Interview conducted by Olivia FrostFeb 8 2022
In this interview, Dr. David Honigs, Ph.D, the Field Applications Scientist at PerkinElmer, Inc., talks to AZoM about the development of Honigs Regression.
Could you introduce yourself and the work that you do?
I am David Honigs and I work for PerkinElmer instruments. I began the development of Honigs Regression back when I was working for a company called Perten, which PerkinElmer has since purchased. I want to talk about the basic ideas of Honigs Regression and this type of analysis.
I will also give some insight into how Honigs Regression works in contrast to Partial Least Squares, which is a common regression technique frequently used in many fields, including near-infrared spectroscopy. One of the things that makes Honigs Regression so attractive is how quickly it can update with new data.
Why do we make regressions in the first place?
We make regressions because near-infrared spectra are easy to measure and it is the chemistry that is the hard part. So whenever we can measure a proximate chemical constituent by near-infrared, we would like to use that near-infrared spectrum instead of the chemical measurements.
The calibration or the regression which creates the calibration relates the NIR spectra to the chemical constituents. Those calibrations made by the regression process are easy and cheaper. One of the things that I like is that there is never really anything hazardous to them. You can sip on a cup of coffee while you are doing it, and it does not create any lab hazards.
Could you talk about some of the ideas behind regression?
A regression line is sometimes called a best fit or sometimes the least-squares fit. If we plot data points and look at the absorbance at one wavelength versus absorbance at another, you can see the best line that goes through them. That line is often called the regression line.
If we have more than one dimension, then we wind up with a regression in more than one dimension. So instead of having a best line, we are finding the best plane that fits between the two variables. And so with those different variables, essentially the sample concentration is projected to be on that plane.
As we add more dimensions, we would go from that line with one dimension, comparing two things to a plane, comparing three things to a cubic space, comparing more and more, and higher and higher geometries.
What does this mean in terms of variables in regression?
So multiple linear regression is using more than one variable. Multiple linear regression generally uses what is called a linear model. We expect that as everything increases, as that doubles along the line, the concentration doubles and our signal is proportional to that change. A non-linear increase would be some other pattern, such as an exponential. The data fits a known pattern but it is growing at a different rate than a straight line.
The fact is that when we deal with regression and near-infrared spectra, we use this linear assumption. It is embedded in Partial Least Squares, for example, and there is linear technique underneath everything. But the world tends to be non-linear and so we are then left trying to take our linear model, our PLS and try to fit it into a non-linear situation. When that happens, the results of the regression and the calibration line are not going to fit the data as well as we might like.
How does one resolve this issue between the calibration line and data then?
One more concept I want to introduce is the idea of a dumbbell distribution. We call the distributions that because they tend to have a lot of samples at a couple of spots, not so many in between.
These have what you call a within-group relationship. It turns out that a dumbbell distribution is exceptionally hard for PLS to handle well, and it comes up all the time. It especially comes up with factories that are making defined products that are related to each other.
These are the basic problems that we face with our calibrations. Most of the tools that we use in multiple linear regression in PLS are linear and nature is not so linear. A lot of the time we have groups of products with no samples in between and the regression has a hard time trying to decide whether it is going to explain the between-group distance or whether it is going to explain the within-group difference.
How do you resolve the issue of dumbbell distribution?
If you want to have a really good correlation coefficient, when you get some samples that are very low, and some that are very high, the resulting regression line will be very long. Either R squared or R, however, you are looking at it, is going to be a significant number, but that does not mean it is actually explaining anything because most of what is explained is just the difference between the two groups and not what is going on inside of either of those groups.
Once the calibration has been built and the regression work carried out, it is worth noting that the regressions need to be updated year after year when the process changes, when supplies change and when crop years change.
It is because things keep changing that the calibration is not universal. The calibration was made with a certain set of samples and as soon as we step outside of that group of samples and what they represent, things are going to change. These are the problems that I was facing year after year in my job.
How do you get out of the trap of having to do these models over and over and over?
If you hold yourself to a mathematician's level of truth, you can build some amazing things that are based on the truth that will always help you. They may not solve your exact problem, but at least you can be confident in the tools that you build with this type of approach.
One thing I can say is that if the spectrum is the same, the concentration must be the same. Every single equation out there, whether it is PLS or ANN or anything else, has this property. We would say that this problem is one to one. This spectrum has this answer, and a different spectrum may have a different answer. The problem is ideally one-to-one.
The real question is, are the spectra actually the same? And the answer is that they are rarely even close.
