What is the Advanced Reconstruction Toolkit (ART)?
The advanced reconstruction toolkit is an umbrella term used to refer to a range of technologies offered by Zeiss for X-ray image reconstruction and contrast improvement. This includes the OptiRecon, DeepRecon and PhaseEvolve products.
DeepRecon is the first implementation of deep learning methods for X-ray reconstruction. This enables greatly improved acquisition speeds of up to 10 times or greatly improved image quality at a fixed acquisition speed. This unlocks performance and applications that were previously impractical to perform using traditional reconstruction.
This year we introduced DeepRecon Pro, which unlocks the performance of deep learning for reconstruction for a much broader array of sample classes by enabling users to train their own reconstruction networks completely automatically without the requirement for deep learning expertise.
This is a really exciting development because the implementation of these approaches is often gated by the availability of proficiency with deep learning technology. We believe that DeepRecon Pro will, for the first time, effectively bring the power of this technology into every lab.
PhaseEvolve is a technology that greatly improves image contrast on samples, at low energies or at high spatial resolutions, where phase contrast effects may otherwise dominate.
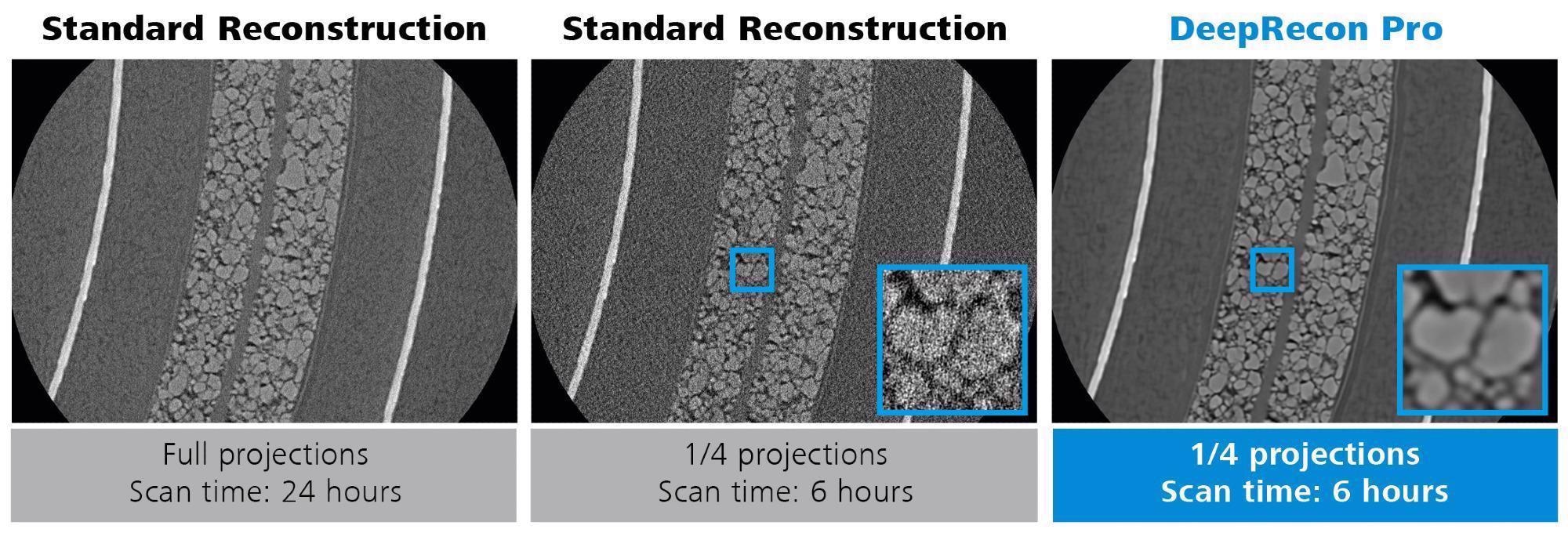
Can you explain the advantages DeepRecon has over other similar technology?
Traditionally, reconstruction is performed using analytical techniques such as Feldkamp-Davis-Kress (FDK) reconstruction. These approaches work well when data is extremely low noise and wellsampled through multiple angles.
