Interview conducted by Olivia FrostApr 4 2022
In this interview, Charlie Kauffman, the Senior Product Specialist for Ingredient Performance at PerkinElmer Inc., talks to AZoM about ingredient and dry mix performance analysis for baked foods.
Could you provide a brief introduction to PerkinElmer’s Rapid Visco Analyzer?
The Rapid Visco Analyzer (RVA) is essentially a little robot chef. It heats, cools, and mixes according to whatever program you send to its robot brain, and it measures ingredient performance while doing this to give you a quantified measurement of how that ingredient behaves.
During analysis, the RVA applies things like heat treatments, milling conditions, particle size effects, enzyme treatments, and chemical modifications designed to affect the performance of an ingredient.
The RVA is governed by a regular Windows PC and a custom software program from PerkinElmer. There is a huge library of stock methods for modified starches, native starches, cereal grains, and more.
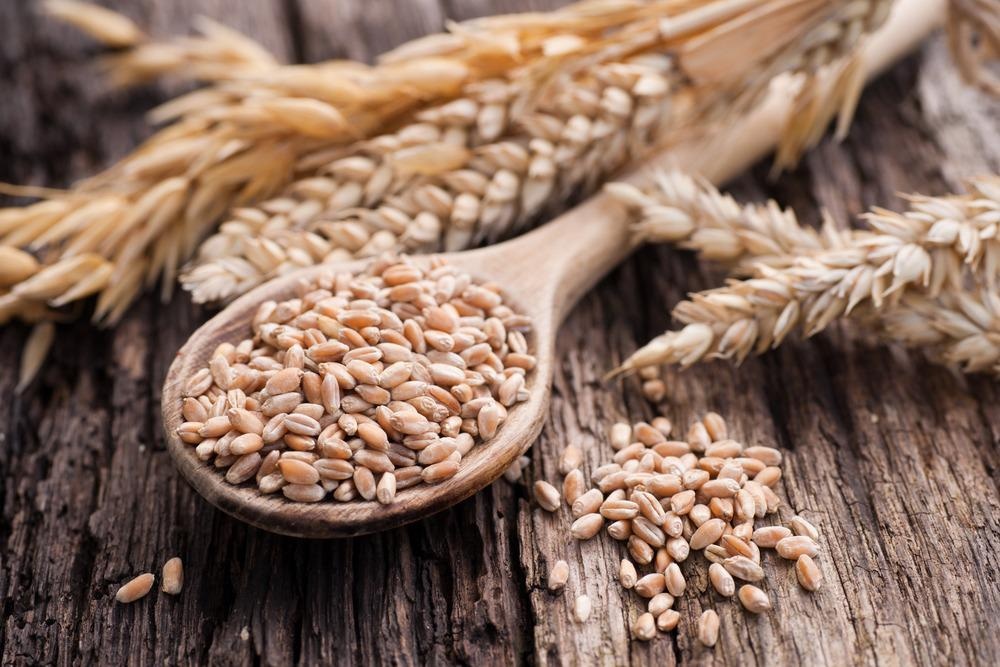
Image Credit: ShutterStock/Christian Jung
The program is also infinitely customizable so that users can coax out the best possible descriptive power from their analyses, adjusting the temperature and mixing settings to get the best combination of repeatability and descriptive power.
That is really what it is all about - measuring, characterizing, and quantifying what is normal or optimal.
How can the RVA benefit food manufacturers?
As food manufacturers, we want to make the same product all the time. Consistency is what builds brand loyalty and keeps customer complaints to a minimum.
To properly do that, we have to measure performance in terms of starch pasting, gelation behavior, hydration behavior and shear thinning.
For a product like flour, RVA results can indicate starch damage, sprout damage, relative waxiness or pasting behavior. It is a common frontline quality tool for individual mix components, both for big mixes and extrusion premixes.