We have to figure out how to handle the spectra to get rid of any differences. It is fundamental to Honigs Regression that we deal with spectra where this is true. If the spectrum is the same, the answer is the same. It is called a previously solved problem. If we have that previously solved problem, how do we make that more true?
That is the first job and the way we do that is with pre-treatments. So if instead of the spectrum being the same, we now change this to say, if the pretreated spectrum is the same, then the concentrations must be the same. We are saying that it is okay to filter out things like scatter and particle size that are not important to the problem and try to work on this reduced problem.
How do pretreatments help in relation to the spectrum?
Some pre-treatments do not remove all of the undesirable things and so we are still left with many variations that give the same answer, and some actually change the information that is the real spectrum.
When they do this, they are also going to potentially change our answer. So the pre-treatments that do this, that will take and remove the scatter, will take and remove the baseline shift. Those types of things must be more useful as data treatments than the others. Data treatments that demonstrate this behavior are standard normal variant, de-trending, mean centering, orthogonalization, similar sorts of idempotent functions
By returning to the first principle, there is this idea of a pretreatment that we are going to use that will change the spectrum to the point where it is identical to other spectra of this same material. That has to do with instrument matching, particle size, scattering effects, and optical geometries. That is a really important step to understanding this.
Another thing that happens in regression is that the mean becomes the most certain spot. When thinking of a line that is the best fit, our error is in the regression line. Our uncertainty you see has a waist and that waist is right on the mean. And so that is the most certain spot, when a sample is there, we know that its concentration must be on that line, plus, or minus the least amount.
Instead of thinking of regressions as just a bunch of data points with the line through them, we think about regressions as having uncertainty to them and that the most certain spot is the mean. As a matter of fact, it is said that if you do not know anything other than the mean if you guess the mean you are going to be as close as possible in that circumstance.
How can we make use of this?
One thing that has been tried previously is cascading calibrations. We have one calibration that possesses a certain accuracy over a large range. And then we break that into two calibrations, which will handle the separate ranges. And then we could even break those two into two more.
We wind up with a total of four separate calibrations. Our answer essentially pings off of the first one, into the second one, which picks which of the four we should use, which gives us the result. People have been doing this for at least 30 years in the field of near-infrared, and probably much longer than that in other areas.
This process handles those dumbbell relationships, and the first calibration is going to separate it into which group it belongs to in the dumbbell. The next one is going to be focused on one end or the other, and that will help it better fit the between-sample variability instead of the between-group variability. So this is a useful technique.
Why are people not using this technique more?
People tend not to use this technique as much as it gets harder and harder to make and maintain so many calibrations. If you have a lab error when you are trying to decide which sample you should use to make the calibration, there is a limit as to how many of these divisions you can make. You can definitely divide things into two. Sometimes you can divide them into four. After that, it really depends on the accuracy of your lab technique and the variability of your data.
Instead of using separate calibrations, each depicting the next calibration and so on, I could group the data by the spectrum.
I know that if it has got the same spectra, it has got to give the same answer. And so the leap I am taking here is that if it has almost the same spectra, it should have a similar answer. They should at least be related.
If we just keep cascading spectra, these answers get better, but there are more calibrations to make. I have seen people do this when accuracy is critical, but I have not seen very many people maintain that over time. It just becomes too difficult.
Is there a way to maintain calibrations that do not cause too many problems in the long term?
There are ways that we could break up the different regression lines, we could ask the operator which value it is.
We are asking the operator what the answers should be beforehand and then the remaining calibration just touches it up a little bit. This is how it is actually done, and I have seen this done in many different places. Operators tend to call these channels.
When you see what is called channel creep, you start to see a whole bunch of calibrations measure the same things, varying slightly only on what slight product differences are. They are essentially asking the operator what the answer should be and then giving it back to them. You wind up with many separate products, many separate biases and chasing these biases becomes a full-time job.
Then you get the wrong answer, and there is no warning that it is the wrong answer. The instrument does not have any way of knowing if that additional information is accurate or not. As I said before, you can cascade calibration, you have that one calibration to rule them all, and then you pick the high and low and separate them and separate them again. You have to maintain all of those.
If your lab has a significant amount of error to it, it gets harder and harder to decide which calibration the sample should be used to make. That is the limit on this approach. If the spectra look the same, maybe they are similar. So we are taking our rule of ‘same spectrum same answer’ to include similar spectra similar answers.
Is Honigs Regression better than the other calibration techniques?
