A recent study published in Chemical Engineering Science has marked a significant advancement in membrane emulsification technology, crucial for sectors like pharmaceuticals, food processing, and cosmetics. This research has successfully developed a predictive model for membrane permeance in a cross-flow multi-channel membrane system operating without emulsifiers. The model's precision and practical applicability signify a notable contribution to the field of membrane technology.
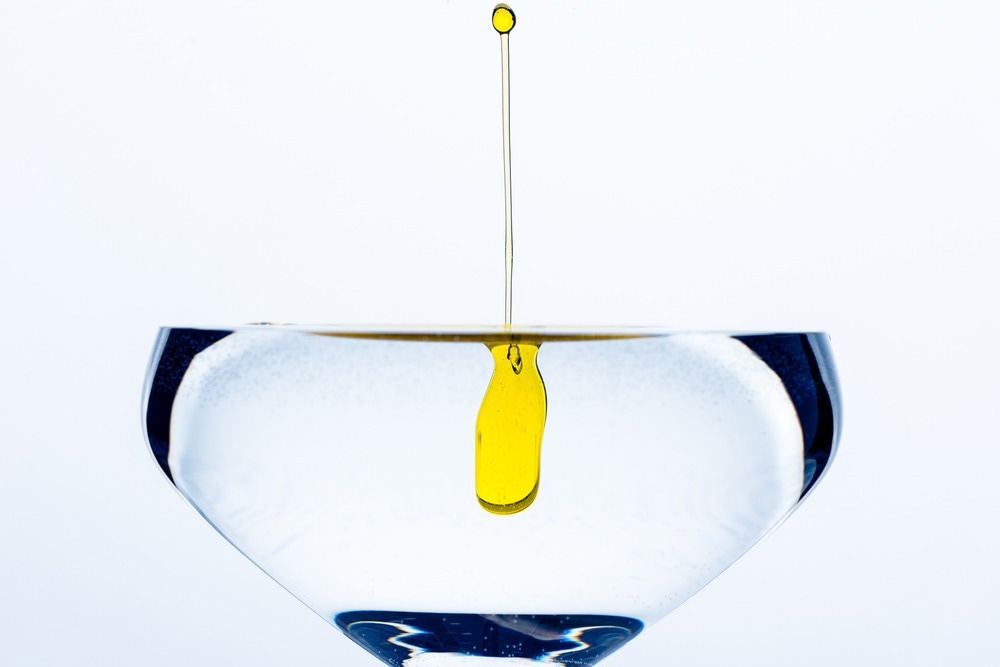
Image Credit: Robert Ruidl/Shutterstock.com
Membrane Emulsification: Technical Overview
Membrane emulsification is a process where two immiscible fluids are mixed to form a stable emulsion, typically oil-in-water or water-in-oil. This technique's efficiency hinges on membrane permeance, a parameter influenced by factors like pore size, transmembrane pressure, and oil-water interfacial tension. Until now, accurately modeling these interactions posed a significant challenge due to the complexities inherent in the process dynamics.
Study Methodology and Empirical Model Development
Utilizing commercial 37-channel ceramic membranes with pore sizes ranging from 50 to 500 nm, the study conducted a series of controlled experiments. These membranes, composed of alumina particles sintered at high temperatures, exhibited a porosity of 30-35% and tortuosity between 1.5 and 2.5. The research focused on understanding how variations in pore size, transmembrane pressure, and interfacial tension impact membrane permeance.
The culmination of this experimental investigation was the development of an empirical model capable of predicting membrane permeance with a relative error margin of ±10%. This model provides a significant tool for determining optimal membrane pore sizes under varying operational conditions, thereby enhancing the efficiency and effectiveness of membrane emulsification processes.
Industrial Implications and Future Applications
The study's findings have direct implications for industries relying on precise emulsification processes. By enabling better prediction and control of membrane permeance, the model allows for more efficient system design and operation, potentially leading to cost savings and improved product quality. The model's adaptability across different membrane pore sizes and operating conditions also broadens its applicability across various industrial scenarios.
The implications of this study for the food and beverage industry are particularly noteworthy. Membrane emulsification plays a pivotal role in the production of various food products, where the stability and consistency of emulsions are critical for quality and consumer appeal. The new model for predicting membrane permeance has the potential to revolutionize this aspect of food processing.
Furthermore, this research opens avenues for future exploration in membrane-based emulsification. The model could be refined to accommodate more complex fluid dynamics or extended to other types of membranes and emulsion systems, potentially leading to further advancements in the field.
Conclusion: Advancing Membrane Technology
In summary, this study represents a significant leap in membrane emulsification technology, addressing a longstanding challenge in accurately predicting membrane permeance. The developed model stands as a testament to the ongoing advancements in this field and holds promise for enhancing industrial processes across multiple sectors. As membrane technology continues to evolve, such research underscores the importance of empirical modeling in driving innovation and efficiency in industrial applications.
Source
Jingcheng Li et al. (2023) Experimental and model analysis of membrane permeance in cross-flow multi-channel membrane emulsification, Chemical Engineering Science. Available at: https://www.sciencedirect.com/science/article/abs/pii/S0009250923010990 (Accessed: 20 November 2023).