For the sake of obviousness, a number of the examples in the Software section utilized artificially-prepared mixes of spheres and another type of particle. Now a real-world sample will be looked at, which is made up of two classes of particles.
There are some small round particles plus larger, more irregular objects visible in the typical raw image below. (In this article all thumbnail and particle images are reduced in size).
.png)
Image Credit: Vision Analytical Inc.
.png)
Image Credit: Vision Analytical Inc.
Frequency distributions can be observed for four measures for this sample using the image above. The ECA diameter graph shows a bimodal distribution, probably representing the two types of particles seen in the raw image.
The task to carry out is to extract separate shape and size statistics on the two sub-classes of particles. There are three ways of accomplishing this, which are described in this article. Before beginning, note that the ellipse length and width graphs are nearly identical, which confirms that almost all of the particles possess aspect ratio (length/width) near to 1. However, that does not necessarily confirm that they are spherical.
.png)
Image Credit: Vision Analytical Inc.
Single Measure Analysis
The “large data window” for the ECA diameter is shown below. Statistics for the complete size range are also shown. The particle count is around 50,000, which is big enough to generate smooth distributions in the measure graphs. The likely overlapped parts of the two particle classes are indicated by the dotted red lines.
.png)
Image Credit: Vision Analytical Inc.
To get a good picture of the two classes of particles we can split the ECA distribution into two parts. The two parts will both contain a small amount of data from the other size class because of the overlap. In order to isolate the first peak we close down the size range to that part of it which is less than 5 microns, as shown below.
.png)
Image Credit: Vision Analytical Inc.
The smaller particles represent about 63% of the total and look to number about 31,000. The median is 2.2 microns and the mean size is 2.5 microns. The distribution is skewed slightly to the right (skewness > 0).
.png)
Image Credit: Vision Analytical Inc.
The size range has been restricted to that part which is above 5 microns, which is shown in the above graph. These are the larger objects which can be seen in the raw image. Particle count is around 18,000, which represents 37% of the cumulative total by number. The Mean is 9.8 microns. Skewness is shown as slightly positive, this is because the lower-end tail is cut off, but the distribution is probably pretty close to normal (unskewed).
Using this page of particle thumbnails for objects greater than 5 microns, it can be observed that they are an irregular shape.
.png)
Image Credit: Vision Analytical Inc.
Multi-measure Analysis
By taking into account other measures besides ECAD it may be possible to separate the two particle classes more accurately. Using the original Smoothness graph it looks like the two classes could possess different smoothnesses. In order to confirm that, the correlation between Smoothness and ECAD can be examined:
.png)
Image Credit: Vision Analytical Inc.
In this scatter chart the two subpopulations are clearly visible, although there is an amount of overlap present even here. It is clearly shown on this chart that the larger class of particles are smoother on their surface than the smaller group. The red line depicts the division of the sample into two parts which was carried out in the single-measure analysis. Using that method you can see that many of the “group 1” particles (the lower size group) actually got counted in group 2.
To allow more accurate separation of the two parts, restrict ECAD to be > 5 and Smoothness to be > 0.5, then just the particles that are inside the red box below should be captured.
.png)
Use the Particle Insight post-process reanalysis tool to do that. Enter the restrictions in Limits:
.png)
To regenerate all statistics click ‘Recompute graphs and statistics.’ The ensuing ECAD graph is fairly close to the actual size distribution in the upper group, and it is more precise than simply using the upper part of the original ECAD distribution.
.png)
It is trickier to locate a rectangle which holds the lower size group, but ECAD < 8 microns and Smoothness < 0.6 will catch the majority of them.
.png)
.png)
With the majority of particles at the low end, this class of particles is observed to be extremely non-normal. This is common of very fine particles, both liquid and solid.
.png)
A small overlap area exists which the two rectangles have in common, resulting in a few particles being counted in both classes. However this method does a fairly good job of separating the classes, particularly the group 2 (larger) class.
Particle Classification
The Classification tool available in Post-Processing can carry out the same separation as described above, but in fewer steps. Define two classes as shown below:
.png)
Click OK, and for each class a brief statistics summary is listed:
.png)
Select Statistics, with Type equal to Fines, and Measure set to ECAD, the same regeneration as before using ‘Recompute graphs and statistics’ for ECAD will be produced. To obtain results for the Large Objects class, simply set Type to Large Objects and click Statistics again.
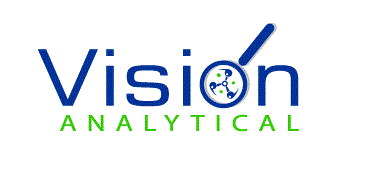
This information has been sourced, reviewed and adapted from materials provided by Vision Analytical Inc.
For more information on this source, please visit Vision Analytical Inc.