Performing high-resolution imaging can lead to impractically long acquisition times which limits the application of X-ray imaging, particularly in industrial areas where throughput is critical, in in situ experiments where absolute temporal resolution is critical, or even when performing challenging imaging experiments such as interior tomograph where a high degree of noise is inherent to the experiment.
If these requirements are not met, image reconstructions have an unacceptable level of artifacts and noise and, thus, extremely limited utility.
Deep learning is an exciting new technology that has transformed fields as wide as weather prediction and stock market analysis, but its application in microstructure analysis is still in its infancy.
DeepRecon is the first implementation of deep learning for reconstruction using a trained network that can have the same image quality as traditional techniques at a much-reduced acquisition time or, equivalently, much-improved image quality at a fixed acquisition time. It makes use of prior knowledge directly derived from the data being analyzed, greatly improving the quality of results.
What fields will most benefit from the adoption of DeepRecon?
DeepRecon Pro allows the power of deep learning for reconstruction to be available to everybody across all applications. This includes both academic and industrial scientists, for instance, in battery analysis.
An academic researcher might be interested in the fundamental microstructure of different battery formulations and wants the highest quality image available, even for low contrast materials within the battery.
For this application, DeepRecon Pro can be used to greatly boost image quality, reduce the noise level in the reconstructed image and thereby reveal structures that might otherwise be hidden by the noise.