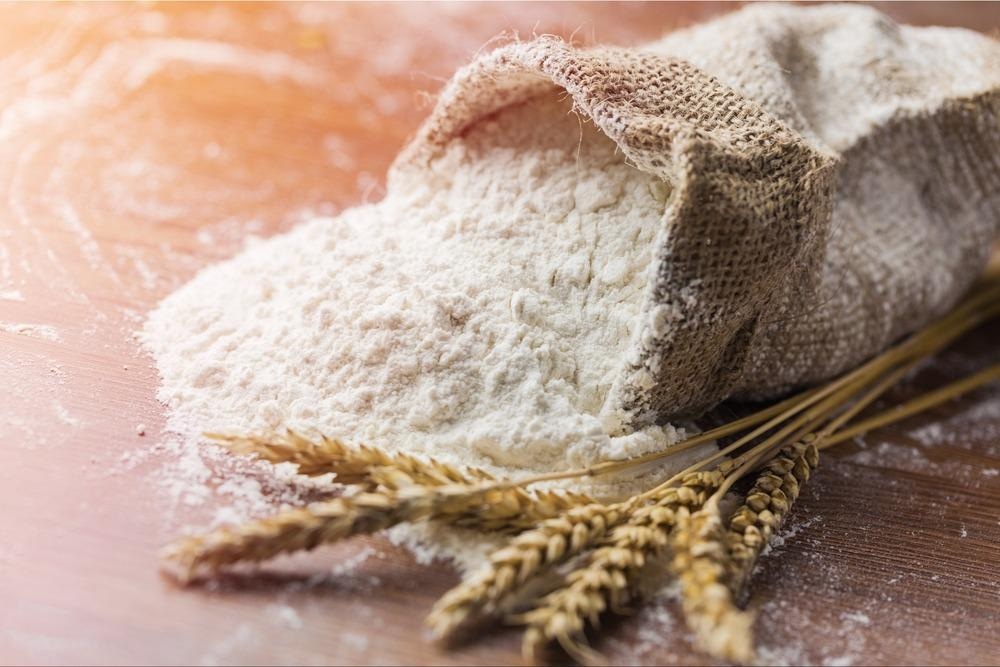
Image Credit: ShutterStock/Billion Photos
The RVA represents an excellent asset for the quality control of incoming ingredients and keeping abnormal ingredients out of the plant.
For dry mixes, whether this is a bake mix or premix, or an extrusion premix, the RVA can detect deviations from normal in that dry mix composition. For example, if there is too much or too little of any one component in a mix, it affects the concentration of every other component. These things tend to stick out like a sore thumb with RVA analysis.
As it uses a performance-based test that involves cooking the sample and putting it through its paces, the RVA can reduce the need for expensive and time-consuming test bakes.
Essentially, the RVA identifies problems in dry mixing, allowing manufacturers to make an educated choice as to how they are going to rework a dry mix if they identify something abnormal.
How does RVA analysis fit into wider quality control processes and systems?
RVA results for a series of tests correlated to traditional SRC measurements. Measuring performance is important, but not enough people do it. Too often, we are satisfied with knowing what is in a product when we should be more concerned with how a product behaves.
Traditional quality control methods do not reliably detect starch damage or problems in dry mixing, storage conditions, enzyme treatments and pasting behavior.
For example, you can have two rail cars full of corn grids with identical constituent breakdowns, but these will perform radically differently. Many of us have been in a situation before where an ingredient or mix looks fine according to a NIR device or other tool, but it fails to perform when it enters production.
To properly implement the RVA and make sure that we are not leaving any unturned stones behind us, we generally focus on a production process in a particular order.
The lowest hanging fruit is the incoming ingredients. It is easy to characterize the performance of individual ingredients, identify what is normal and keep abnormal ingredients out. If we do that successfully, many problems related to downstream waste will evaporate.
Then we tend to focus on what is stable long enough to allow us to make a decision based on any information gained.
For example, the next area we would focus on would be stable finished products, followed by premixes, where we can identify and rework these before they proceed further in production. Transient process intermediates are generally the last area that we look to clean up after nailing everything else down.
Could you elaborate on the importance of analyzing ingredient behavior during dry mixing and how this can be approached?
Dry mixing is where our best-laid plans go to die. Things get left out, things get added twice, or the ribbon is not turned on for long enough, leading to problems with homogeneity.
There is also a chance that individual ingredients or a multi-component blend of functional ingredients from a supplier were out of balance, and there was no way for us to test this before mixing.
