When focusing on quality, a primary challenge is establishing criteria to determine if samples pass or fail tests. This complexity is compounded by multiple factors, notably the variability in human judgment. With numerous operators conducting analyses and making decisions based on their subjective views of what constitutes good or bad, there is an inherent human bias. This bias is closely tied to each operator's level of expertise.
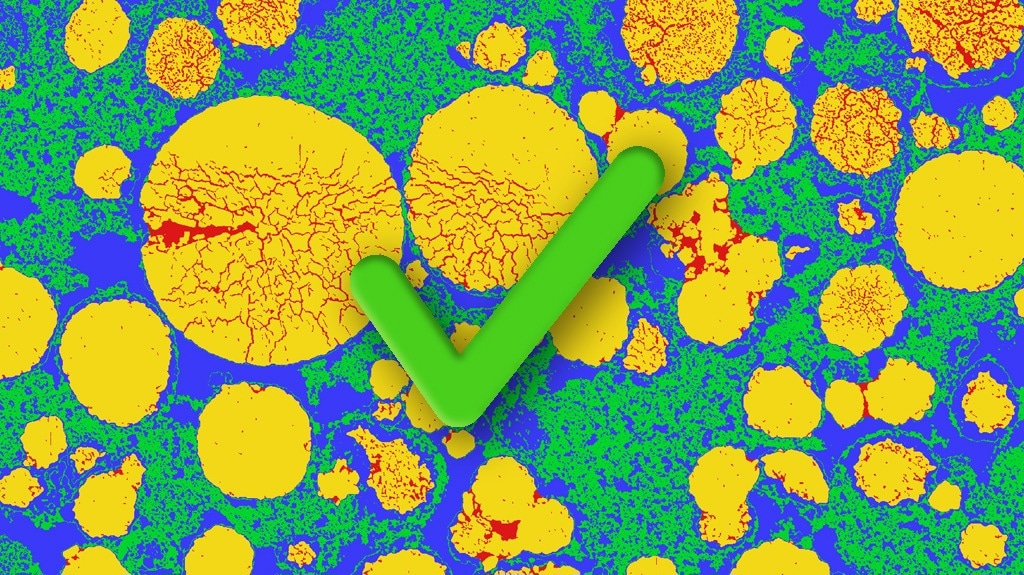
Image Credit: Thermo Fisher Scientific
Ultimately, the quality expert wants to compare apples to apples to point the finger at the challenge that manufacturing is experiencing and promote a solution.
Tools like key process indicators (KPI) and standard operative procedures (SOP) mitigate these challenges, but there are technological limits that hinder the effectiveness of such instruments. This is especially true when an operator has to evaluate the quality of a sample by looking at an image or a 3D render and deciding on a pass or fail.
A common challenge in battery development is examining the cathode electrode after the battery has been cycled several times to understand the causes of failure. The cathode particles tend to crack as a consequence of the electrochemical cycle and the intense chemical reaction rate. As these cracks expand through the particles, the electrode efficiency diminishes.
This phenomenon is typically accompanied by a migration of the binder, which weakens the conductive network between particles and reduces the electrons’ mobility between the charge collector and the particles.
To measure this effect, the battery is opened, and a sample of the cathode layer is extracted. The sample is then treated with a broad ion beam to expose the interior of particles, and a series of microscopy images are acquired over the processed area. Considering the features’ size, scanning electron microscopy is intuitively the best fit for this imaging challenge.
The process to this point can be easily streamlined and designed in an SOP, but the final challenge remains: How does one decide, based on the acquired images, if the sample matches the expected behavior and complies with the specifications?
Image interpretation is an extremely subjective activity. Even when defining the criteria for interpretation in fine detail, inevitably, variations will arise when different operators judge the same image. Luckily, software comes to the rescue.
Image segmentation software can understand the different logical sections (or segments) of an image. In the example below, the software identified the carbon and binder, the hollow areas, and the particles and their cracks, then displayed this separation using different colors.
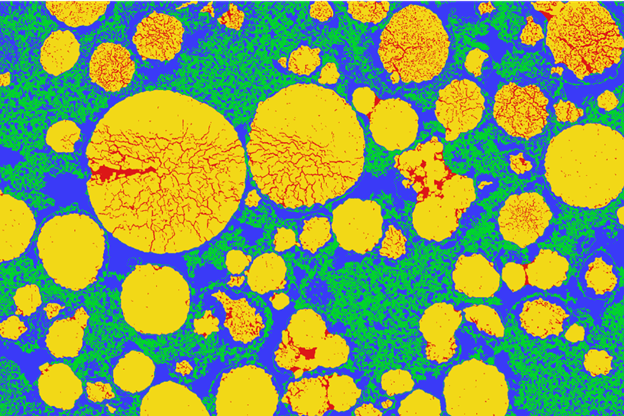
Image Credit: Thermo Fisher Scientific
Original and Segmented Images
The software will then perform automated measurements of the parameters of interest (defined in the KPI) and provide a number, a table, or other numerical output. The operator can then compare the measured property with the expected reference value and assess with certainty whether the sample complies with the specifications.
Examples of Measurements
This type of workflow makes the result of the analysis repeatable and reproducible; the software will always process an image in the same way and return the same results, independent of who loads the image in the software.
Finally, the software can make logical connections between measured parameters and report information on more complex properties. For example, it can measure the area of the image occupied by each component or calculate the ratio of particles with cracks above a certain severity versus undamaged ones.
