A paper recently published in Scientific Reports demonstrated the effectiveness of a novel strategy based on photoluminescence (PL) spectroscopy and machine learning (ML)-based theoretical analysis for plastic litter identification in the environment.
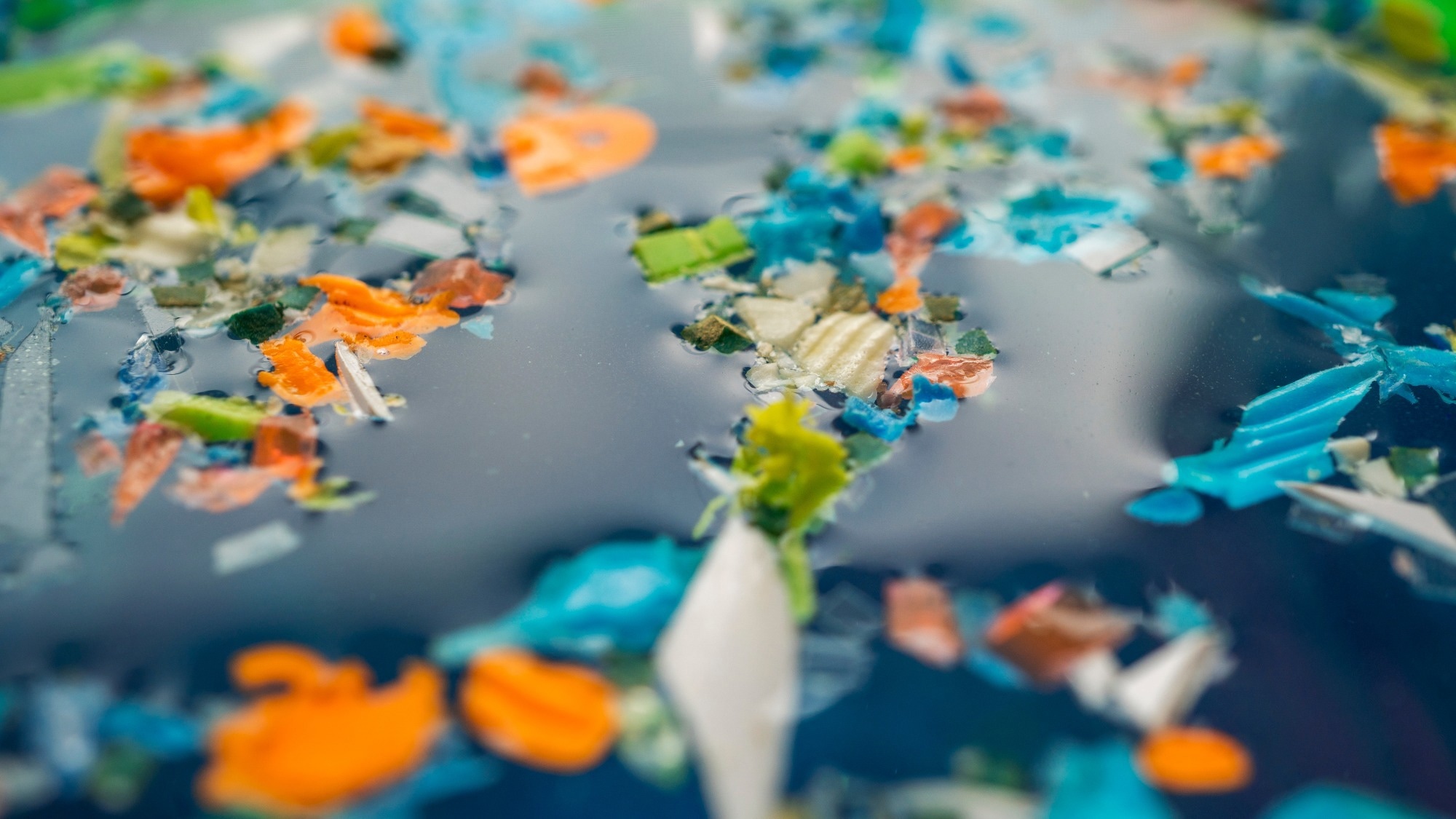
Study: Identifying plastics with photoluminescence spectroscopy and machine learning. Image Credit: SIVStockStudio/Shutterstock
Background
Plastic pollution is one of the key environmental issues affecting the world due to the large-scale accumulation of plastic debris in the environment, specifically the aquatic environment, which can be attributed to the extremely low plastic recycling rates.
Plastic debris can persist for several decades as most plastic types do not degrade under the natural degradation process. However, plastics fragment into microplastics or micrometer-sized particles under severe environmental conditions that harm the environment.
Recently, the presence of microplastics has been detected in food and animals, and even in human bodies, such as the placenta and blood, which necessitated the development of new strategies to track plastic litter in the environment and implement effective plastic waste management plans to prevent plastic litter accumulation.