Dry mixing is generally a big, poorly-controlled money fire that the RVA can help put out to a great degree. Historically, the way that people have evaluated dry mixes (especially bake mixes) is to bake these into small cakes.
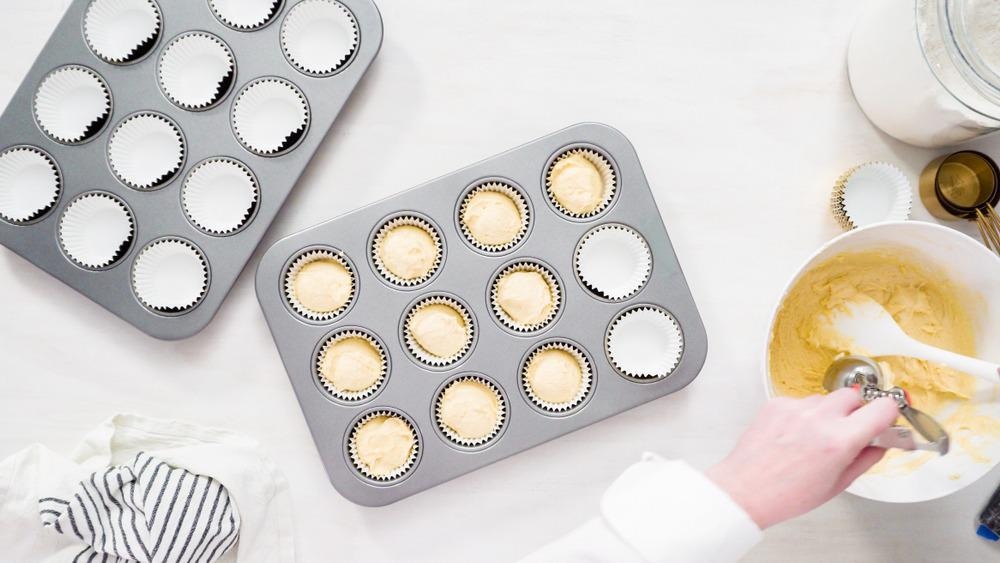
Image Credit: ShutterStock/Arina P Habich
There are reasonably rigorous protocols for doing this, generally involving cooling the cakes to room temperature over a period of 8-12 hours and having an operator physically measure these with a ruler and a set of calibers.
This approach has a lot of operator input and is labor-intensive and subjective. We are well into the 21st century now, and we want to eliminate this kind of manual process whenever possible, so we were approached years ago about using the RVA as a faster, cheaper, more descriptive alternative to test baking and as a tool to measure that wide-ranging mix of performance characteristics.
How can the RVA help address these issues?
We wanted to show that evaluating the performance of the mix in an aqueous slurry and not in a cake can be an appropriate replacement for test baking. The key to the whole operation is to measure the cooking performance of the mix and accurately detect deviations from normal in product composition and performance, and the RVA certainly does that.
We heard from test bakers themselves that test bakes alone did not yield appropriately descriptive information. Customer claims were not received because of any quality that they could have caught via test baking; there was no way for them to know whether these claims were based on actual problems with the product or things that had gone wrong in individual franchises.
We commissioned a study at the Northern Crops Institute in Fargo, attached to North Dakota State. We designed an experiment that would show how the RVA compared to test baking and which protocol was more descriptive in its results.
They had an RVA within their test baking facility, and they deliberately created bake mixes with altered levels of component ingredients to simulate things that could go wrong in real life – for example, under-doses and overdoses where the component ingredient was still present at the mix.
The idea is to answer questions around how well the RVA measures these changes and how well test baking does this. We also wanted to know if the bakers were right in that test baking is not that descriptive, and statistically, which protocol is better at detecting the problems generated in these bake mixes.
This was published a few years ago in Cereal Foods World. It found that for almost all of the ingredient modifications, the RVA was the better detector. In cakes, test baking only picked up altered levels of major functional drivers in the mix, such as egg powder and soda.
