In this interview, Mathew Andrew, a Scientist from Carl Zeiss talks to AZoM about the concept of Xradia 3D X-ray microscopes (XRM) and how they are taking X-Ray Microscopy to the next level with their range of products.
Can you tell us about the concept of Xradia 3D X-ray microscopes (XRM)?
If you want to image things at a very high resolution, you have got a few different options available to you, depending on what you want to do and the way you want to do it. Most commonly people are familiar with traditional light microscopes, where typically samples are either polished as a block or sliced very thinly into a section.
These prepared samples are then imaged in only two dimensions. You have sliced it, and it is very thin. Essentially, you have more or less destroyed the sample prior to investigating it. There are some exceptions to this, such as doing 3D microscopy in vivo and non-invasively, or using 3D imaging systems such as confocal or light-sheet microscopy, but most often you are limited to 2D.
You can go to very high resolutions with an electron microscope, down to a nanometer, or even less in spatial resolution. Once again, however, this is only 2D. The challenge remains, therefore; how do we image things in 3D and (even more challenging) image them without cutting them open. If you think about everyday life, this challenge also presents itself to you. When you go into a hospital, one of the diagnostic techniques commonly used is called a CAT or a computed tomography (CT) scan. This is used because the other option is exploratory surgery, extracting a piece from a certain area, and putting it on a slice, and then putting it in the light microscope. This is what is called a biopsy, and is super useful, but is invasive and carries subsequent risks. Mostly invasive surgery is not a viable option, so X-rays can be used. Famously, X-rays can see through tissues, and CT can be used to image in 3D. If we want to do this at very high resolution, this is called “microCT”; rather than the millimeter scale (modern medical CTs can do a bit better than this) we go down to the micron scale (or even lower).
To achieve this, instead of having the X-rays source and detector rotate around you as would happen in clinical CT, you have the source and detector stay still, and the sample goes into the instrument and rotates. X-ray images are acquired at equally spaced angular increments, and from this, we reconstruct a three-dimensional volume.
The fundamental advantage of X-ray imaging is that it is non-invasive – it allows you to image structures deep within objects. The major challenges in materials characterization and analysis lie in the ability to image complex processes as they occur (so-called in situ experiments) and the ability to image complex structures across multiple scales. The unique thing about the ZEISS Xradia systems is that rather than being a microCT, they are X-ray microscopes. The big difference between X-ray microscopy and microCT is the way that x-rays are detected. The way that magnification is achieved in microCT is through projection-based magnification – a sample is placed very close to the X-ray source, and the X-rays diverge much as light does with a film projector, onto a large X-ray detector. This is very useful but has some big downsides, the principal of which is that you need to be able to move the sample close to the source. ZEISS Xradia systems have secondary magnification, making them unique. They are the only systems commercially available that have this secondary magnification on the detector. We convert X-rays to visible light, and then we put that visible light through optical magnification before detecting them using a high-resolution CCD camera.
