distributions for µ = σ2 = 9 and µ = σ2 = 36. The Poisson distributions are represented by the solid diamonds and squares, while the Gaussian distributions are plotted as continuous lines.
.jpg) |
(18) |
Where the special condition
has been substituted in Equation 18 to make the mean and standard deviation for the Gaussian curve match the mean and standard deviation of the Poisson distribution. The two distribution types have a good correspondence between them for a value of m as low as 9. The Gaussian distribution is an adequate approximation to the Poisson probability distribution for µ ≥ 9 for the purposes of computing and integrating standard deviations. The Gaussian probability distribution fulfills Equations (13), (15), and (16) for the distribution of the sum of counts from two Gaussian distributions. Actually, the distribution of N+ is also a Gaussian function. Moreover, the distribution of the difference of two counts:
has a Gaussian distribution, if the distributions of N1 and N2 are both Gaussian1. The mean of the distribution for N– is
But, the standard deviation for N– is
.jpg) |
(21) |
One has to note the crucial "+" signs in Equation 21 despite of the "–" signs in Equations 17 and 20. The results surrounding Equations 20 and 21 stem from the symmetry and shape of the Gaussian function.
The calculations in the next section need one more principle for obtaining standard deviations from functions incorporating Gaussian probability distributions. If N is distributed in accordance with a Gaussian probability function and ß is a constant, then
also has a Gaussian distribution with a standard deviation1, 2
Where, σN represents the standard deviation of the probability distribution for N.
The aforementioned equations for the Gaussian approximation are the tools required for determining the precision of the net peak counts for a peak sitting on a background.
Peak Precision in the Presence of Background
A simple approach for subtracting the background lying under a peak to obtain the net peak counts is illustrated in Figure 5. This is a practical algorithm whenever the background changes slowly enough in the vicinity of the peak to be approximated by a straight line spanning the position of the peak. A region of interest (ROI) of width ηP is marked on the peak, followed by the integration of the counts in that region. The total integrated area (NT) comprises of the background counts (B) and the net peak counts (P).
.jpg)
Figure 5. A simple method for background subtraction.
B is calculated by integrating two additional regions of interest, one is distance ‘d’ to the left of the peak ROI, and other one is distance d to the right of the peak ROI. For these background regions, the widths are set equal (ηB/2). The counts integrated from the left background region are designated NB1, whereas the counts summed in the right background region are designated NB2. As a result, the estimate of the background incorporated under the peak becomes:
.jpg) |
(25) |
With a standard deviation
.jpg) |
(26) |
Hence, it is possible to estimate the net peak counts by subtracting the background estimate via Equation 27.
In this estimate of the net peak counts, the standard deviation is:
.jpg) |
(28) |
Which simplifies to
.jpg) |
(29) |
Where, the first term ‘P’ within the square root sign is the contribution made by the peak counts to the precision if there were no background (when B = 0). The second term represents the degradation of the precision due to the presence of background. It is to be noted that the factor in the parentheses amplifies the background contribution.
Following the principle defined in Equation, the figure of merit for Equation 39 can be defined as:
.jpg) |
(30) |
Equation is actually Equation 6, but consists of an additional factor under the large square root sign that represents the degradation of precision caused by the statistical uncertainty in the background. It is to be noted that the degradation is sensitive to the peak-to-background ratio (P/B). The degradation due to the presence of background tends towards zero when the peak-to-background ratio tends towards infinity. The degradation, to some extent, is less sensitive to the ratio of the peak and background window widths. A typical compromise is to select ηB = ηP so that the multiplier in parenthesis in front of B/P becomes 2. The background degradation is lowered to some extend by a wider background integration width (ηB).
Figure 6 shows the plot of Equation 30 for a Gaussian peak of height l and FWHM, Γ. b is the average amplitude of the background. This graph is the same as Figure 2, but contains the precision degradation caused by the background. The parameter, (l/b)/[1 + ( ηP/ηB)], that specifies each of the four curves in Figure 6 relies strongly on l/b and mildly on ηP/ηB.
.jpg)
Figure 6. The dependence of the relative standard deviation in the net peak counts, σP/P, on the ratio of the peak amplitude, l, to the background amplitude, b, and on the width of the integration regions. <P> is the estimate of the net peak counts obtained by subtracting the background estimate from the peak plus background counts.