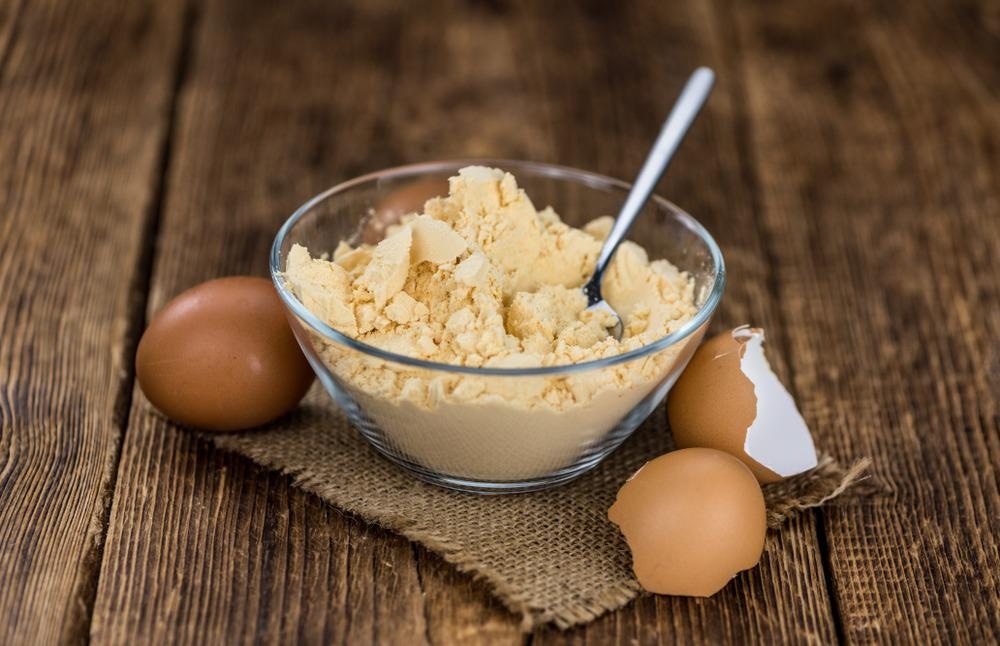
Image Credit: ShutterStock/HandmadePictures
Almost across the board, the RVA was found to be a more sensitive tool than test baking. It does not pick up ingredients that are present in relatively small amounts and are somewhat functionally inert, like vanilla. We will not see double doses or under-doses of vanilla in the RVA results, but everything else that has a functional impact on the mix is visible.
The study confirmed that bakers were right, and test baking may not be as descriptive as the industry had thought.
What are the next steps in developing the RVA’s ability to determine deviations in mix composition?
Deviations in mix composition can create predictable deviations from control. We plan to develop key metric modeling systems that will identify individual things that may have gone wrong, allowing us to learn what these deviations are.
The RVA will then be able to tell the operator what the variability is actually caused by. Even as it stands now, if there is a functional variation in the mix, the RVA will almost certainly find it.
Can the RVA be used to characterize individual and other starches?
We can use the RVA to control incoming flour and other starchy ingredients, making sure that nothing out of specification gets into the plant and the mix – and analyzing if these erroneous qualities do occur in order to support an educated choice about how to rework this.
These abnormal plots could have been evaluated with a series of bench-scale test plans and evaluated with the RVA to determine how aggressive you can be with the rework of the abnormal product into the normal product stream. This takes a lot of the guesswork and deliberate conservatism out of the rework process.