An industrial researcher may be interested in how battery cycling induces microstructural change. This is effectively an in situ experiment, and DeepRecon Pro can be used to greatly improve absolute temporal resolution, enabling the researcher to acquire a much greater number of experimental points and greater resolution on the underlying processes affecting the structure.
In battery production, a QA-QC engineer might be interested in using DeepRecon Pro to significantly improve acquisition time so that they can inspect a larger number of produced parts in the same amount of time, effectively reducing incremental part analysis cost without compromising on image quality.
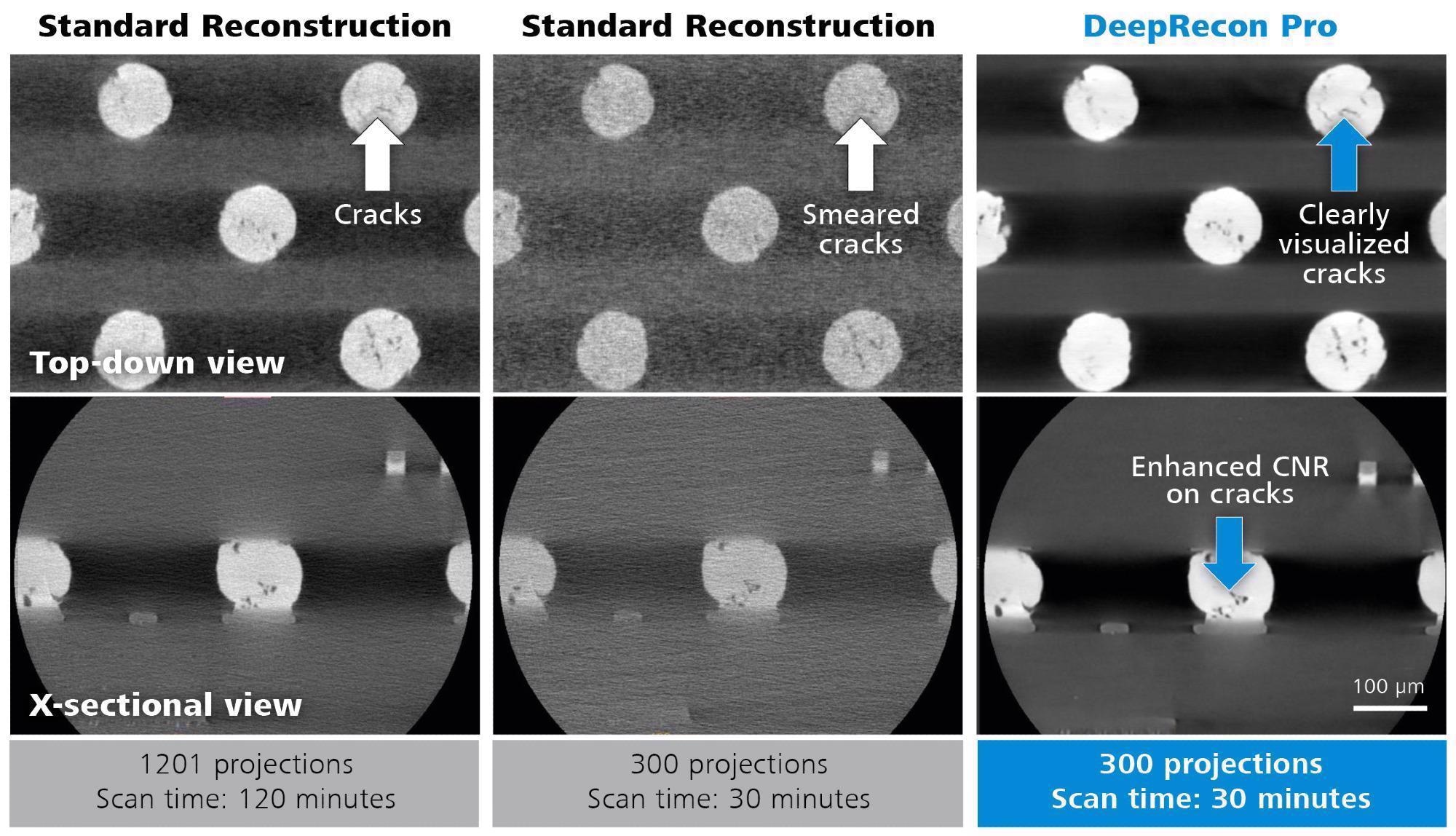
On what platforms will DeepRecon Pro be available?
We have released DeepRecon Pro and PhaseEvolve for the Versa X-ray microscope (XRM) and Context micro CT platforms. However, we are looking at how this could be broadened into other parts of our X-ray portfolio and beyond.
How have X-ray imaging needs evolved, and how does DeepRecon Pro meet these needs?
Our users constantly push the boundaries of imaging performance and always require the highest quality, highest resolution, lowest noise, and lowest artifact level images of their systems. As time has gone on, this need has only increased and is always innovating to release better, more powerful, high-performance systems.
This includes innovation in X-ray imaging hardware, but we also recognize that modern algorithmic approaches, innovative data science, and computational imaging capabilities offer a very fertile ground for technological development.
In parallel, there has been an ongoing desire for improving ease of use, or to make these advanced algorithmic approaches more accessible to researchers no matter their familiarity with these technologies or skill. The intersection of these is the heart of why we are so excited about DeepRecon Pro: it is both incredibly powerful and incredibly easy to use.
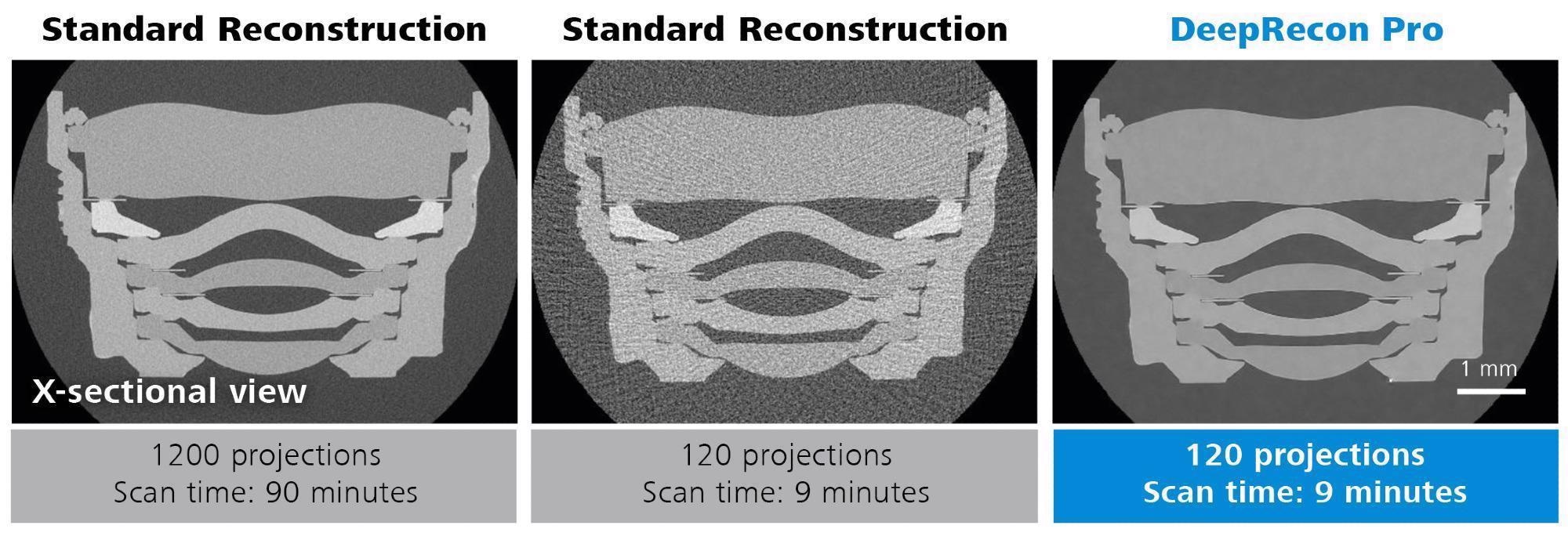
How does AI influence the imaging capabilities of DeepRecon Pro?
