This interview highlights how to overcome challenges in electrochemical cycling with novel material strategies. Roland Brunner, Group Leader of Material and Damage Analytics at the Materials Center Leoben Forschung GmbH (MCL) discusses the applications and challenges often faced with energy storage.
What is Zeiss’ background in materials sciences? How does this background benefit Zeiss’ clients?
ZEISS is working with scientists at the forefront of materials research in a variety of application fields. For example, in lithium ion battery research ZEISS is collaborating with researchers to understand and exploit unique material properties across length scales in order to help build the new, cleaner energy future.
ZEISS is the only manufacturer of light, electron, X-ray, and ion microscopes covering a range of length scales, modalities and dimensions: that’s why leading researchers value ZEISS microscopes as a critical piece of their characterization suites. Together, we are building the future of materials science and engineering in energy research and beyond.
What are Li-ion batteries, and why are they so important?
Li-ion batteries are regarded as a major breakthrough in energy storage technology. Li-ion batteries are expected to be the leading energy storage system at least until late 2020's, according to the EU-SET plan and global battery roadmap.
A Li-ion battery is a rechargeable battery that uses lithium and electron transport between two electrodes for the storage and release of energy.
Electron and Li-ion transport are driven by externally applied electrical potential during charging, as well as by reactions during discharging.
The battery consists of an anode-cathode, a separator, and respective current collectors for the electrodes. The electron and Li-ion conducting pathways within the electrodes are strongly governed by the electrode microstructure.
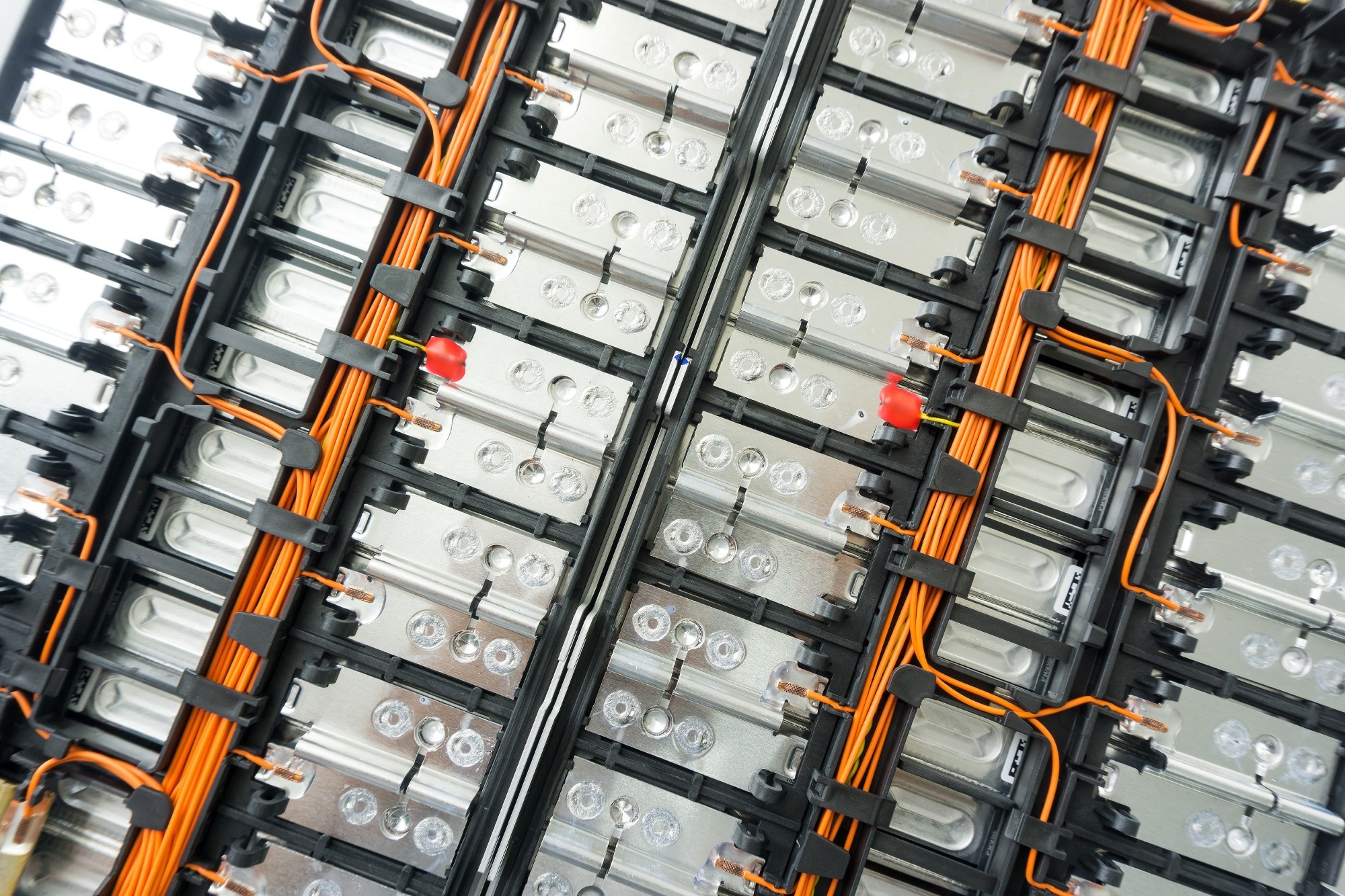
Image Credit:Shutterstock/ SmileFight
What are some of the applications of these batteries?
