When purchasing raw materials, it is very important to confirm that the right materials have been received for economic, quality and regulatory reasons. Often these raw materials, examples of which are food or pharmaceutical ingredients, are transported in packaging that are either plastic or paper that can be opened so that verification test using analytical techniques such as assays or infrared spectroscopy can be performed.
Opening material packaging, however, has some disadvantages:
- Reduced Cleanliness and Sterility – After a raw material packaging is opened, a sample has to be extracted or a sampling probe must be done with the material. This sets up the possibility of contaminating the sample with chemical or biological pollutants. Moreover, if the same probe is used on several products, the risk of cross-contaminating the raw materials increase.
- Increased Spoilage – Some raw materials are vacuum packed. They are sheltered from oxygen, humidity, or ultraviolet light. When the package is opened, the exposure to these elements that may decrease shelf life or degrade the material in a manner that results in failure of downstream processes.
- Threat to Personnel Safety – At some occasions, incoming materials may present a danger to receiving or laboratory personnel who opens the packages and performs the analysis. Other personal protection measures like gloves and respirators may be required, increasing costs and risks.
Some technologies that exist today allow sampling of raw materials through their packaging. To cite an example, specialized Raman spectroscopy techniques can at times be sampled through semi-transparent packaging. However, Raman spectroscopy suffers from fluorescence interference, inclined to have sampling areas that are small, can burn the sample or packaging, and is sometimes hard to use as a quantitative technique, making it difficult to be utilized for many applications.
Near-infrared spectroscopy holds promise for packaging and containers sampling because it can go through several millimeters into a material, making the characteristics of both the packaging material and the sample contained within seen.
.png)
Figure 1 presents an example of this problem. With a variety of the common sugar dextrose (blue), a spectrum of a standard 6-mil polyethylene bag (green), and a spectrum showing dextrose inside the polyethylene bag (magenta), taken through the bag. By comparing the spectra, it is clearly shown that the absorption of the dextrose features can be seen through the bag.
.jpg)
Figure 1. FT-NIR spectra of dextrose (blue), 6-mil polyethylene bag (green), and dextrose inside a 6-mil polyethylene bag (magenta)
For that reason, the main test in using near-infrared spectroscopy is getting rid of the contribution of the packaging from the total absorption spectrum. Basic removal techniques, like spectral subtraction, may be able to work under very controlled circumstances. However, they are not sophisticated enough to deal with changeable thickness of packaging, scattering properties, packaging additives, and other variations in packaging and underlying raw materials; for the reason that they are subjective and they require some level of operator expertise.
This problem can be made problematic by the fact that some near-infrared spectrometers do not have the performance required to effectively resolve the absorbance peaks of the packaging and underlying material, making it hard for an operator to judge how much to subtract. For example, the C-H overtone band in dextrose at 5920 cm-1 is only about 10 cm-1 (5 nm) wide, which is outside the resolution of many near-infrared instruments.
Nevertheless, when modern Fourier Transform near-infrared (FT-NIR) spectrometers, like Galaxy Scientific’s QuasIR™ series, are joined with novel chemo metric techniques, such as Galaxy Scientific’s Advanced-ID™ algorithm, classification of materials through packaging becomes attainable. To illustrate the real-world effectiveness of this technique, an experiment was set up to evaluate some raw materials inside various forms of packaging.
Experimental Method
Materials
Three basic samples were acquired from local sources to represent incoming raw materials: lactose- a sugar present in milk; dextrose- the naturally occurring form of glucose; and aspartame- an artificial sweetener. These samples were refined, white powders that are almost identical to the naked eye.
.png)
Figure 2. Containers used for the experiment.
