Updated by Ibtisam Abbasi 22/09/23
The main reasons for the boom of Artificial intelligence (AI) include its capability to enhance automation and boost productivity. The availability of extensive training data, enhanced computational capabilities, and advanced algorithms has facilitated the extensive implementation of AI, particularly in the domain of materials science and engineering, as the conventional trial-and-error approach has proven to be inefficient and time-consuming when studying materials. The article highlights several novel applications of various AI tools in materials research.
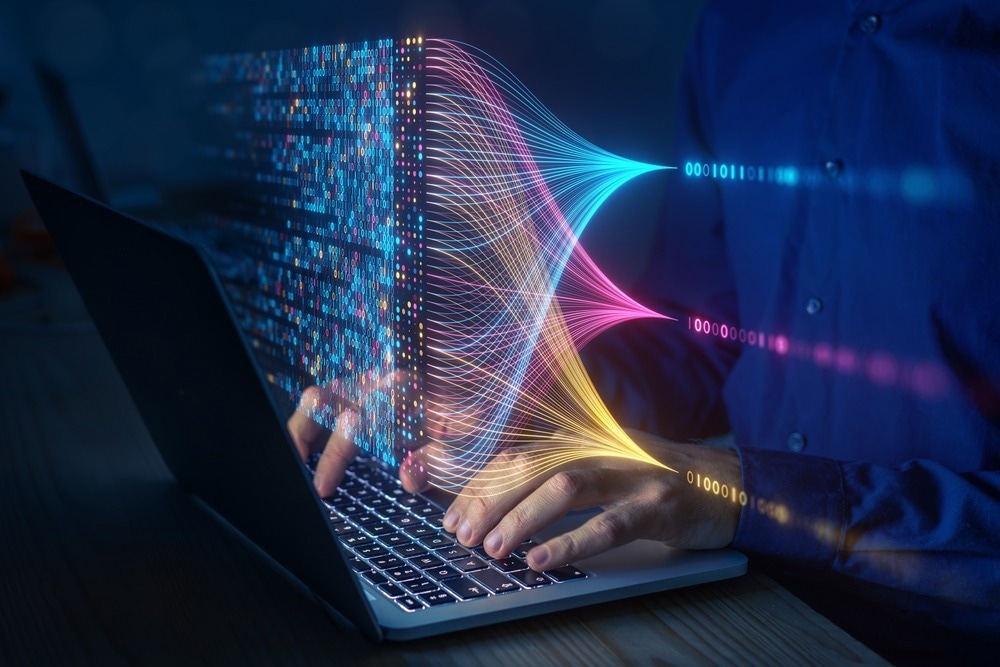
Image Credit: NicoElNino/Shutterstock.com
AI-based Language Models for Materials Science
With the widespread integration of machine learning (ML) into diverse scientific domains, AI has emerged as a vital form of language in the realm of physics. It holds the capability to efficiently aid in comprehending the complex underlying principles, thereby enhancing and optimizing the existing mathematical frameworks.
In the latest article published in APL Machine Learning, researchers have mentioned that AI has gained significant attention, particularly due to its capacity for predicting material properties and aiding in the comprehension of previously unexplained mechanisms. The integration of AI language models that exhibit massive potential in facilitating efficient predictions has emerged as a new frontier within the realm of materials research.
Despite the presence of various materials databases like MATDAT and MatWeb, researchers often find themselves compelled to extract relevant data from an array of studies. It has been proven that these language models, along with the efficient summarization of the materials science knowledge included within published literature, can also seamlessly convert the unorganized raw text into meticulously structured tabular data.
Structure and property predictions are the most essential tasks in materials research, and language models are currently introducing a novel chapter to this domain. Models like RNN, LSTM, and transformers have demonstrated their prowess in tackling intricate challenges related to protein folding, material property prediction, and the analysis of failures within complex nonlinear architected materials.