A specific case of ηP/Γ = 3 and ηP = ηB is considered for clarification. This case makes it obvious that the ratio of the peak amplitude to the background height, l/b varies in proportion to the peak-to-background ratio (P/B). As a result, there is a relationship between the parameter defining the four curves and the peak-to-background ratio (P/B). P/B = ∞ and l/b = ∞ when there is no background under the peak. Therefore, the bottom curve shown in Figure 6 is the case of zero background, and is identical to the function plotted for zero background in Figure 2.
Moving vertically from the bottom curve (∞) to the next curve (10) incorporates a background, and establishes a finite peak-to-background ratio. Stepping to the next curve (1) is equivalent to decreasing the peak-to-background ratio by a factor of 10. The top curve (0.1) decreases the peak-to-background ratio by another factor of 10. It is to be noted how statistical precision in the net peak counts is degraded with increasing background. The relative standard deviation in the net peak counts (σP/P) increases with decreasing peak-to-background ratio.
Figure 6 shows another quirk due to the presence of the background. The minimum relative standard deviation in the net peak counts for an infinite peak-to-background ratio (i.e., zero background) is derived by using a peak integration window width that is more than double the FWHM of the peak. However, at low peak-to-background ratios (0.1), the minimum relative standard deviation is attained at ηP = 1.17 Γ, which signifies that the peak integration window width should be set at 1.17 times the FWHM of the peak in order to achieve the lowest detection limits. Such a narrow window makes the results extremely sensitive to a misalignment of the center of the peak integration window with respect to the centroid of the peak. The shape of the peak is obscured by counting statistics near detection limits, making it difficult to center the integration window on the peak. Hence, it is recommended to set the peak integration window width two to three times more than the FWHM of the peak so that the consequences of misalignment can be minimized while degrading the relative standard deviation by only 26%. (See A1/2σP/P at ηP = 3 Γ and ηP = 1.17 Γ on the 0.1 curve in Figure 6.). This is a very small price to pay for protecting the results from the effects of misalignment of the peak and window.
How Background Controls Detection Limits
Statistical confidence limits are required to determine detection limits in the presence of background. The Gaussian approximation to the Poisson distribution can be used to compute the confidence limits. Figure 7 shows a Gaussian probability distribution and describes the 5% and 95% confidence limits. The horizontal axis in Figure 7 uses a simplifying substitution in the exponent of Equation 18.
.jpg)
Figure 7. The Gaussian probability distribution and the definition of the 5% and 95% confidence limits.
.jpg) |
(31) |
This substitution shifts the mean to zero, and presents the deviation from the mean in units of the standard deviation, σ. This normalized Gaussian probability distribution has the form found in most statistical tables2:
.jpg) |
(32) |
The 5% confidence limit takes place at z = –1.6449. Since the area of the Gaussian to the left of this coordinate value is 5% of the total area, the probability of observing events to the left of z = –1.6449 is only 5%. Likewise, the 95% confidence limit takes place at z = +1.6449, because 95% of the area of the Gaussian distribution lies to the left of this value of z. In other words, the probability of observing events with values of z to the left of z = +1.6449 is 95%.
Expressing these limits in terms of N via Equation 31, the 5% and 95% confidence limits can be expressed as follows:
N95% = µ + 1.6449 σ |
(33b) |
The 5% and 95% confidence limits must be carefully applied from a Gaussian distribution to the Poisson probability distribution at low values of µ, as the Poisson distribution is defined only at integer values of N, whereas the Gaussian distribution is continuous in N. Therefore, the limits derived from Equation 33 must be rounded to the nearest integer value of N when applied to the Poisson distribution. The value of µ must be ≥37 in order to keep the rounding error below 5% of 1.6449 σ. Generally, this is not a rigorous limitation.
To calculate the detection limit, the procedure shown in Figure 5 using Equation 27 will be used to measure the net peak counts. The net peak counts must be higher than a threshold value set at ND in order to claim detection. The traditional approach is to set the threshold value high enough that there is only a 5% probability of the background counts exceeding the threshold if the peak is truly absent. This establishes the 5% false positive condition. Equation 29 is used to determine the standard deviation in the net peak counts when the net peak counts are zero.