To represent common kinds of packaging, six different containers were used:
- Two (2) different clear 6-mil polyethylene bags
- One (1) green 6-mil static dissipative polyethylene bag,
- One (1) white opaque 4-mil polyethylene bag
- One (1) white paper security envelope with blue dye
- One (1) manila envelope
Sample Measurement
FT-NIR spectra were gathered together using a QuasIR™ 2000 spectrometer (Galaxy Scientific, Nashua, NH, USA) set with a diffuse reflectance fiber optic probe. The diffuse reflectance probe was held upward using a versatile probe stand and the samples were positioned on top of the probe tip for it to be measured. Each sample was measured at 4 cm-1 resolution and 32 scans were averaged. Three measurements were taken in each of the raw material, the package without raw material, and the package with the raw material. Samples were moved on the probe between every measurement.
.jpg)
Figure 3. Diffuse reflectance probe (in stand) with sample in polyethylene bag
Data Processing
For data collection, Spectral Sage™ software was used and for the analysis, Advanced-ID™ algorithm and software were used. The pure packaging spectra were all included in the study. The spectral range was limited to 4100-6800 cm-1 because that was the region where most of the spectral differences were present.
To compare, a standard correlation was also carried out. The correlation was calculated between the raw material with packaging and the pure raw material spectrum. The spectral range of the standard correlation was also set to 4100-6800 cm-1. The pure raw material spectra used for both of these analyses are shown in Figure 4 and the spectra of the pure packaging material are presented in Figure 5.
.jpg)
Figure 4. Three spectra of each raw material: lactose monohydrate (green), dextrose (blue), and aspartame (red)
.jpg)
Figure 5. Spectra of pure packaging material
Results and Discussion
It can be challenging to collect near-infrared spectra of materials through packaging due to the fact that the packaging can often contribute a lot to the resulting absorption spectrum. There are two major reasons for this. First, a lot of materials used for packaging are made from plastic or paper which have significant absorption bands in the near-infrared. For example, polyethylene has strong absorption bands in the C-H stretching overtone region around 5500-6250 cm-1 (1600-1800 nm).
Second, the distribution properties of the packaging and the material behind it, and the fact that the light must go through the packaging twice to return to the optical probe, means the helpful path through the packaging may be huge in comparison to the path in the material behind it.
Figure 6 demonstrates this difficulty. The green spectrum shows the outcome of sampling aspartame though a manila envelope. The spectrum looks nearly matching to the spectrum shown in magenta, which is the spectrum of a pure manila envelope. It is almost not possible to differentiate by eye the aspartame present in the spectrum which has a blue color. When an easy correlation algorithm or other common spectral matching technique is utilized to compare the sample spectrum to the pure material spectrum, the outcomes are evidently poor.
.jpg)
Figure 6. Spectrum of pure aspartame (blue) compared to the spectrum of a manila envelope (magenta) and aspartame sampled through a manila envelope (blue). It is nearly impossible to distinguish the aspartame through the packaging by eye.
To deal with this problem, Galaxy Scientific’s Advanced-ID algorithm first makes a least squares regression that consists of both the packaging and the potential raw materials so that the sample spectrum can be approximated. After that, an extracted spectrum is formed by calculating a residual with the regression coefficient for the raw material spectrum set to zero. Next, the extracted spectrum is used to match against the inventory of potential raw materials.
Continuing the prior example of sampling aspartame through a manila envelope, Figure 7 shows the extracted spectrum (blue) produced by the Advanced-ID algorithm. The extracted spectrum can be easily measured up to a pure spectrum of the material in query. In Figure 7, the extracted spectrum (blue) is compared to two possible raw materials: aspartame (red) and dextrose (magenta). Even by using the eye, it can be seen that the extracted spectrum is a good match to aspartame.
.jpg)
Figure 7. An example extracted spectrum from aspartame through a manila envelope (blue) produced by Advanced-ID can be compared to pure aspartame (red) and dextrose (magenta).