Furthermore, the integration of these models with generative neural networks, like Generative Adversarial Networks (GANs), could empower researchers to systematically navigate the complex phases of material design possibilities, a domain that remains beyond the reach of conventional methodologies.
How are Deep Learning Methods Revolutionizing Materials Science?
Deep learning (DL) is among the most rapidly expanding subjects within materials data science, showcasing a swift increase in applications that encompass atomistic, image-based, spectral, and textual data modes. DL facilitates the scrutiny of unstructured data and the automated recognition of distinctive attributes.
Computational Materials highlighted the increasing use of DL-based approaches for materials science technology through its latest article. Historically, materials have been developed through experimental processes, relying on trial-and-error methodologies. DL techniques present a considerable acceleration when contrasted with traditional scientific computing approaches. Additionally, in specific applications, these methods are approaching levels of accuracy comparable to those achieved by physics-based or computational models.
DL methodologies can be utilized to establish a robust relationship between atomic structures yielding precise outcomes. Approaches such as SchNet and the crystal graph convolutional neural network (CGCNN) have recently been employed to forecast an extensive number of essential properties across both crystalline and molecular materials.
Materials' microstructure is frequently depicted as complex multiphase high-dimensional 2D/3D images. This characteristic renders image-based DL methodologies particularly advantageous, allowing flawless representation of resilient, and reduced-dimensional microstructure. These representations can subsequently be used to formulate predictive and generative models. In short, DL methods are revolutionizing materials science methodologies.
Implementation of Graph Neural Networks
Graph neural networks (GNNs) are constantly on the up-rise concerning their applications. As per the article published in Communications Materials, their significance is particularly relevant in the fields of chemistry and materials science due to their direct applicability to graphs or structural depictions of molecules and materials. Consequently, GNNs possess comprehensive access to all substantial information indispensable for the thorough characterization of materials.
In materials science, a complex task is the pursuit of inverse materials design aimed at developing new materials or molecules that exhibit desired properties while satisfying distinct criteria. To address this challenge, GNN-driven generative techniques have been proposed. These methodologies have proven particularly effective for tasks like drug discovery. In many instances, the output solely depends on the chemical structure of molecules without incorporating additional details regarding the specific three-dimensional geometry.
GNNs can obtain internal representations of materials that prove valuable and enlightening for particular objectives, such as forecasting specific properties of provided materials. In the context of GNNs, the complete molecular structure, including geometry, serves as input. The GNN then automatically learns insightful molecular representations to guess the designated target properties. A substantial proportion of GNNs tailored for chemistry and materials science can be categorized within the Message Passing Graph Neural Networks (MPNN) framework.
Supervised and Unsupervised Learning Examples in Materials Science
Supervised Learning
Example methods
- Regularized least-squares
- Support vector machines
- Kernel ridge regression
- Neural networks
- Decision trees
- Genetic programming
Selected material applications
- Prediction of processing structure-property relationships
- Development of model Hamiltonians
- Prediction of crystal structures
- Classify crystal structures
Unsupervised Learning
Example methods
- k‐Means clustering
- Mean shift theory
- Markov random fields
- Hierarchical cluster analysis
- Principal component analysis
- Cross‐correlation
Selected materials applications
- Analysis of composition spreads from combinatorial experiments
- Analysis of micrographs
The Different Steps of an ML Workflow
Data Processing
Data processing is an essential step of machine learning that directly affects the performance of the machine learning model used for predictions in materials science. Data processing usually consists of two parts: data selection and feature engineering.
- Data Selection: In this step, data is selected comprehensively with respect to its type, quality, and format.
- Feature Engineering: Feature engineering helps to extract suitable features from raw data obtained from experimentation or simulations to enable the application of ML algorithms in materials science.
Modeling
Modeling comes after data processing. It includes selecting appropriate algorithms, training, and making accurate predictions. There are several algorithms available to implement the four different types of ML methods discussed earlier. They can be divided into two types:
- Shallow Learning: Shallow learning methods include support vector machine (SVM), decision tree (DT), and artificial neural network (ANN). They are mainly used in linear classification [4].
