Smart agriculture is all about harnessing the power of technologies such as artificial intelligence, robotics, and the Internet of Things, for automation and to improve crop quantities and quality. Even with fluctuations in produce prices, a ~5% improvement in crop yields can ensure agriculture remains profitable.1 How and why can smart agriculture help achieve this?
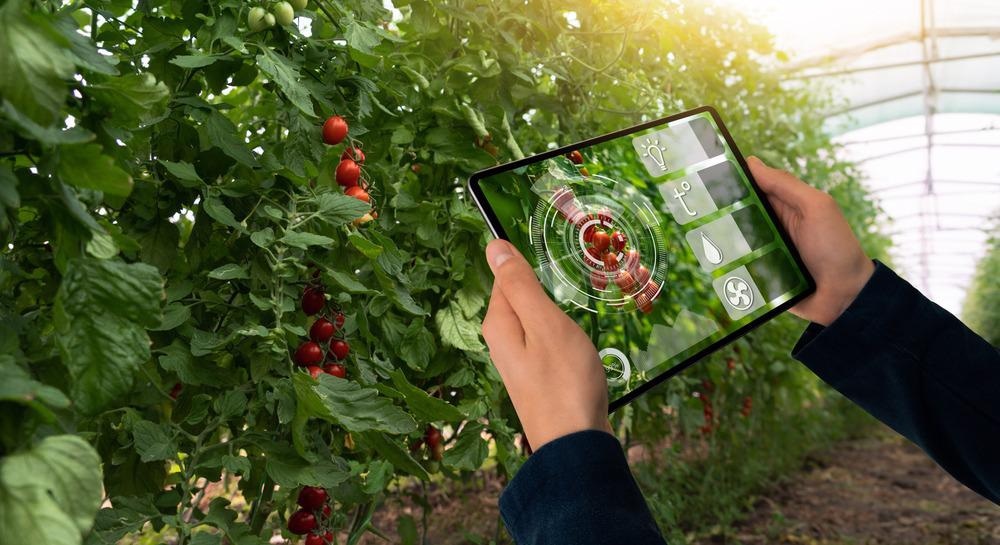
Image Credit: Shutterstock/Scharfsinn
Managing agricultural land can often be very challenging due to its size and scale. Manual inspections, therefore, become very labor and cost-intensive. Crop disease is one task that is typically reliant on visual crop inspection before samples are sent to a laboratory for analysis, introducing further delays before a diagnosis can be confirmed.2
For plants farmed in greenhouses, sensors for humidity, temperature, and light levels are often used to ensure optimal growth conditions for the crops. However, many sites will still rely on manual logging and monitoring of environmental conditions, which prevents quick responses to any changes or technical issues such as the failure of a heating unit.
All of these challenges can be solved through the use of smart agriculture, and for many of them, the use of machine vision. By combining data gathering devices, such as sensors, with feedback and control systems, many tasks in agriculture can be entirely automated.
In the example of a greenhouse, sufficient sensor coverage means condition monitoring, logging and control can easily be automatized and, in conjunction with machine learning algorithms, even be used to increase energy efficiency.3
Machine Vision
Problems that can be addressed through the use of machine vision in smart agriculture include disease inspection, crop distribution measurements, growth monitoring and produce counting, among many more.4
While using artificial intelligence and automation for tasks that require visual identification can be challenging, machine vision has already proved to be a powerful tool in precision agriculture with an excellent return on investment.
With data obtained through machine vision, these datasets can be analyzed to identify areas where pesticides are most needed, thereby minimizing their use.
Some applications, particularly for the identification of crop distribution, use cameras mounted on drones to provide aerial surveillance of the area of interest. Field robots that help with picking and weeding can have cameras mounted on the robot itself.