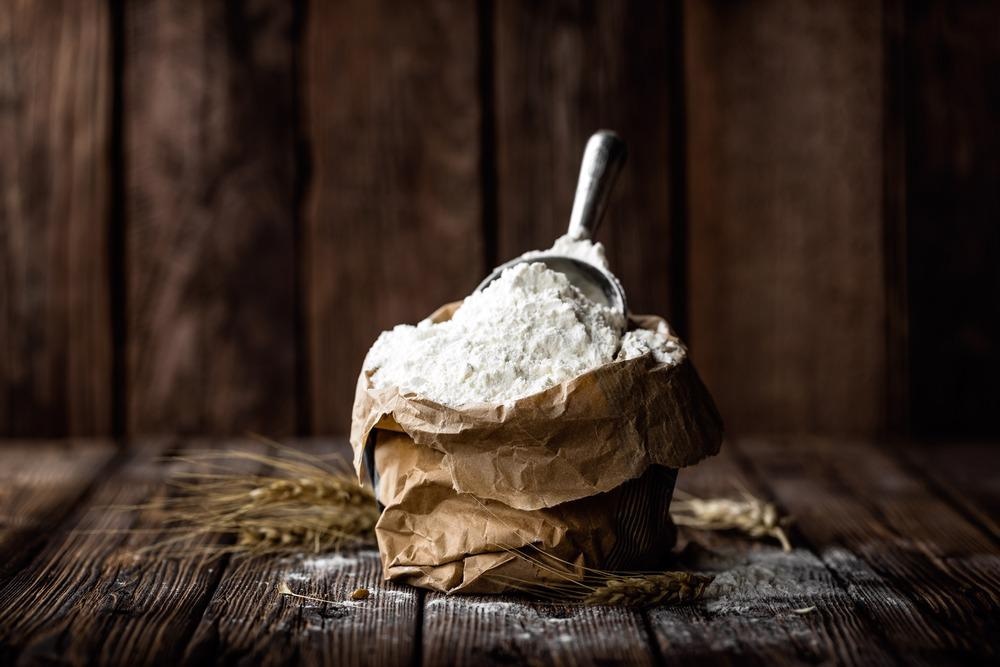
Image Credit: ShutterStock/Sunny Forest
In terms of characterizing individual starches, the RVA is the industry-standard tool for starch quality characterization. This usually involves a viscosity curve generated via a stream of viscosity data over time as a sample is heated and cooled.
The classic starch pasting curve represents changes at the level of the starch granule itself and at the level of the component carbohydrate molecules.
What we are observing is starch pasting behavior. The starch and food industries often use the terms ‘gelatinization’ and ‘pasting’ interchangeably, but they shouldn’t because these are two separate things.
Gelatinization refers to the melting of the internal crystal and structure of the starch granule. There is no viscosity change associated with this - just the crystal and structure melts. However, it is immediately followed by granule swelling, which is starch pasting, and there is, of course, a viscosity change associated with that.
What is the significance of starch pasting performance, and how does this affect extrusion behavior?
Starch pasting performance impacts final product performance. It impacts things like mouthfeel, set strength and crispness. The RVA is the most descriptive way to monitor the overall performance of these starches and make sure that they are performing the way they are supposed to – that they have been modified, for example, to the extent that they are supposed to have been.
One of the functional drivers for starch applications in food is the relative waxiness of that starch. People talk about waxy wheat or waxy rice, referring to the amylopectin fraction in the starch component molecular makeup.
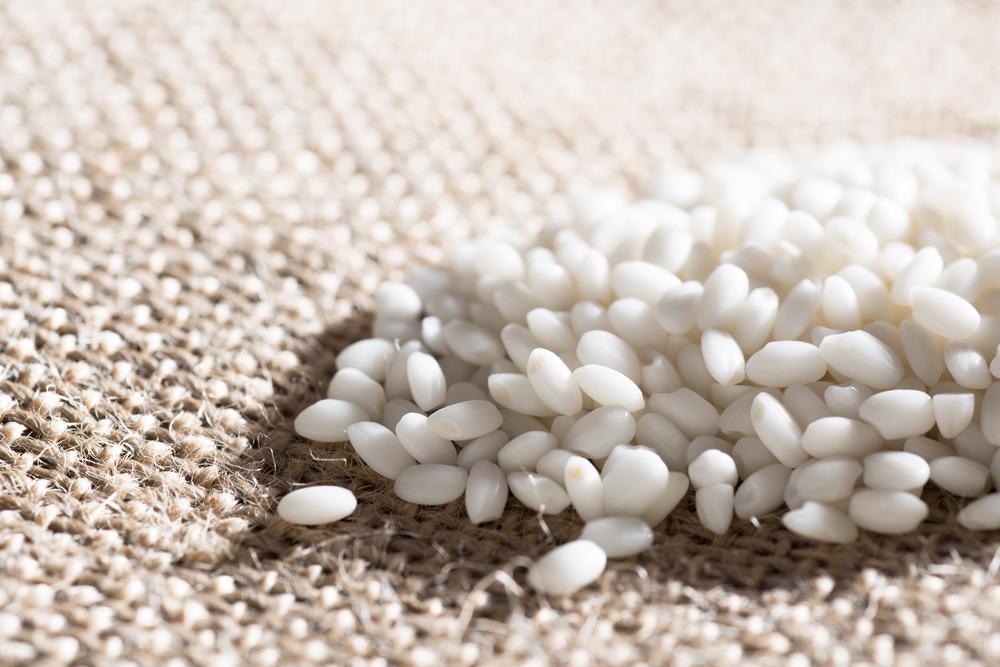
ImageCredit: ShutterStock/bigbigfish
Starch is generally made up of two carbohydrate molecules, amylose and amylopectin. Amylose is a straight-chain molecule that hydrogen bonds with well. It stacks nicely and forms films that confer some shininess.