AI is an encompassing term; machine learning is a specific subset, and the particular variant that we use in DeepRecon Pro is deep learning. In deep learning, we use convolutional neural networks for reconstruction that are trained using a workflow with deep insight into the specific physics and mathematics inherent to X-ray imaging to remove image noise and artifacts.
What advantages does DeepRecon Pro deep neural network technology confer to the user?
Deep neural networks refer to a form of trainable nonlinear image filters that are widely used for imaging tasks such as semantic and instance segmentation, image classification, object recognition, and other regression problems.
One of the principal advantages that deep learning has over shallow learning approaches is that it does not require the preselection of specific image features into a composite feature vector. This broadens their potential applicability across multiple applications and image types, but they are often challenging to train and require extensive training data.
One of the key capabilities of DeepRecon Pro is its ability to train a new reconstruction network on a single tomographic dataset to optimally remove the sampling artifacts and noise specific to that data set. This training can be performed in approximately three hours and is highly robust and repeatable.
This training capability is extremely exciting as it allows scientists to unlock the hidden dependencies already present in the large datasets used for tomographic imaging through the use of these deep neural networks.
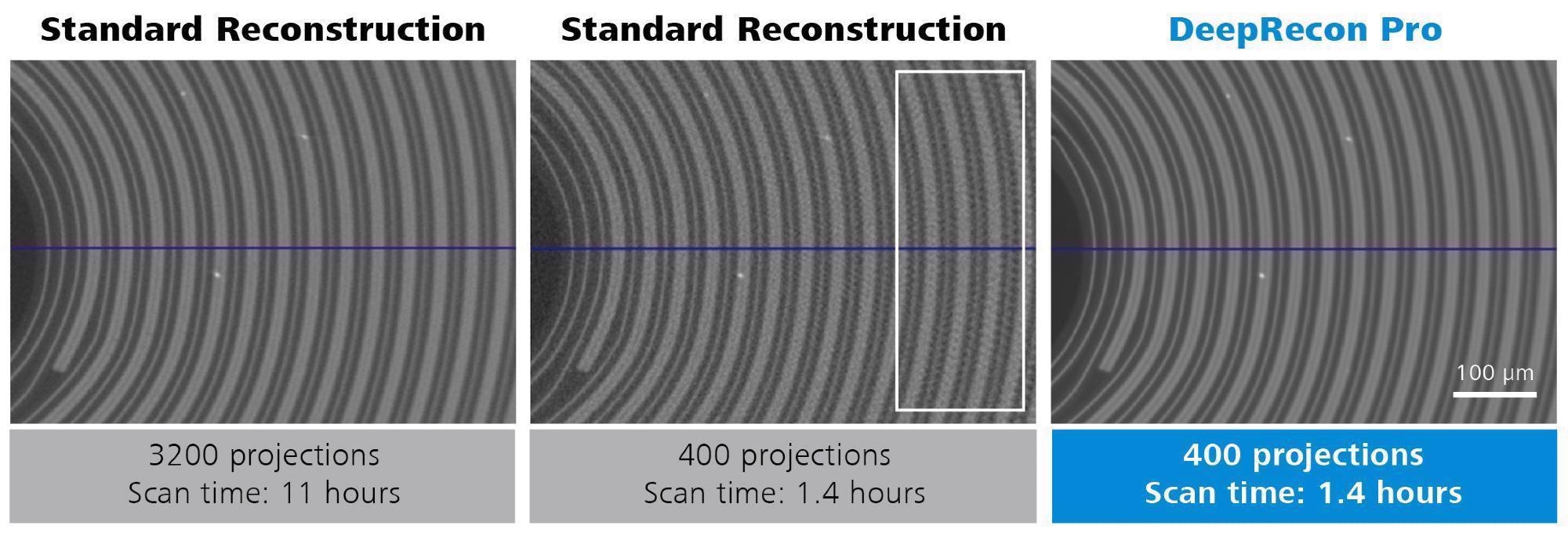
How has DeepRecon Pro boosted the speed of X-ray imaging?