Applications are for renewable energy (energy management for photovoltaic etc.), e-mobility (full electric vehicles and hybrid solutions), or mobile electronic devices (headsets, smart watches, cell phones, etc.)
How can these batteries be improved for energy storage?
When it comes to energy storage, Li-ion batteries can be improved in terms of their capacity, cycling stability, safety, and charging rates.
How do Si-based anodes play a role in the development of batteries?
Silicon can play a major role in the development of batteries; for instance, it can be used as an active material in the anode embedded in a graphite and carbon binder matrix, forming a porous composite electrode.
In addition, conducting agents, such as carbon black or carbon nanotubes, are distributed within the carbon binder domain to demonstrate sufficient electrical conductivity.
Si is one of the most promising high-capacity materials for practical applications in next-generation Li-ion batteries. It also has several advantages with respect to other materials; for instance, it has a theoretical specific capacity (Li15Si4 with 3578 mAh/g, which is about ten times larger than conventional graphite 372 mAh/g), it is low cost to make, and it is non-toxic.
Image Credit:Shutterstock/petrmlinak
What are some of the challenges associated with using Si-based anodes?
A big challenge when it comes to using Si-based anodes concerns a large volume swing, which means that the high-capacity material undergoes volumetric expansion and contraction during electrochemical cycling.
The change in volume can be up to about 300%, consequently leading to capacity fading due to the loss of electrical contact, cracking of the active material, and the reformation process of the so-called solid electrolyte interface.
It is essential to restrain those changes in volume or to make the anode material resilient against the induced degradation as a result of the large volume swing.
How can novel material strategies be used to overcome these challenges?
The excellent performance of Li-ion batteries is not only linked to the electrochemical properties but also to the microstructure or morphology of the electrode material.
Therefore, the structure-property relationship is a key ingredient when it comes to improving the performance of Li-ion batteries. Clever material architecture design guidelines to control the properties can be used to overcome the challenges and, in particular, the impact that the large change in volume has on the battery's capacity.
How can the investigation of the structure-property relationship in anode materials assist this?
As already mentioned, the performance of the battery is strongly linked to the underlying microstructure of the anode. In general, material properties are correlated with the microstructure or morphology of the material in question. Thus, controlling the material architecture means controlling the material properties.
Therefore, the understanding and analysis of the microstructure in context to the material properties like the ion transport characteristic or electrical conductivity, etc., is essential to provide design guidelines for improved batteries.
