This article focuses on bimodal particle size distribution (PSD) analysis and its significance, applications, and recent studies.
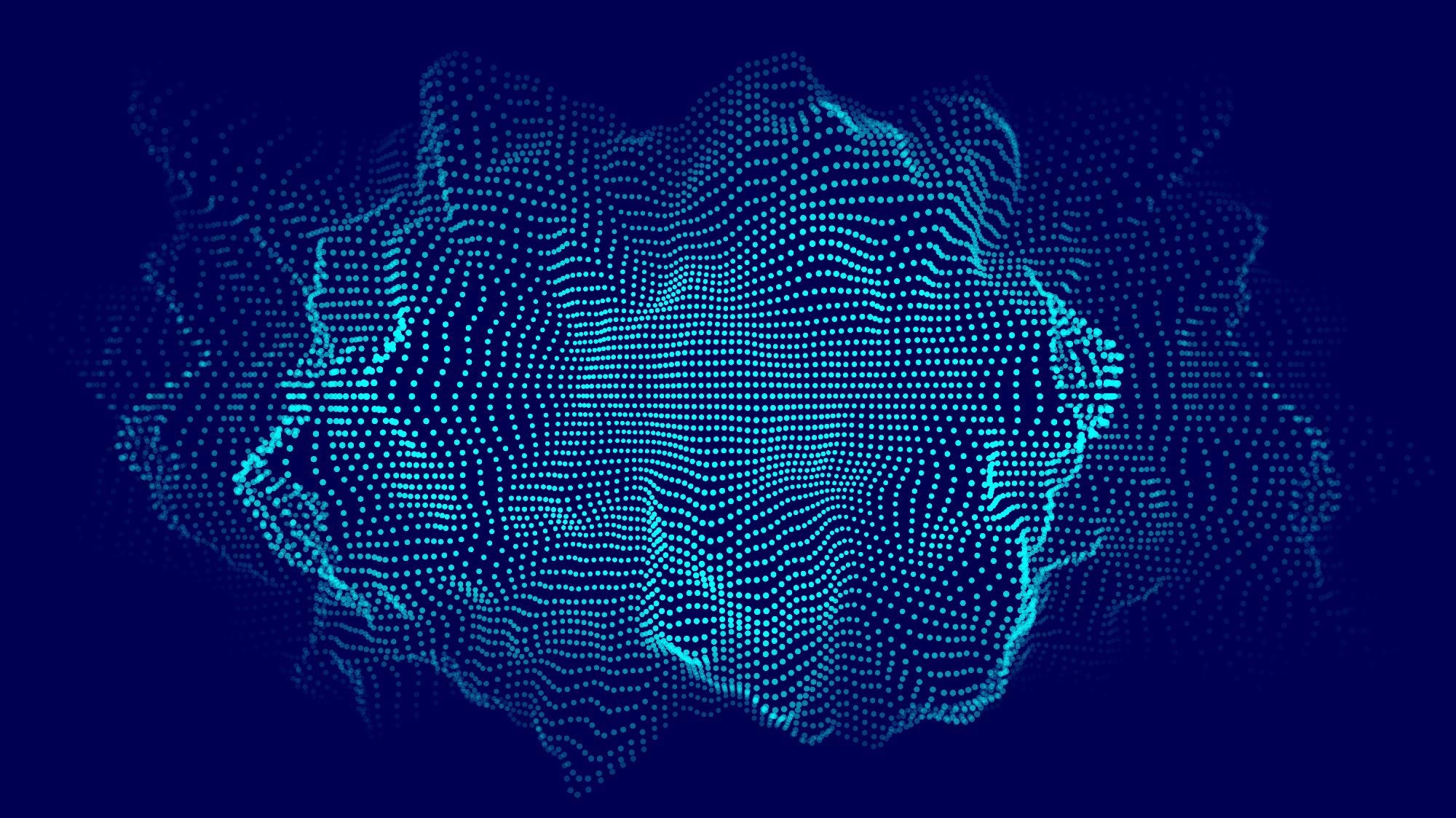
Image Credit: estar 2020/Shutterstock.com
What is Bimodal Distribution?
A bimodal distribution often results from a process that involves the breakup of several sources of particles, different growth mechanisms, and large particles in a system. For instance, bimodal volume distribution frequently occurs in combustion and atmospheric aerosols, where the larger mode is the result of redispersion or breakup, while the smaller mode represents molecular condensation.