DeepRecon Pro can boost acquisition speeds in a few different ways. We classify applications as either repetitive (one sample type being imaged multiple times), semi-repetitive (one sample being imaged multiple times, such as for in situ experiments, multiple interiors, or a vertical stitch ), or a unique sample.
DeepRecon Pro considerably improves acquisition speed by up to 10 times for each of these application classes but the way it does so is slightly different for each.
In the case of the repetitive or semi-repetitive application classes, a reconstruction network can be trained on an initial (low throughput) tomography. Each subsequent scan can then be performed much faster, thereby improving the total throughput of either the repetitive or semi-repetitive application.
In the case of the unique sample, a reduced acquisition time can be used and an image quality improvement network can be trained so that the image quality at that reduced time is equivalent to a longer acquisition reconstructed by using traditional analytical techniques.
This approach is not recommended for repetitive or semi-repetitive applications as often the network performance achieved when trained on more data (the longer acquisition) is better than one trained on less data (the shorter acquisition used for the unique sample).
Who will primarily benefit from this boost in speed?
Many applications can benefit from an improved imaging speed, ranging from academic researchers interested in the absolute temporal resolution of in situ experiments to industrial engineers interested in maximizing the number of samples that can be processed in a given period of time.
Can you explain how DeepRecon Pro provides enhanced image quality?
If throughput is not the primary concern, but contrasts or artifacts or noise are, then an image quality network can be trained. This uses a specialized training workflow that incorporates deep insights into the origin of the noise and artifacts in X-ray reconstruction, and their relationship to both the projection and volume domain, to train the network to remove both of these effects.
This effectively removes both of the sources of the spurious image signal and greatly improves the relative phase contrast by improving the contrast-to-noise ratio. This can be extremely important when imaging challenging systems or samples, such as high-resolution interior tomographies within large objects or low Z / low contrast materials.
How do the increase in speed of data acquisition and enhanced image quality impact resolution?
Generally, image quality resolution and speed trade off against each other in X-ray imaging. The great advantage of the products within the advanced reconstruction toolbox, particularly DeepRecon Pro, is that it allows for a tremendous improvement in image quality or throughput without sacrificing resolution or the complexity of the imaging experiment.
How does DeepRecon Pro avoid this "trade-off"?
The convolutional neural networks used for image reconstruction in DeepRecon Pro are extremely powerful when correctly trained and can be used to greatly extend the effective performance envelope of our X-ray imaging systems in a given situation. It uses the same limited datasets as inputs, but the outputs of reconstruction are far superior when compared to traditional analytical reconstruction.
What is next for ART? How can Zeiss improve on the system in the future?
The advanced algorithmic approaches within the advanced reconstruction toolbox are an extremely fertile area for future research and development. While I can't give too much away, you can expect us to frequently release new advancements within this space to improve X-ray contrast, analytical sensitivity and precision, resolution, as well as the removal of challenging artifacts and imaging problems.
I also think we will look to build new technologies that allow for these advanced capabilities to be accessed in a much more flexible and easy to scale way.
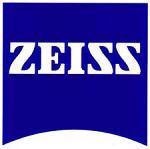
This information has been sourced, reviewed and adapted from materials provided by Carl Zeiss Microscopy GmbH.
For more information on this source, please visit Carl Zeiss Microscopy GmbH.
Disclaimer: The views expressed here are those of the interviewee and do not necessarily represent the views of AZoM.com Limited (T/A) AZoNetwork, the owner and operator of this website. This disclaimer forms part of the Terms and Conditions of use of this website.