The new strategies must be based on a quantitative understanding of the characterization and distribution of plastic litter. Plastic samples obtained from various sites can be analyzed to gain crucial insights into the material type and average size of microplastics and their global distribution.
However, detecting plastics in the environment is extremely challenging as different additives are used frequently during production to change their physical properties. Among the techniques that can be utilized to detect plastic litter, non-destructive spectroscopic techniques are considered more suitable as they allow cross-validation with different analytical tools.
Although solutions based on Fourier transform infrared (FTIR) spectroscopy or Raman spectroscopy are typically used to investigate plastic samples, both techniques have several physical limitations. PL spectroscopy has recently gained considerable attention for the identification of plastic litter owing to its simplicity and lower cost compared to FTIR or Raman spectroscopy.
Thus, the technique can be used to extend the monitoring of plastic litter systematically and establish spectral dataset databases by capturing the plastic sample diversity. The sample property prediction from the PL spectrum is based on spectral feature modeling.
Although PL spectroscopy can distinguish common plastic types from the non-plastic samples in the marine environment by comparing their spectral intensity ratios, it cannot accurately predict the individual plastic types. Mathematical analyses, such as supervised ML, are considered effective in scanning high-dimensional spectral data to identify suitable common spectral features for proper sample identification.
However, ML models often demonstrate low prediction accuracy for the newly measured plastic samples. Thus, successful ML models have been primarily used with FTIR spectroscopy as they require a substantial amount of data for learning.
Although the combination of PL spectroscopy with ML models has a higher potential to produce more high-throughput data easily, the feasibility of such combinations has not been explored until now.
The Study
In this study, researchers proposed a strategy based on PL spectroscopy and ML-based theoretical analysis to identify plastic litter. The study's objective was to realize a systematic collection of data characterizing the composition and amount of plastics based on an accessible and simple experimental approach that can effectively capture the diversity of plastics and an analysis pipeline that can extract all relevant parameters from a substantial amount of experimental data.
The proposed strategy leveraged ML model capabilities to identify spectral features that can accurately predict the identifying sample characteristics for newly collected plastic litter. Unsupervised dimensionality reduction (DR) was used to implement a classification method that can deal with the new data robustly and flexibly.
Reducing the input data dimensionality was crucial to improve the ML model's generalizability. The DR process retained all essential information in the raw data. Signal dissection by correlation maximization (SDCM) and principal component analysis (PCA) were used for DR in this study.
Additionally, a set of commonly used supervised classification methods/classifiers, including random forest, logistic regression, nu-support vector machine, support vector machine, and Gaussian naïve Bayes, was used to generate ML models. The ML model prediction performance was analyzed by measuring the model recall, precision, and accuracy.
All generated ML models used the intensity data from PL spectroscopy as input and yielded a predicted material type. The data comprised 1294 measurements from three sample categories, including non-plastic from the marine environment, plastics from different manufacturers and retail products, and 23 material types, which were then combined into nine classification categories.
The material types included polyamide (PA), polycarbonate (PC), polyethylene (PE), low-density polyethylene (LDPE), high-density polyethylene (HDPE), polyethylene terephthalate (PET), polymethylmethacrylate (PMMA), polypropylene (PP), polystyrene (PS), polyvinyl chloride (PVC), sand, wood, Posidonia oceanica (plant), Sepia ofcinalis (bone), Echinocardium cordatum (shell), Hexaplex eggs (shell), Monodonta turbinata (shell), Neverita josephina (shell), and Lithophyllum racemus (algae).
Researchers observed the variations in the PL spectrum between samples and overall ML model performance and detected identifying PL spectra characteristics.
Significance of the Study
Using an ML-based theoretical approach, the proposed strategy of analyzing PL spectroscopy effectively identified plastic litter. Most of the generated supervised ML models successfully identified non-plastic and plastic materials by predicting the plastic litter characteristics based on the PL spectra with more than 95% accuracy.
ML models combined with SDCM demonstrated the best prediction performance. SDCM algorithm successfully identified the potential links between the sample spectrum characteristics and sample properties/spectral features specific to a single plastic sample.
This method could help detect local plastic litter sources and implement more efficient mitigation strategies. The best-performing combination was based on an unsupervised learning technique as it demonstrated a similar prediction performance for both currently available spectroscopic and new data, which significantly differed from the available data.
To summarize, the findings of this study demonstrated the feasibility of this proposed strategy for plastic litter identification on large scales. However, the reliability of the strategy must be evaluated by establishing and maintaining a spectral library with additional experimental heterogeneities and complete sample records.
Disclaimer: The views expressed here are those of the author expressed in their private capacity and do not necessarily represent the views of AZoM.com Limited T/A AZoNetwork the owner and operator of this website. This disclaimer forms part of the Terms and conditions of use of this website.
Source:
Lotter, B., Konde, S., Nguyen, J. et al. (2022). Identifying plastics with photoluminescence spectroscopy and machine learning. Scientific Reports. https://www.nature.com/articles/s41598-022-23414-3