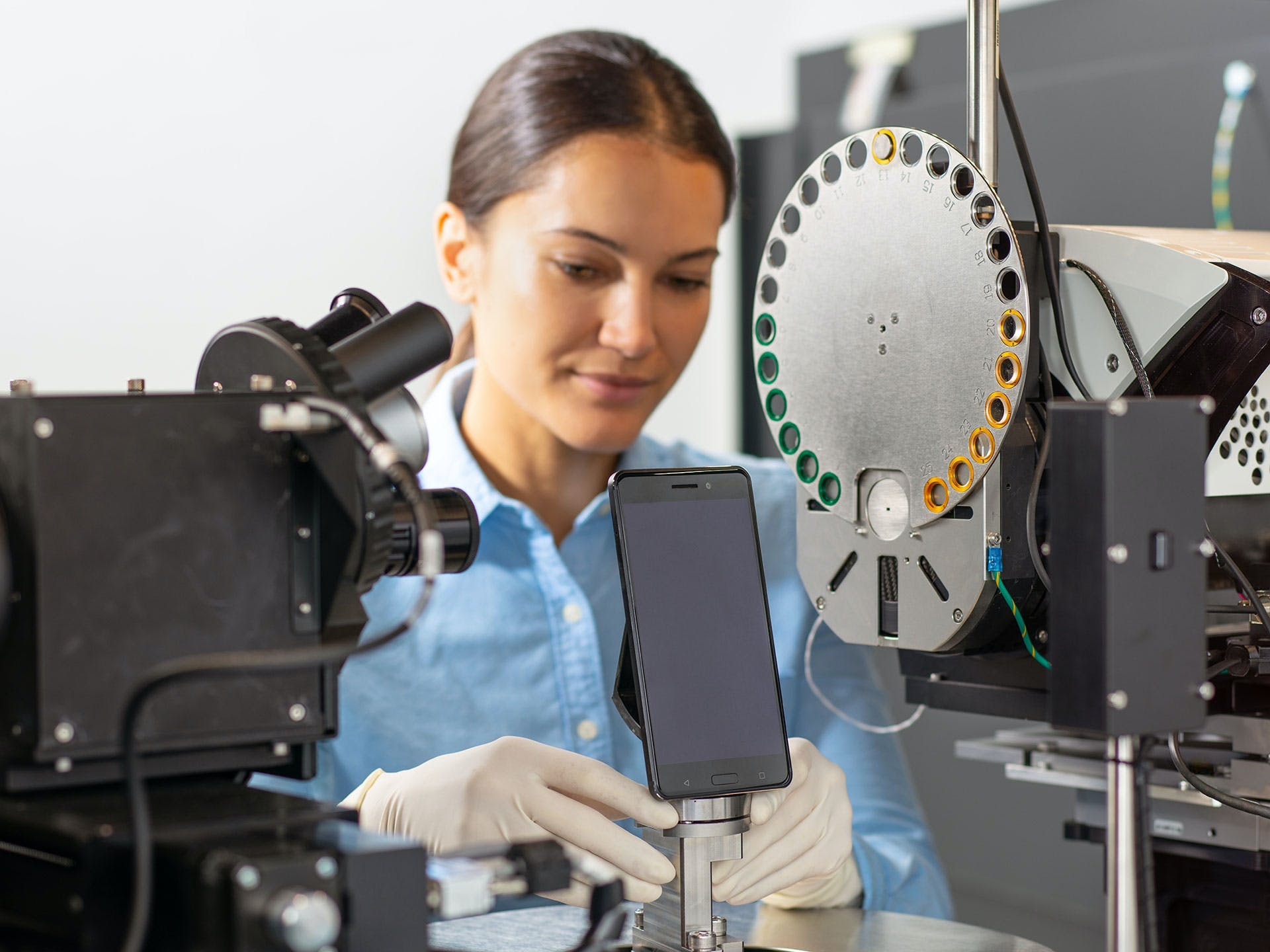
What that allows you to do is to image objects within large objects while maintaining very high resolution. That is important if you want to address those major scientific challenges such as multiscale and in situ characterization. In situ characterization refers to the imaging of processes as they occur in real-time within materials. For example, maybe I want to get a carbon fiber composite and stress it, compress it, pull it, heat it up, or cool it down. Perhaps I want to study fluid flow through porous materials or a battery that goes through charge and discharge cycles.
As these things get more complex, the equipment needed to do them gets more advanced. Similarly, when analyzing complex multiscale structures it is essential to be able to zoom in at specified regions within a large sample. In both cases, material outside the region of interest prevents you from placing the sample close to the source, limiting geometric magnification. You can maintain a high resolution, however, with the secondary magnification within an X-ray microscope.
The downside of this approach is that it can sometimes lead to long acquisition times, especially when you are doing these complex workflows, and noise levels can be quite high. Addressing these issues is what we are talking about today, the ways we can use advanced algorithms to reduce imaging artifacts and noise, speeding up acquisition, and improving image quality.
What does 3D reconstruction involve?
The three major components of any X-ray imaging device is an X-ray source, an X-ray detector, and a relative rotation between this pair and the sample. In our case, we have a rotational sample stage, and we rotate the sample through a series of equally spaced angular increments, moving 360 degrees, for example. At each increment, usually less than a degree per increment, we will acquire a new projection, expose the camera and take a new image. Each projection is a “compressed” 2D version of the 3D object being imaged, as X-ray flux goes through and dissipates along it, representing different material densities that the object is comprised of.