These molecules also confer some brittleness to the eventual food product, but they do not confer a lot of expansion, which is part of what we are looking for when extruding food.
In contrast, amylopectin is a real tumbleweed of a molecule. It does not stack well with itself, but it does create a lot of space for expansion as a finished food. This is important when considering viscosity as a function of molecular weight because amylopectin is a huge molecule, and amylose is a relatively small molecule.
For extruded food, the high amylose fraction in starch enhances crispness. This is a desirable quality, but we may also want this to be puffy and delicious to the right extent, so it doesn’t trap air. Amylopectin-heavy extrudates do expand well, but this is generally at the expense of crispness.
The formulation team and R&D folks at these food companies try to balance the starch makeup of a product, using the RVA to determine this.
How are the different models of RVA suited to different applications or samples?
We have two basic RVAs. The RVA 4500 has an operating temperature range of 0 °C to 100 °C. That is the traditional RVA.
We also have a nearly identical-looking RVA called the RVA 4800. This has an enhanced mode for high-temperature analysis and a sealed sample module designed to evaluate samples at temperatures up to 140 °C.
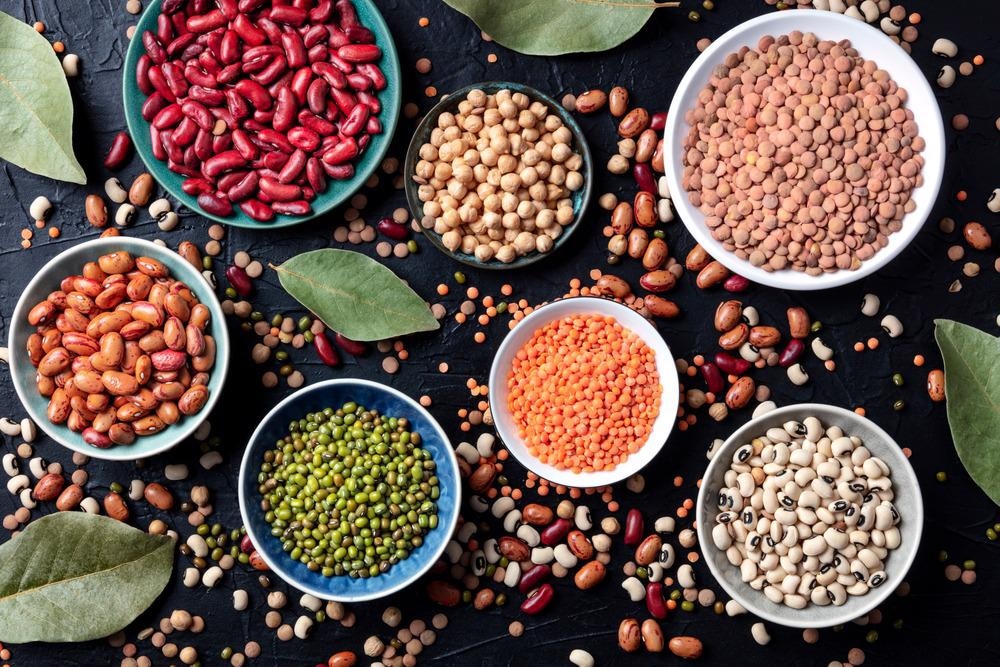
Image Credit: ShutterStock/Plateresca
This is ideal for heat-resistant ingredients like resistant starches, high-amylose starches, pulse ingredients, and soy proteins. None of these ingredients change until they are heated to over 100 °C.