For many applications, standard broadband visible cameras are suitable but, particularly for disease diagnostics, using multispectral imaging with wavelengths in the near-infrared or even X-ray may be beneficial.5 Different regions of the electromagnetic spectrum are sensitive to different structures, e.g., thermal imaging is well-suited for identifying water stress in plants as well as disease onset.6
Camera Requirements
Taking full advantage of the power of machine vision for agriculture relies on the selection of the correct camera. First, it is important to identify the problem to be solved as this will determine the wavelength region, required resolution, speed, and interface of the camera.
Secondly, the environmental conditions for imaging will also be important. Imaging in the visible range with varying lighting conditions will require a camera with a high dynamic range to capture images without oversaturation or losing details in the readout noise.
For example, for pest and damage identification, the visual signs of a problem may be very small. Affected plant areas may be hidden under other leaves, which could be problematic for top-down visible imaging. For these reasons, Allied Vision, the expert in machine vision camera solutions, offers a range of camera options for agriculture applications.7
For agriculture, sometimes a wide field of view is desired. Achieving high-resolution means using cameras with a large number of pixels, such as the Prosilica GT6400. Although speed is sacrificed when using higher resolutions, the region of interest can be specified to increase the frames per second and minimize the amount of data transfer and volume to be analyzed.
As these cameras need to be used in the field and portable, camera size and housing type can be crucial. Depending on how the cameras will be integrated into devices, closed housings are usually needed for most applications, and Allied Vision offers several options and combinations for their full camera range. Drone-mounted devices need to be lighter, and so smaller cameras like Alvium or Mako may be an appealing choice.
One of the challenges for high-resolution imaging of crops is the transfer of high volumes of data. USB 3.0 is a straightforward interface type and readily accessible, with excellent transfer bandwidth of approximately 350 MB/s to help reduce deadtime between image acquisitions. However, GigE may be preferable in scenarios where very long (up to 100 m) cable lengths are required to ensure no data loss during transfer.
The flexibility and customizability of Allied Vision’s cameras are crucial for challenging machine vision applications like smart agriculture. Often, imaging such complex objects under challenging and varying environmental conditions require the use of multiple cameras and combined analysis of the information.
However, this is key for finding new innovative methods for agriculture to increase crop yields and improve plant health while minimizing the use of expensive and potentially harmful pesticides.
Reference
- Department for Environment Food and Rural Affairs (2019) Total Income from Farming, https://www.gov.uk/government/organisations/department-for-environment-food-rural-affairs, accessed 25th April 2021
- Wale, S. J., Platt, H. W., & Cattlin, N. D. (2008). Diseases, pests and disorders of potatoes: a color handbook. Elsevier.
- Escamilla-García, A., Soto-Zarazúa, G. M., Toledano-Ayala, M., Rivas-Araiza, E., & Gastélum-Barrios, A. (2020). Applications of artificial neural networks in greenhouse technology and overview for smart agriculture development. Applied Sciences (Switzerland), 10(11). https://doi.org/10.3390/app10113835
- Mavridou, E., Vrochidou, E., Papakostas, G. A., Pachidis, T., & Kaburlasos, V. G. (2019). Machine vision systems in precision agriculture for crop farming. Journal of Imaging, 5(12). https://doi.org/10.3390/jimaging5120089
- McCarthy, C. L., Hancock, N. H., & Raine, S. R. (2010). Applied machine vision of plants: A review with implications for field deployment in automated farming operations. Intelligent Service Robotics, 3(4), 209–217. https://doi.org/10.1007/s11370-010-0075-2
- Vadivambal, R., & Jayas, D. S. (2011). Applications of Thermal Imaging in Agriculture and Food Industry-A Review. Food and Bioprocess Technology, 4(2), 186–199. https://doi.org/10.1007/s11947-010-0333-5
- Allied Vision (2021) Allied Vision, https://www.alliedvision.com/, accessed 25th April 2021
- Allied Vision (2021) Analysing H2O via SWIR, https://www.alliedvision.com/en/news/detail/news/the-future-of-agriculture-unfolds-as-researchers-analyze-h2o-absorption-via-swir.html, accessed 25th April 2021