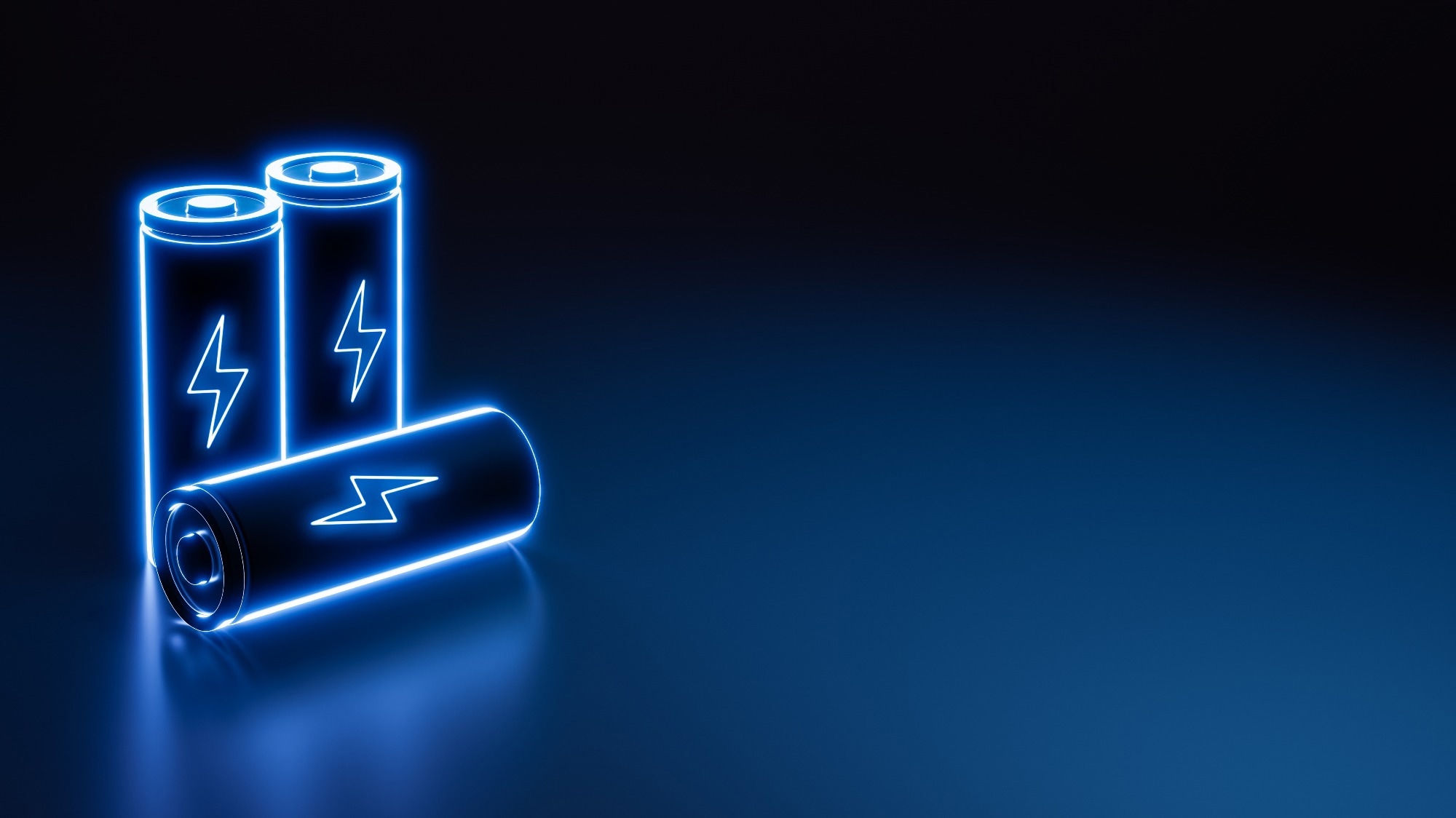
Image Credit:Shutterstock/Black_Kira
How can 2D and 3D characterization on different length scales be used to investigate the structure and chemical element distribution of anodes?
When choosing the most suitable material, manufacturing processes, and processing parameters, an accurate representation of the electrode microstructure utilizing advanced 2D and 3D imaging methods to distinguish between different material phases (segmentation) as well as reveal statistical information, such as about the pore network connectivity or tortuosity (highway for Li transport), porosity, Si-distribution, Vol% of different phases, etc., is extremely useful.
As a result, a loop between characterization and processing can be established for improved design guidelines. In terms of retrieving information and knowledge that can then be transformed into statistically relevant quantified data, there are a few main points to consider; the experimental characterization, the type of data obtained, as well as the analysis of the data.
Why is the development of machine learning-based image analysis workflows important?
A comprehensive analysis of the microstructure is essential in order to gain statistically relevant information. However, this requires the generation of a huge amount of image data.
For example, 3D imaging data sets consist of 100 to 1000 slices, and each of these slices may contain millions of pixels that need to be efficiently and accurately processed.
Manual labeling is very subjective and highly labor intensive, and the conventional algorithm that is typically used can be very narrow in its application to a problem. It also cannot be automatized, and it is unable to treat big data. Supervised AI workflows that incorporate things like convolutional neural networks provide novel possibilities in this context and are, therefore, important for accelerated data treatment.
How can neural networks be used to predict microstructure, and why is this a necessary process?
Neural networks are inspired by a brain architecture using neurons as a fundamental unit for communication and signal transmission.