Until now, there was no good way to evaluate these ingredient systems. But now there is, and it is within the familiar context of the RVA that everybody already knows.
Could you expand on the role of extrusion and how food manufacturers can optimize ingredient properties for this process?
Extruders are ingenious pieces of technology because there are two ways that they can cook starch – thermally and mechanically.
Extruders cook starch in both ways - with heat and shear, affording users control over two things: control over how hot the barrel is and how fast the sample is moving through it.
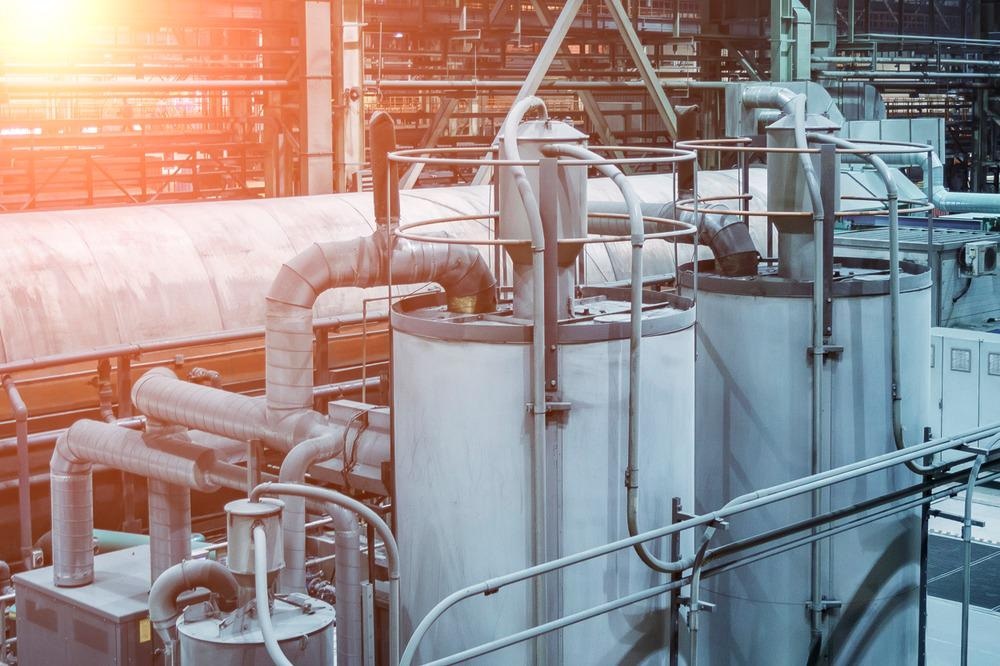
Image Credit: ShutterStock/Vladimir Mulder
We always start with the incoming ingredient. We know that the starch is being cooked in two ways with heat and shear and that shear has a disproportionate impact on the damage inflicted on these starch granules. Extended cooking is extremely damaging to the starch granules, and imparting a higher level of cooking can destroy these altogether.
Barrel cooling and screw speed are always at odds, but these are two things that we have control over.
If we increase the temperature of the barrel, we increase the thermal cook imparted on the extrudate. However, we are also decreasing the shear encountered by that extrudate as it goes through - if it gets hotter, it gets more slippery.
Increasing the screw speed, on the other hand, increases the shear cooking because the extrudate moves through the barrel faster. This also decreases thermal cooking because it spends less time in the hot place.
We can also control how resistant something is to cooking by controlling the moisture of the pre-conditioned dough — more moisture results in a sample that is more cook resistant.
Changing the barrel cooling, the temperature of the barrel and the screw speed has a characteristic effect on the RVA result. This is the information that extruder operators use to make informed decisions about piloting the extruder and maintaining a consistent product.
Are these considerations around extrusion different for final products?
Extrusion effects on finished products are similar. Breakfast cereal is an excellent example of this. Over the last 35 years, the generic branded, value-added cereal is getting closer to the target product that it is designed to imitate.