Bimodal PSD Analysis
To use a PSD analysis in a bimodal distribution, obtaining a weighted value from the distribution is necessary. However, the relationship between various moments must be considered before obtaining the value, as several analytical techniques yield multiple moments of the distribution. For instance, the comparison of average particle sizes obtained from gas absorption and dynamic light scattering (DLS) measurements, respectively, requires such consideration.
The zero’th moment and the first moment are the total concentration or number of particles and the number of average particle diameter (dp), respectively. The second moment is proportional to the overall surface area of the system and sample turbidity for spherical particles and large particles relative to light wavelength, respectively. The third moment corresponds to the overall volume of the system, while the fourth moment is used to model the sedimentation of particles that follow Stokes Law.
The fifth and sixth moments are proportional to the mass flux of particles that are sedimented from a suspension and light scattered from very small particles relative to the light wavelength, respectively. Additionally, fractional moments are commonly obtained in transport-based measurements such as aerosol convective diffusion and intrinsic viscosity.
However, a function with a minimum number of free parameters must be used to describe the PSD, as obtaining the particle size data from dp = 0 to dp = ∞ is often not possible. The function can be used to calculate all moments. For instance, a function with modulus or peak value, standard deviation, and mean of the distribution as parameters requires three moments for describing the distribution.
The log-normal distribution based on the Gaussian distribution is the most commonly used PSD function. A log-normal distribution is typically used in systems where particles have broken up or formed according to a certain power of particle sizes, such as surface area or volume. Gaussian distribution with three parameters can be used to describe monodisperse particles.
PSDs characterized by a large long size tail can be described by the power-law relationship between the particle number and size using log-Gaussian and log-normal distribution. Power law distribution is used over a limited range of particle sizes, typically in cleanroom applications.
Other distribution functions, such as the Schultz distributions and Lorentzian distribution, contain over three log-normal or Gaussian distributions. These functions can describe several skewed distribution curves.
Significance of Bimodal PSD Analysis
Bimodal PSDs in high ice water content regions were derived to analyze their implications for microphysical models, while bimodal PSDs of thermite composites were produced to evaluate their performance. Additionally, the effect of bimodal distribution on the rheology and maximum packing of crystal-bearing magma was successfully evaluated.
In a study published in the Journal of Atmospheric Sciences, researchers determined the occurrence of bimodal PSDs in midlatitude cirrus clouds by analyzing the ground-based remote sensing data.
Reliable measurements of cirrus PSDs and their bulk properties such as total particle concentration, mean particle size, and condensed water content are necessary to understand the processes controlling the microphysical and macrophysical cloud properties to thoroughly comprehend the feedbacks of cirrus clouds on the climate system.
Bimodality is often considered a defining feature due to the presence of larger particle modes with sizes more than several hundred microns and smaller particle modes with sub-100-µm sizes.
Initially, a wide discrepancy in the total particle concentration values was observed during the literal interpretation of small dimension modes of in situ cirrus datasets and conventional analysis of cloud process model and in situ data, respectively.
Thus, the general nature and inconsistency of cirrus PSD were investigated using a ground-based remote sensing dataset. A bimodal PSD and its uncertainty were retrieved by developing an algorithm using Raman lidar-derived extinction and millimeter-wavelength radar Doppler moments.
The findings of the review indicated that strongly bimodal particle spectra with several hundred-micron large precipitation modes of particles coexisting with a distinct cloud mode of fewer than 50-µm particles dominated the cirrus PSDs, and the bimodality was a function of location and temperature. The presence of a total particle concentration greater than one inverse cubic centimeter was rare, and the concentration as per the remote sensing data was on the order of 100 cm-3.
To summarize, the bimodal PSD analysis has emerged as an effective analytical technique to understand the PSD of systems with a bimodal distribution.
More from AZoM: Effects of Particle Size on Wood-Plastic Composites
References and Further Reading
Zhao, Y., Mace, G. G., Comstock, J. M. The Occurrence of Particle Size Distribution Bimodality in Midlatitude Cirrus as Inferred from Ground-Based Remote Sensing Data. Journal of the Atmospheric Sciences. 68(6), 1162-1177. 2011. https://journals.ametsoc.org/
Sample records for bimodal particle size [Online] Available at https://www.science.gov/topicpages/b/bimodal+particle+size
Particle Size Distribution Functions [Online] Available at https://www.eng.uc.edu/~beaucag/Classes/Nanopowders/ParticleSizeDistribuhtml/ParticleSizeDistribu.htm
Disclaimer: The views expressed here are those of the author expressed in their private capacity and do not necessarily represent the views of AZoM.com Limited T/A AZoNetwork the owner and operator of this website. This disclaimer forms part of the Terms and conditions of use of this website.