We have done enough work to say that the Honigs Regression can compare reasonably well to ANN calibrations in quite a few different situations. It is not always better, but it is not necessarily worse either. The advantage that the Honigs Regression has compared to ANN or even PLS is that it is really quite easy to create, and is especially easier to update. That ease of update happens when you take essentially a sample and add its spectrum to the library.
For every spectrum that you add, you need to add its lab value for the concentrations you are interested in. So as we add new labs and new concentrations to the library, that automatically updates the Honigs Regression. It does not recompute the calibration, it is using the same calibration and adjusts how it calculates the sample means based on the new examples that it has. This makes it very easy to adapt to new situations without throwing out everything that you have done before.
As it can be updated, Honigs Regression is what is called a learning regression, which means we have a library of spectra and labs from previous examples. If we ever see that same exact spectrum again, we know that it is that same laboratory. It is kind of like a ‘reference table’ in a way.
When we collect new spectra and new labs and we keep expanding that library, we have more examples to compare it to. We have more detail as we continue to expand the library. Expanding the library is evolutionary learning called a slow learning process. We do not bring about a revolution and overthrow all that we have done before. We simply keep making what we have a little bit better and a little bit better.
I like the improvement in accuracy that Honigs Regression offers. I like that it is pretty simple to do, but in my opinion, learning is the key. That is because keeping calibrations up to date and changing biases and adding a few more calibrations from a new season, is a lot of the workload that an application specialist has to do.
Some examples of learning that I have seen include a single calibration made for use on whole wheat that can also work for ground wheat, barley, malted barley, flour, and other bakery mixes. So this learning does exactly the opposite of channel creep, instead of breaking out the data into more and more calibrations that we have to maintain separate biases on, if not separate calibrations on, we wind up being able to put more and more diverse things together into one calibration.
Would having just one calibration improve near-infrared measurement techniques?
The closer you come to one master calibration, the closer you come to making near-infrared a primary measurement technique instead of a secondary technique. You are getting to the fundamental cause when you relate the spectrum to the laboratory measurements.
Honigs Regression learns from samples with high M distances. That is, samples that do not fit the model very well or samples with a high global H. Those are samples that are not near very many others. It learns from them as soon as they are added to the library.
Now, when most people are making regression, the temptation is to throw those high M distance or high global H samples out and make the regression without them because they tend to cause PLS to misbehave and pay more attention to the between-group distance than it does to the within-group variability.
The Honigs Regression does not do that. It can have those samples in the library without having them make the calibration change. You do not have to update the calibration. When you get these samples, you just add them to the library. That means that you can have a tested and trusted calibration that you are very confident in and that it will adapt to local conditions and unusual samples just by adding a few more examples of them.
Basically, the Honigs Regression never stops learning. You can keep updating it and redoing the calibration for it.
How do you maintain a fixed calibration when the Honigs Regression is being persistently updated?
When talking about updating, the question that comes up in regard to maintaining calibration is biases. When noticing a change in calibration bias, it is necessary to update the bias and ask what is that bias fixing? It is likely fixing differences between the labs that the dataset was calibrated on and that you are comparing it to now. That bias is fixing differences between instruments or it is fixing differences in the same instrument over time.
An awful lot of the time it happens that you have a new variable in the spectrum, something has changed to the formulation and on average you can say that it causes a bias. You put that bias in, the calibration will keep running. The customer will be happy, but you really have not explained what is happening.
That is because when you treat the spectra, you treat them all as one group. You do not look at the separate different types of groups that are going on that cause a sample to be biased. The key is using data to adjust the bias without messing with the data that you use to adjust these samples.
To me, the idea of fixing a bias means that something is wrong with your calibration or your instrument or your laboratory. It does not mean that you fixed anything. It means that you have covered something up. If you were adding samples to the library and the library adapts, that is actually fixing something, that is getting the cause in the data set.
Could you perhaps give us an example of how this could work in an application setting?
When using a calibration made on one type of material to predict a different one, we do not expect it to work well out of the box. So when we use a ruminant calibration on a ruminant test set, we can see that the support-vector machine and the Honigs Regression give almost the same accuracy.
In this case, the support-vector machine is just a little bit lower than the Honigs Regression.
The PLS is a good technique. It is giving a reasonable answer, but PLS is just not as accurate as HR or support-vector machines on complicated problems or complicated distributions.
When we use these calibrations to predict our monogastric feeds, the support-vector machine is not a good choice, but it does significantly better than either the PLS or the Honigs Regression. As we would expect, if we take a calibration and we use it on something that is not at all like what it was calibrated for, and there is nothing like that in the library for Honigs Regression, it does not do very well.