Convolutional neural networks (CNN) are a class of artificial neural networks that mimic biological architectures. Simply put, it consists of an input and output layer and so-called hidden layers. Each neuron in each layer is connected to all of the neurons in the next layer.
Such networks are highly suitable when it comes to efficiently, objectively, and accurately distinguishing between the different material phases found in the electrode microstructure.
Once the model is sufficiently trained, automation of the segmentation process is feasible.
What structure parameters can this process be used to assess?
In principle, the CNN can be used to distinguish between different phases that are present within the electrode (e.g., anode microstructure). Once an accurate and validated segmentation is performed, the phases can then be quantified with respect to their Vol% and analyzed to gain information about the tortuosity, connectivity, or ability to form networks, etc.
Examples of such phases in the anode are the Si-phase, the graphite-phase, the carbon binder domain-phase, and the pore-phase. Such phases can also be associated with induced damaging sites upon electrochemical cycling, like delamination, cracks, dendrites, etc.
What methods are used to investigate other structural parameters, such as porosity, pore size distribution, and tortuosity?
We use different methods within the lab like FIB-SEM-nano-tomography, X-Ray tomography, but also neutron tomography or synchrotron tomography at large-scale research facilities like ESRF or ILL to investigate the microstructure on different length scales.
To extract structural parameters, characterization of length scales ranging from mm to nm is important. As there is no single method that is able to cover all length scales, we have to develop workflows that comprise different methods to help users come to the best possible resolution, field of view, and contrast.
How is this information fed back to production to influence the manufacturing process?
Analyzed and collected microstructure parameters/features are correlated with electrochemical properties, for instance, their capacity behavior upon cycling. Based on the findings, suggestions are made for improvement, e.g., the use of different material configurations, process parameters, or tools.
It is an iterative workflow that links characterization, research, and the manufacturing process with the industry.
How are workflows tailored to be used in cooperation with Zeiss based on Microscopy methods?
In order to tackle the disadvantages associated with Si, the design of the microstructure architecture is very important. How the material evolves and controls the cycling stability is an important question that needs to be answered for the development of next-generation Si-anodes. Therefore, a deep structural and chemical understanding of next-generation Si-based anode materials is necessary over different length scales down to the lower nm-regime.
Within our collaboration with Zeiss, we are able to push the limits by developing novel characterization workflows for energy-related materials that incorporate advanced image-based (microscopy) methods. Zeiss has the opportunity here to develop improved equipment. By working together to incorporate novel equipment, design advanced workflows and link those together, we are able to improve the capacity of Li-ion batteries.
Acknowledgments
We acknowledge the financial support from the European Union (EU) under the Horizon 2020 research and innovation program (grant agreement No. 875514 “ECO2LIB” and partly by Die Österreichische Forschungsgesellschaft (FFG) under Mobilität der Zukunft, Proj. No. 891479 “OpMoSi”.
Multi-scale quantification and modeling of aged nanostructured silicon-based composite anodes | Communications Chemistry (nature.com)
About Roland Brunner 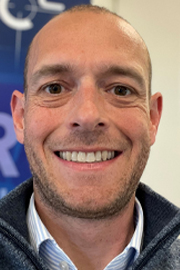
Dr. Roland Brunner, Priv. Doz. is a group leader at the Materials Center Leoben Forschung GmbH (MCL), within the Department of Microelectronics. Since 2021 he is deputy head of the department of Microelectronics. His team focuses on image-based material characterization and analysis in energy storage and microelectronics. Within his group advanced imaging and analysis workflows leveraging artificial intelligence are developed to characterize the structural and chemical properties across the cell- and microstructure-levels from mm to nm in 2D as well as 3D.
He did is PhD in semiconductor/material physics at the Montanuniversitaet Leoben (MUL). Before he joined the MCL in 2012 as key researcher, he worked at the MUL as a University Assistant and at the University of Tokyo, Japan as a research physicist. In 2014 he obtained his facultas docendis and was appointed to priv. Doz. for material science at the MUL. He is also coopted by the University of Graz in the Doctor School of Natural Science.
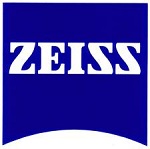
This information has been sourced, reviewed and adapted from materials provided by Carl Zeiss Microscopy GmbH.
For more information on this source, please visit Carl Zeiss Microscopy GmbH.
Disclaimer: The views expressed here are those of the interviewee and do not necessarily represent the views of AZoM.com Limited (T/A) AZoNetwork, the owner and operator of this website. This disclaimer forms part of the Terms and Conditions of use of this website.