- Deep Learning: Deep learning (DL) uses a non-linear cascade processing unit for automatic feature extraction. It derives low‐level features to obtain more abstract high‐level representations of attribute categories. DL models typically outperform SL models for non-linear tasks. Examples of DL models used in materials science: convolutional neural network (CNN), recurrent neural network (RNN), deep belief network (DBN), and deep coding network.
Model Validation
There is always a need to validate the stability of an ML model. Model validation is conducted post-training of the model to evaluate the accuracy of the model by using new unseen data, which differs from the data in the training data set. ML methods usually divide the original data into a training set and a test set, and use the training set for model training and the test set for model validation.
Discover the materials of the future...in 30 seconds or less | Dr. Taylor Sparks | TEDxSaltLakeCity
Video Credit: TEDx Talks/YouTube.com
What Does the Future Hold?
Currently, the dataset for the majority of materials is limited and often termed a “small” data volume. This status is anticipated to persist for an extended duration. The latest article published in Computational Materials states that algorithms based on this small data should be optimized rather than developing new frameworks focusing on the interpretation of big data.
Historically, the dataset has remained static, with machine learning models primarily focused on specific datasets. However, the current trends in this field emphasize data iteration, with a heightened emphasis on refining model efficacy through iterative techniques like active learning. This requires a more methodical approach to data management ensuring efficiency and accuracy.
Present applications of AI within materials science predominantly revolve around material screening or the utilization of patterns revealed by models to devise materials, a practice often referred to as forward design. In the coming years, there will be an increased demand for inverse design, a process necessitating the forecasting of material composition and structure from limited datasets in alignment with desired properties.
AI is continuously providing valuable insights, particularly for materials research. AI is not a replacement for human researchers; instead, it is set to function as an effective tool, accelerating the discoveries and unveiling the complex concepts related to materials science.
Find out more about how artificial intelligence has been applied to other areas of science.
References and Further Reading
Hu, Y. et. al. (2023). Deep language models for interpretative and predictive materials science. APL Machine Learning, 1(1). Available at: https://doi.org/10.1063/5.0134317
Choudhary, K. et al. (2022). Recent advances and applications of deep learning methods in materials science. npj Comput Mater. 8, 59. Available at: https://doi.org/10.1038/s41524-022-00734-6
Reiser, P. et al. (2022). Graph neural networks for materials science and chemistry. Commun Mater 3, 93. Available at: https://doi.org/10.1038/s43246-022-00315-6
Xu, P. et al. (2023). Small data machine learning in materials science. npj Comput Mater 9, 42. Available at: https://doi.org/10.1038/s41524-023-01000-z
Yue Liu, et al. (2017) Materials discovery and design using machine learning. Journal of Materiomics. https://doi.org/10.1016/j.jmat.2017.08.002
Abdul Hamid Halabi. (2019) NVIDIA CEO Ties AI-Driven Medical Advances to Data-Driven Leaps in Every Industry. [Online] NVIDIA. Available at: https://blogs.nvidia.com/blog/2019/04/09/world-medical-innovation-forum-2019/ (Accessed on 26 March 2020).
Tim Mueller, et al. (2016) Machine Learning in Materials Science: Recent Progress and Emerging Applications. Reviews in Computational Chemistry. https://tsapps.nist.gov/publication/get_pdf.cfm?pub_id=915933
Jing Wei, et al. (2019) Machine learning in materials science. InfoMat. https://doi.org/10.1002/inf2.12028
Jonathan Schmidt, et al. (2019) Recent advances and applications of machine learning in solid-state materials science. npj Computational Materials. https://doi.org/10.1038/s41524-019-0221-0
Disclaimer: The views expressed here are those of the author expressed in their private capacity and do not necessarily represent the views of AZoM.com Limited T/A AZoNetwork the owner and operator of this website. This disclaimer forms part of the Terms and conditions of use of this website.