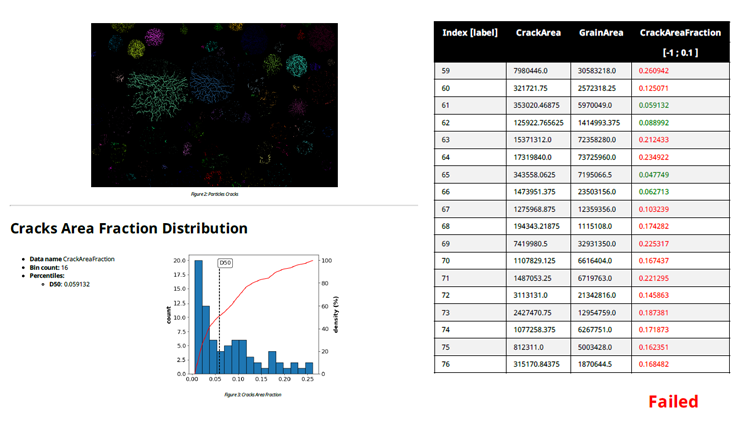
Example of a non-compliant sample displayed in a scatter plot with Pass/Fail threshold. Image Credit: Thermo Fisher Scientific
Example of Complete Report
Thermo Scientific™ Avizo™ Trueput Software for Battery Quality is an archive of workflows for the quality inspection of battery samples. From raw images to a complete PASS/FAIL report, Avizo Trueput Software removes operator bias from results. Its repeatable approach will give users extra confidence in their data, which, in turn, supports data-based decision-making.
Avizo Trueput Software’s continuously growing catalog of workflows will enrich the collection of quality analysis tests with insights on common protocols run by peers in the field. Every test is streamlined from image to report and is easy to implement in any SOP for up-scaling.
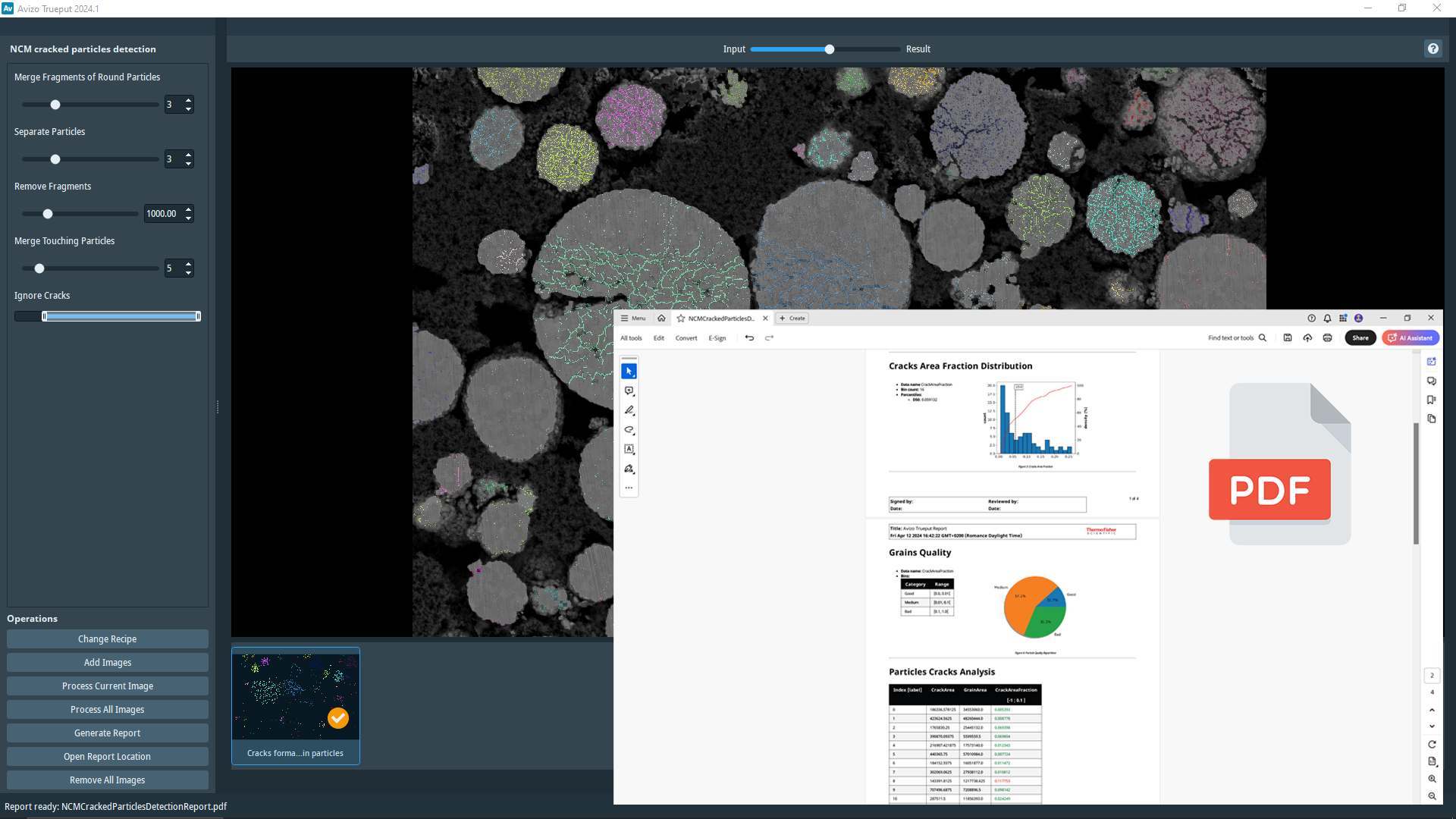
Image Credit: Thermo Fisher Scientific
Learn more about Avizo Trueput Software
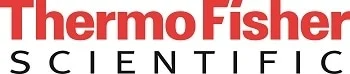
This information has been sourced, reviewed and adapted from materials provided by Thermo Fisher Scientific - Software.
For more information on this source, please visit Thermo Fisher Scientific - Software.