.jpg) |
(34) |
Hence, the threshold for a 5% false positive (95% true negative) is derived from Equation (33b).
.jpg) |
(35) |
When the peak is truly present at the detection limit (PDL), the traditional definition of the detection limit requires a 5% probability of a false negative, and 95% probability of claiming a true positive. In this case, Equations 33a and 29 can be used to determine the detection threshold (ND) as follows:
.jpg) |
(36) |
Setting the detection thresholds equal between Equations 35 and 36, and using the fact that PDL « B to avoid PDL from the square root sign in Equation 36 leads to the detection limit:
.jpg) |
(37) |
A more meaningful relationship can be established when the detection limit is expressed in terms of activity or concentration, where calibration equation relating concentration to net peak counts is
Where, C represents the activity or concentration, and K represents the calibration constant. CDL represents the detection limit expressed in units of concentration. Substituting Equation 38 in Equation 37 yields Equation 39.
.jpg) |
(39) |
The detection limit can be estimated using Equation 39 in the following way. A sample with a concentration C for the analyte is about a factor of 10 above the expected detection limit is quantified. The net peak counts (P), the background counts (B), and the counting time (t) are noted and entered into Equation 39 to estimate the predicted detection limit (CDL).
The most important point to be noted is that Equation 39 helps determine the factors controlling detection limits. For a sample with a specific concentration (C) and a selected value for ηP/ηB, the numerator of Equation 39 is a constant. The denominator shows that the detection limit relies on:
a) The peak-to-background ratio (P/B)
b) The net counting rate in the peak (P/t)
c) The counting time (t)
If any one of these parameters is increased by a factor of 4, there is a corresponding decrease in the detection limit by a factor of 2.
The counting time (t) is the most easily controlled parameter that influences the detection limit. However, most applications have a limit to the counting time. If an improvement of a factor of 10 is required in detection limits, and the counting time is already 1 day, then 100 days would be the counting time. It is not a viable option to have such a long counting time.
In certain cases, it is possible to increase the peak-to-background ratio by improving the spectrometer or by pre-concentration of the analyte in the sample. It is also possible to use similar techniques to increase the net counting rate in the peak.
Conclusion
It is evident that to achieve the lowest detection limits, parameters such as the peak-to-background ratio, the counting time, and the net counting rate in the peak need to be maximized. This same strategy is also the key for obtaining the best relative standard deviation for concentrations exceeding the detection limit.
References
1. Ron Jenkins, R.W. Gould, and Dale Gedcke, Quantitative X-Ray Spectrometry, Marcel Dekker, New York, First Edition (1981), pp 209 – 229.
2. Phillip R. Bevington, and D. Keith Robinson, Data Reduction and Error Analysis for the Physical Sciences, WCB McGraw-Hill, Boston, Second Edition, (1992).
3. Ibid. ref. 1, pp 229 – 244 and 252 – 276.
4. 1997/1998 EG&G ORTEC Catalog, pp 2.176 – 2.178
5. ORTEC Modular Pulse Processing Electronics Catalog (2001), Oak Ridge, USA, pp 8.3 – 8.4
6. Ibid. ref. 4, pp 2.282 – 2.283.
7. Ibid. ref. 5, pp 10.6 – 10.7.
8. D.A. Gedcke, ORTEC Application Note AN57, Dealing with Dead Time Distortion in a Time Digitizer, (2001).
9. D. A.Gedcke, ORTEC Application Note AN58, How Histogramming and Counting Statistics Affect Peak Position Precision, (2001).
10. Ibid. ref. 1, pp 337 – 360.
11. Robert L. Coldwell and Gary J. Bamford, The Theory and Operation of Spectral Analysis Using ROBFIT, American Institute of Physics, New York, 1991.
12. Ronald M. Keyser, Nucl. Instr. and Meth. A286 (1990) pp 403 – 414.
13. John D. Valentine and Asad E. Rana, Centroid and Full-Width at Half Maximum Uncertainties of Histogrammed Data with an Underlying Gaussian Distribution — The Moments Method, IEEE Trans. Nucl. Sci. Vol. 43, No. 5, Oct. 1996, pp 2501 – 2508.
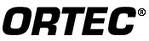
This information has been sourced, reviewed and adapted from materials provided by ORTEC.
For more information on this source, please visit ORTEC.