To compare the efficiency of the Advanced-ID algorithm to a conventional method, each of the collected samples were processed and compared to the simple correlation (also known as HQI or Hit Quality Index) result. Table 1 presents the top match result using simple correlation, the coefficient of correlation to the top match, the top match of the Advanced-ID method, and the coefficient of correlation of the extracted spectrum to the top match. The cells are shaded red if the match was wrong, yellow if the match was right but had a correlation value below 0.9, and green if the match was right and had a high correlation coefficient. To be concise, only one sample of the three taken is shown in the table. The other two samples had very close correlation outcomes.
As shown in Table 1, the traditional correlation method makes a correct match on only 10 out of 18 samples. In addition, four of those samples have a correlation coefficient below 0.9, which usually indicates of a poor match.
Table 1. Comparison of results of simple correlation to the Advanced-ID method where the extracted spectrum, (after packaging removal), is correlated to the pure material spectra.
.jpg)
The Advanced-ID technique produces the accurate match 18 out of 18 times and every match has a correlation coefficient above 0.98. This indicates that the match to the pure spectrum is very good. It is obvious from Table 1 that Advanced-ID can effectively take out the target spectrum from the packaging material and supply a correct match with high confidence in the result.
In contrast, the simple correlation algorithm does not do well in the presence of packaging and regularly generates “lactose” as the top match due to the resemblance between the packaging and lactose spectra. If the spectrum of the packaging material had been included in the list of possible raw material spectra that the correlation algorithm compares to, the packaging material would have been the top match.
As the packaging spectrum is subtracted from the original sample spectrum, the results of the simple correlation method might slightly improve, but it would need advanced information about which packaging material was present. Even then, it would still be much less effective than Advanced-ID due to differences in the packaging thickness and other inconsistencies. As already noted, manual subtraction is also subjective and depends mainly on skill of the operator.
In order to achieve the least-squares regression and produce the extracted spectrum, it should be noted that Advanced-ID necessitates spectra of the packaging to be obtainable. Still, the packaging does not need to be exactly the same as that used with the sample. To show this, the packaging material used in the “PE Bag 2” samples was not included in the library of potential packaging materials. Since the other packaging materials were made of polyethylene, they were able put it in the sample spectrum and remove it successfully despite the differences in the material composition or thickness.
The correlation coefficients from this set of data were only a bit lower than correlation coefficients from the “Clear PE bag” data set where the packaging spectra were included in the packaging library. The investigation still did well because the least-squares fitting allows for variation in the packaging spectra that makes up for variations in packaging thickness or subtle differences in material. This explains that only a relatively small library of packaging spectra are needed to make the algorithm work well.
One more vital point is that all of the packaging materials utilized in this experiment were included in the packaging library for every analysis. There was no need for a prior knowledge of where the sample was contained in. The algorithm fits every one of the possible packaging spectra and offers higher regression coefficients (e.g. mathematical weights) to the ones that closely match the sample spectrum. This makes the method very easy to use, even when the packaging differs between different raw materials or raw material suppliers.
Lastly, it’s significant to understand that the matching algorithm used on the extracted spectrum was a simple correlation. Just as more sophisticated matching methods, the use of derivatives for example, can improve the simple correlation results, the same techniques can be applied to the extracted spectrum produced from Advanced-ID.
To cite an example, results could be improved by pre-processing the extracted spectrum, feeding extracted spectrum into SIMCA or cluster analysis, or using another statistical method that weights the spectral regions based on noise bands or other factors.
Conclusion
Raw materials can be known through common types of packaging like polyethylene and paper by the use of Galaxy Scientific’s Advanced-ID algorithm and the QuasIR series FT-NIR. This method significantly improves the capacity to validate incoming raw materials using near-infrared spectroscopy.
.jpg)
Figure 8. Galaxy Scientific’s QuasIR™ 2000 with ergonomic trigger prob
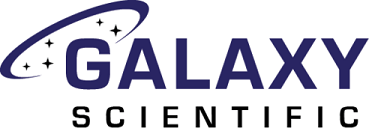
This information has been sourced, reviewed and adapted from materials provided by Galaxy Scientific Inc.
For more information on this source, please visit Galaxy Scientific Inc.