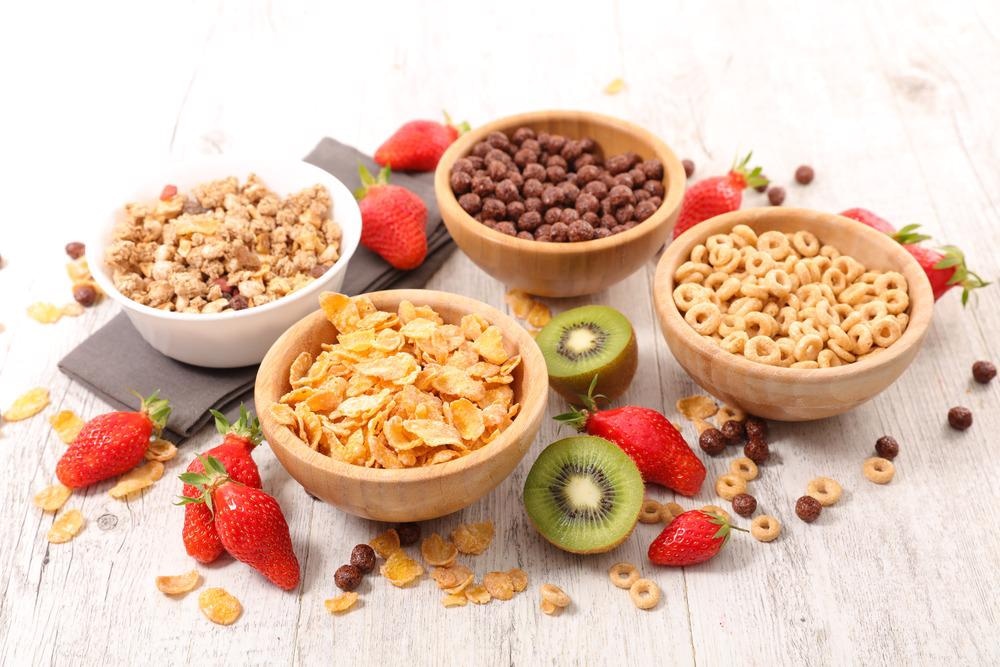
Image Credit: ShutterStock/margouillat photo
Manufacturers have gotten closer to the target product using the RVA to reverse engineer the extruder settings of the target product. There are specific considerations when preparing extruded samples.
Dry pellets can be milled and screened for particle size. Wet pellets are harder to characterize but not as hard as pre-conditioned dough. Wet pellets can be frozen in liquid nitrogen, ground with dry ice, and then controlled for particle size. The same approach can be used with pre-conditioned dough.
About Charlie Kauffman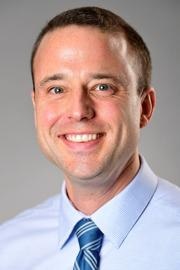
Charlie Kauffman is a middle-aged man in Peoria, Illinois who helps PerkinElmer clients analyze ingredient performance and minimize food waste, with the end goal of becoming more ecologically responsible and more profitable.
He is a graduate of the University of Nebraska, a member of the Cereals and Grains Association, and the Institute of Food Technologists. Some of his accomplishments have been intentional on his part.
About PerkinElmer Food Safety and Quality
PerkinElmer Food Safety and Quality is committed to providing the innovative analytical tools needed to ensure the global supply of high-quality, safe, and unadulterated foods.
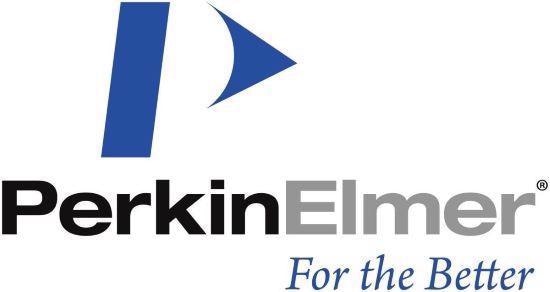
This information has been sourced, reviewed and adapted from materials provided by PerkinElmer Food Safety and Quality.
For more information on this source, please visit PerkinElmer Food Safety and Quality.
Disclaimer: The views expressed here are those of the interviewee and do not necessarily represent the views of AZoM.com Limited (T/A) AZoNetwork, the owner and operator of this website. This disclaimer forms part of the Terms and Conditions of use of this website.