Each 2D projection is not very useful by itself, but the whole set of angular projections then goes into a reconstruction algorithm to give you a three-dimensional representation of the sample. It is a transformation of data that has been acquired in the angular domain into the XYZ three-dimensional domain. The required transformation is quite complex in nature, requiring millions of mathematical operations, impractical to be performed by human hands, and requires utilization of powerful computers, hence the name “Computed Tomography” or CT. The most commonly used algorithm for this is analytical filtered back projection-based methods, also known as Feldkamp-Davis-Kress or FDK reconstruction. Its main benefit is that it is computationally efficient, however, it does not perform well when image quality and fast acquisition is a priority. Today, with the introduction of the ZEISS Advanced Reconstruction Toolbox we are showcasing two other techniques for reconstruction – ZEISS OptiRecon (an implementation of model-based iterative reconstruction) and ZEISS DeepRecon (an implementation of deep learning Artificial Intelligence (AI) for reconstruction).
Can you give us an introduction to the ZEISS Advanced Reconstruction Toolbox (ART) platform?
The Advanced Reconstruction Toolbox is an umbrella term used to refer to a whole range of tools to allow for advanced data acquisition and accurate image reconstruction as compared to what can be achieved with traditional analytical reconstruction. Today we are presenting two major components, however, it is a continual area of innovation for us, so make sure you keep an eye out for more things coming out in the future!
These two components are called OptiRecon and DeepRecon. OptiRecon is an implementation of iterative reconstruction, which allows for an improvement in throughputs of up to four times while maintaining image quality, or an improvement of image quality at the same throughput. DeepRecon is the first commercially available implementation of deep learning in the reconstruction process, and this allows for extreme benefits in both throughput and image quality. This is an up to an order of magnitude improvement in throughput, or an increase of up to 10X.
When it comes to the first version of ART, what XRM performance attributes does it target, and can these technological innovations take application within X-ray microscopy to the next level?
Performance in an X-ray imaging device is a many-headed beast, with dimensions such as resolution, contrast, throughput, sample size, or field of view. For this release of the ART we are targeting image quality and throughput. At its simplest level, performance in an XRM can be viewed as a three-way trade-off between resolution, throughput, and image quality. If you want to improve image quality, you have to either trade-off resolution or throughput. The point of these technologies is that they allow you to improve the entire performance envelope, without making trade-offs. As image quality and throughput are often two sides of the same coin, you can use both reconstruction techniques (OptiRecon and DeepRecon) to either improve throughput while keeping image quality constant or improve image quality while keeping throughput constant.
It should be noted that these algorithms are complementary to improvements that can be made in the hardware as well. For example, in the last year we came out with the new 600 series Versa. This included multiple updates, allowing for up to a factor of 2X throughput improvement, but these incremental grains in hardware should be commensurate with improvements in the rest of the X-ray acquisition workflow, making software and algorithmic development equally important.
In ART’s first version, you are launching both the OptiRecon and the DeepRecon. What are they, and how do they differ from each other?
Most common in tomographic imaging, analytical reconstruction creates an image in a single pass: we weight and filter the projections, then back-project them into the volume domain using a technique called the inverse Radon transform, which is essentially just adding up line integrals from all the projections at different angles. This technique is fast and easy to implement but the mathematical theory behind it imposes high standards for acquired data which is hard to achieve in practice.
In contrast, OptiRecon is an implementation of iterative reconstruction, allowing for up to four times improvement in throughput. Iterative reconstruction builds up a reconstructed volume over a sequence of iterations. We take that volume (for initial guess it can be set to an arbitrary value), we forward project it, and then we compare the forward projection results (the model projection dataset), with the measured image projection dataset. Then, over a sequence of multiple iterations, we build up that three-dimensional volume to best fit between this real projection data set and the model projection data set.