When adding monogastric samples to our ruminant dataset, we recompute the support-vector machine calibration. We recompute the PLS calibration.
For Honigs Regression, we did not recompute the base ruminate calibration. We simply added the samples to the library. With 10 added samples, our performance on the monogastric has not changed and we have not improved anything except for the Honigs Regression. The Honigs Regression is already starting the slow process of adapting.
It is important to note that as you add more things to PLS, the calibrations can get worse. Because you are adding that non-linearity, you are adding that dumbbell distribution, PLS does not handle that well. As we added more samples, PLS is getting better at the monogastric because those are in there, the calibration is recomputed. They start to have some impact, but it that improvement comes with the price of degrading the accuracy with the ruminant. To make the one better, the other one has to get worse. Those two things are linked because it is a linear system.
By this time, with 100 added samples, you can see the Honigs Regression has adapted really well.
What about lab errors, how do these impact the process?
Lab errors come quite rapidly. When adding more and more samples, you would both the ruminant and the monogastric calibration data in ANN, and we make one calibration. The ANN can do pretty well on either type of material. The errors are not identical because the lab errors are different on those types of materials as well.
However, with Honigs Regression it only made one calibration on the ruminant data a long time ago, and simply just used these added lab samples to get better and better. Learning keeps driving our error down, even when the material that we are trying to predict is not exactly the same as our initial calibration.
With PLS, there is a maximum number of samples, and once you have hit that number, things start to get worse. It is not that there is some magic number that PLS cannot handle. The math just does not behave like that.
What happens is if you add more and more samples of different materials, the PLS keeps compromising. If we put the ruminant and the monogastric together, the PLS does worse than if we had them separate.
Why is Honigs Regression useful for near-infrared spectroscopy.
The world is decidedly not linear. With that being said, grouping is good. When we try to put similar things together, our ability to learn will improve and it is going to simplify the problem. I would like to add that learning always helps solve problems.
It is the non-linear approach that makes Honigs Regression very powerful. It is the learning that frankly saves so much time as users do not have to keep adjusting biases on calibrations or keep updating calibrations. Samples can be added to the library and they are good to go.
Learning is also arguably more valuable than the answer. Learning is a compilation of a lot of different things from the data. We are never going to be completely done with a material, that is just not the way things work. We need to have the ability to learn from new data, from new samples, and with that comes the wisdom to provide the correct answer without having to throw everything out and start over again.
About Dr. David Honigs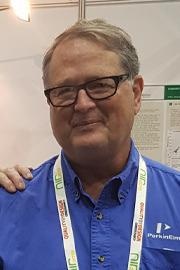
Dr. Honigs did his graduate work under joint supervision of Professor Gary Hieftje and Dr. Tomas Hirschfeld at Indiana University in Bloomington. He served as an Assistant Professor of Analytical Chemistry at the University of Washington for a few years. Following that he worked at NIRSystems (now part of FOSS) on NIR instruments. He started a company, Katrina Inc. which made process NIR instruments.
For the last almost 20 years he has worked at Perten (now Perkin Elmer) on Near Infrared Instrumentation and applications in the food industry. He has 35 research papers as listed on ResearchGate.com He has 10 issued US patents.
About PerkinElmer Food Safety and Quality
PerkinElmer Food Safety and Quality is committed to providing the innovative analytical tools needed to ensure the global supply of high-quality, safe and unadulterated foods.
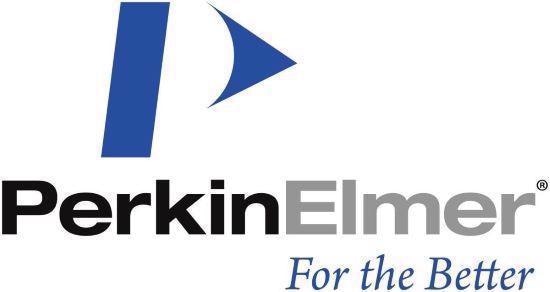
This information has been sourced, reviewed and adapted from materials provided by PerkinElmer Food Safety and Quality.
For more information on this source, please visit PerkinElmer Food Safety and Quality.
Disclaimer: The views expressed here are those of the interviewee and do not necessarily represent the views of AZoM.com Limited (T/A) AZoNetwork, the owner and operator of this website. This disclaimer forms part of the Terms and Conditions of use of this website.