The Spero® laser-based infrared microscope was utilized to produce high definition, chemical images of unstained thin colon tissue sections prepared using standardized histological protocols. Next, routine chemometric analysis techniques were used with the data so that tissue types could be identified and segmented.
.jpg)
Solution
Spectral image data cubes of thin (10 μm) excised colorectal tissue sections were mounted onto infrared glass slides before being acquired with a first-generation Spero® microscope. This had a spectral range of 900 – 1,800 cm−1 (5,500 – 1,100 nm), a pixel resolution of 1.3 μm and diffraction-limited resolvability set by the 0.7 NA, and a single-tile field of view (FOV) of 650 μm × 650 μm.
Recordings were made of image cubes covering the full-band (900 – 1,800 cm-1 at 4 cm-1 steps) alongside cubes targeting 10 sparsely selected frequencies within the band. These were then analyzed using in ImageLab® multimodal chemometrics software from Epina Software, based in Vienna, Austria.
To assist with chemometrics data reduction, the raw image cubes were pre-processed using the following sequence:
- Noise reduction using the well-known maximum noise fraction transform
- Rejection of pixels with an amide I peak value (1,656 cm-1) of less than 0.05 AU (done as a spectral quality test)
- Full-band spectra converted into second derivatives to remove baselines
- 9-point smoothing using the Savitzky-Golay algorithm
- Spectral normalization of all spectral vectors to achieve zero mean and unit standard deviation. This was done to reduce the influence of intensity changes caused by differences in cellular density and tissue thickness.
After these pre-processing steps were completed, full band (900 – 1,800 cm-1) second derivative datasets were analyzed via an unsupervised k-means clustering method. This was a non-hierarchical iterative method that obtains “hard” class membership for each spectrum.
False color images for 6 classes were constructed next, with each pixel in the total imaged field being assigned a class membership with a corresponding color. Using this method of false coloring based on the spectral response of that particular pixel meant that the observer had a fast, simple means of “seeing” the biochemical differences within the sample. In this instance, the key differences correspond to basic types of cell and tissue structures.
Results
From the k-means clustered images, the different structures of the colon tissue are clearly visible. This included the colonic crypts, submucosa, and lamina propria. Within the colonic crypts especially, spectral signatures included combinations of mucin glycosylation bands at 1,044, 1,076, 1,120, and 1,374 cm-1 along with a clear lipid ester band at 1,740 cm-1.
In contrast however, the submucosa was identified by a number of strong bands that can be directly linked to the structural protein collagen having bands at 1,204, 1,236, 1,280, 1,336 (amide III), and 1,452 cm-1 respectively.
Spectroscopic differences between the lamina propria and adenocarcinoma are much subtler. These are located at nucleic acid-related vibrations at ca. 964, 1,062, 1,090, and 1,236 cm-1.
As can be seen, the 10-frequency sparse image cube datasets were used to create high-speed, qualitative chemical maps of collagen, protein and mucin-rich regions which could then be used to guide more detailed exploration and analysis.
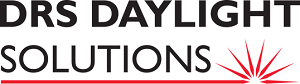
This information has been sourced, reviewed and adapted from materials provided by Daylight Solutions Inc.
For more information on this source, please visit Daylight Solutions Inc.