The advantage of using this approach is that when I say compare, it covers a whole variety of sins. “Compare” actually means you use very complicated loss and regularization functions, which build in explicit knowledge about the way that our instrument works. The first ZEISS Xradia Versa XRM was released 9 years ago, and we were making X-ray imaging systems long before that. Through this time we have come to know a lot about the way that our instruments exhibit noise and artefacts, and we can explicitly build this knowledge into the reconstruction process. That allows us to eliminate most of the image degrading factors, allowing for faster acquisition times or higher quality images.
Even though humans know a lot about X-Ray imaging and apply that knowledge in practice, computers can do the same and even better, because they can review and learn from millions of CT images in a matter of hours. ZEISS DeepRecon is the first commercially available implementation of deep learning, or AI, for XRM image reconstruction. The way that it works is by training a series of convolutional neural networks that the data has to pass through as an integral part of the reconstruction process, before it is presented in the volume domain. These networks remove both image artifacts and noise as they are trained across a dataset, and the best fit network is one that recovers all the features while getting rid of all the noise. This ends up working well, and is why we get an extreme performance benefit (up to 10X faster acquisition times at comparable image quality).
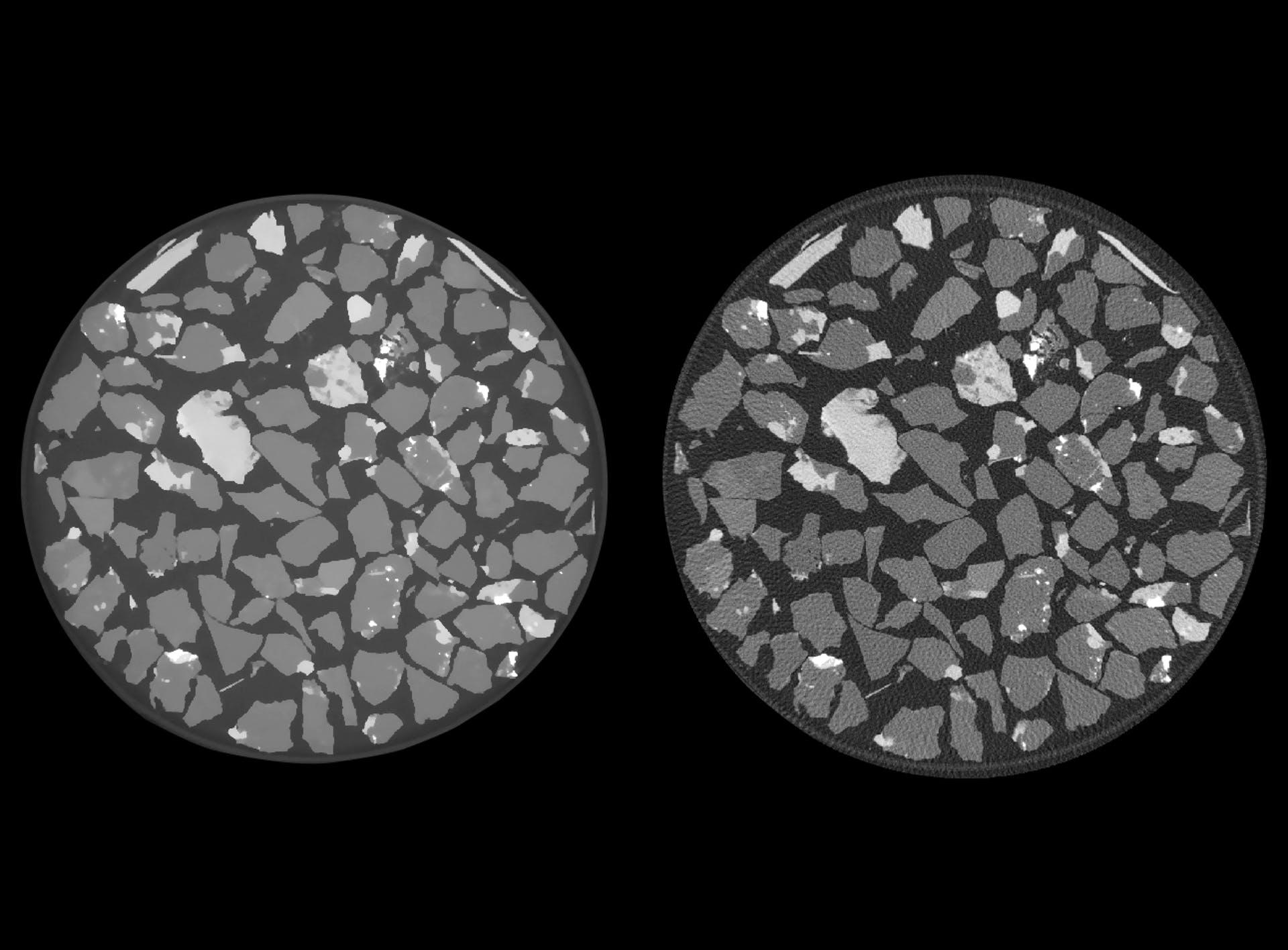
How does iterative reconstruction, such as OptiRecon, work, and how can it be utilized?
