Apr 20 2015
A team of researchers at the University of Notre Dame have conducted some research on the ways in which precise statistical illustration of heterogeneous particulate materials used within statistical micromechanics theories impacts thermo-mechanical properties.
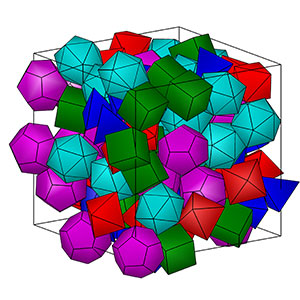
Karel Matous, College of Engineering Associate Professor of Computational Mechanics in the Department of Aerospace and Mechanical Engineering led the team at the University of Notre Dame.
They were able to achieve the comprehensive statistical description with the aid of an innovative adaptive interpolation/integration method used on the largest parallel supercomputers in the country.
When the morphology of many-body units is quantified, it can be applied in several scientific areas at numerous length scales extending from molecular configurations to celestial bodies and structural composites.
“For centuries, great minds like Kepler, Maxwell and Einstein have investigated the statistical characterization of many-body systems and the implications of small-scale structures on the macroscopic transport and mechanical properties,” Matous said. “For the first time, we predicted the properties of granular Platonic solids (crystalline) packs and discovered a significant shape effect in their overall thermo-mechanical behavior. Based on our work, a large class of materials with arbitrary inclusions can now be easily studied.”
The materials study forms a part of a data-driven, image-based, multi-scale modeling approach adopted by Matous’ team, wherein computations were governed by micro-structural experimental information.
“In my research, I study heterogeneous and multifunctional materials in extreme environments by computational and experimental means,” Matous said. “These materials are essential in our daily lives and are utilized in bioengineering, the automotive and aerospace industries, micro-electro-mechanical systems (MEMS) and other applications. Heterogeneous multifunctional materials fill several pivotal roles, including structural support, self-healing capability, power generation and storage, stress mitigation and bio-filtration, just to name a few.”
“These seemingly simple and elegant material functions are governed by a plethora of physics, mechanics and chemistry that test our understanding of microstructure-statistics-property relations and our capacity to tune properties at will. Many of these materials can be better understood if higher-order statistical information is retained in their well-resolved multi-scale analysis. My goal is to advance detailed understanding of mechanics and materials science through developing microstructure-statistics-property relations that can aid the development of new materials.”
Going forward Matous observes that it is crucial to comprehend the impact of anisotropy and material interfaces.
“Extending these models and accounting for interface properties and their behavior is the challenge,” he said. “Also many materials are anisotropic and quantifying a higher-order anisotropic description is still an open question. Moreover, linking this work with image-based characterization techniques, such as microcomputer tomography that has become a popular method for obtaining a description of real microstructures, to analyze real material systems is a natural next step.”
The paper presented by Matous and team was published as the cover article in the Proceedings of the Royal Society A.