Iterative reconstruction works by building up a three-dimensional model over multiple iterations. It takes a volume, forward projecting it, building a model projection dataset, and then at each iteration, comparing that with the real projection data set using a loss function. This then allows us to build an explicit knowledge of the instrument's noise behavior, or of its artifact behavior.
Its big advantage relative to DeepRecon is that it does not require any pre-training of a model before implementation. It can take data without any pre-information about the sample and improve image quality.
DeepRecon models must be trained, so they are best targeted at repetitive workflows where the same sample is being imaged again and again. In this case networks must only be trained once but can be applied many times over many samples.
Why do you think DeepRecon is the first commercially available deep learning reconstruction technology for X-ray microscopy? Were there any obstacles that had to be overcome during its development?
One of the big challenges in building technology for deep learning is the generalizability of the resulting models. When you train a deep learning model, it is trained on a particular training set. We did a lot of work in the implementation and development of this technology to allow for that, and to enable as general a model as possible, within a particular sample class.
At ZEISS, we always try to be forward-looking, and deep learning and AI has been a focus for several years. It is, however, relatively new, and its application to microscopy doubly so, and the skills and focus in many companies have not necessarily been oriented towards this technology. I think that it takes a while for new technologies like this to get digested into particular industries and particular applications, making their way to broadly available commercial offerings.
Iterative reconstruction has been an area of research for much longer, so a lot more people are aware of it. The challenge has been more in the implementation – how do we get the technology into a package that is reasonable for a normal user (i.e. doesn’t require a supercomputer) and for which the parameter optimization is intuitive and user-friendly.
In my opinion, the deep integration of neural networks generally and deep learning specifically into microscopy will be its next big frontier. I think there are many roads still to be run within this technology and technology class, not just in X-ray, but across the imaging spectrum. This is our first foray - I would expect a lot more technology to come out of ZEISS in this area, and I wouldn’t be surprised if, in the long-term, other manufacturers decide to follow our lead.
Can you give us a glimpse into future technologies that are going to be launched in these upcoming versions of the toolbox, and can you tell us what is in store for the future of Carl Zeiss?
ZEISS is a big company; we do everything from measuring machines to microscopes to planetariums. Essentially, if it has got a lens in it, we will build it. This release of ART is targeted at solving common XRM specific artifacts. More generally, these approaches, algorithms, and networks can be implemented and trained using workflows to do a whole range of things, from improving resolution and contrast to removing artifacts, to image segmentation and classification. They are not just X-ray specific, and can be applied across our entire portfolio. They also can enable what we call correlative workflows. This is integrating different tools, imaging different things, and linking them together using machine learning, AI, and advanced algorithms. They represent, I believe, the next great leap in scientific analyses, so don’t be surprised if you hear a lot more from ZEISS on these topics in the months and years to come!
About Matthew Andrew, Ph.D.
Matthew Andrew is currently a research Scientist at Carl Zeiss. Dr. Andrew received his Ph.D. from the Department of Earth Science and Engineering at Imperial College, London. He has published extensively on these topics, including his Ph.D. dissertation, "Reservoir-Condition Pore-Scale Imaging of Multiphase Flow."
Disclaimer: The views expressed here are those of the interviewee and do not necessarily represent the views of AZoM.com Limited (T/A) AZoNetwork, the owner and operator of this website. This disclaimer forms part of the Terms and Conditions of use of this website.