For metallic alloys in aerospace, machine learning can make the development of additive manufacturing (AM) processes both faster and cheaper.
In collaboration with the University of Sheffield Advanced Manufacturing Research Centre (AMRC) and Boeing, Intellegens used the Alchemite™ deep learning software to develop a model that could identify optimal AM parameter sets for laser powder bed fusion (LPBF) and test them for nickel-based alloys across two experimental cycles. The results from the second cycle showed great agreement with the scientists’ estimations.
Following this, the model was used in combination with the 400W M2 machine from GE Additive to develop process parameters for the new additive-specific Aheadd® CP1 powder from Constellium.
In just two builds, the project team was able to move from the new powder to the final parameters, applying powerful machine learning methods with no requirement for expert statistical knowledge. This demonstrates how Alchemite™ machine learning can speed up AM process parameter optimization.
Challenge
A rapidly-evolving manufacturing technology, Additive Manufacturing (AM) delivers stronger, lighter components with the potential for on-demand manufacturing and great flexibility in part geometry.
Across many sectors, these benefits are widely recognized – most notably in aerospace, where they can increase fuel efficiency by supporting the lightweighting of aircraft.
However, implementing AM has significant practical challenges. New component specifications often require a new AM machine or metallic powder material. Since final properties are notoriously sensitive to minor variations in material, machine setup, or environmental conditions, finding the optimal machine processing parameters is tricky.
Typically, optimizing these parameters happens through the use of time-consuming, costly experimentation. Using experimental design methods in combination with the knowledge of AM project teams, parameter space is narrowed down. But even using sophisticated statistical analysis, traditional experimental design rarely reduces the number of tests sufficiently, leaving a heavy experimental burden. And, often, the team loses knowledge between projects as changes are made in powders, machines, and team members.
There are two key ways in which machine learning (ML) can assist in this process. ML methods can take learnings from historical data, capturing knowledge in reusable models so that experience from one project can be applied elsewhere. Such models can be used predictively and to reduce the need for experiments by identifying and using subtle relationships in complex, high-dimensional data.
There are constraints on ML methods, however, most notably that they learn from ‘training data.’ In convengtional ML, this data must be complete for all parameters of interest. The difficulty lies in the fact that there are often gaps within real-world experimental data: the data is often assembled from multiple tests, targeting different properties, and can be sparse and noisy where project teams are unsure which parameters to measure.
Can ML be Applied to Accelerate AM Parameter Optimization?
Solution
This challenge was addressed by Project MEDAL. Supported by the UK National Aerospace Technology Exploitation Program (NATEP), this collaboration involved Intellegens, Boeing, GE Additive, the University of Sheffield Advanced Manufacturing Research Centre (AMRC), and Constellium.
At the heart of the project was Alchemite™, a deep learning technology1 which began life at the University of Cambridge and was further developed at Intellegens.
Difficult material and process design problems are tackled by Alchemite™. The core property of Alchemite™ is its ability to handle noisy, sparse, experimental data. It is able to ingest historical project data and build an ML model from it. It can then employ that model to predict or optimize outputs of new systems.
An ML tool for AM based on Alchemite™ was created in Phase 12 by Intellegens, the AMRC, and Boeing. Laser powder bed fusion (LPBF) was the key focus, and the project constituted a major study into process parameter development.
The model, based on historical data, could be used in real-time to guide the choice of process parameters for the next experiment every time a new input to the system (e.g., a new material, machine, part, or even operator) was introduced. Following this method, parameter space was explored with maximum efficiency.
It is shown in Figure 1 how Alchemite™ (represented by the blue line) can optimize a given target based on far fewer experiments than conventional experimental design methods (in many cases, 10x fewer).
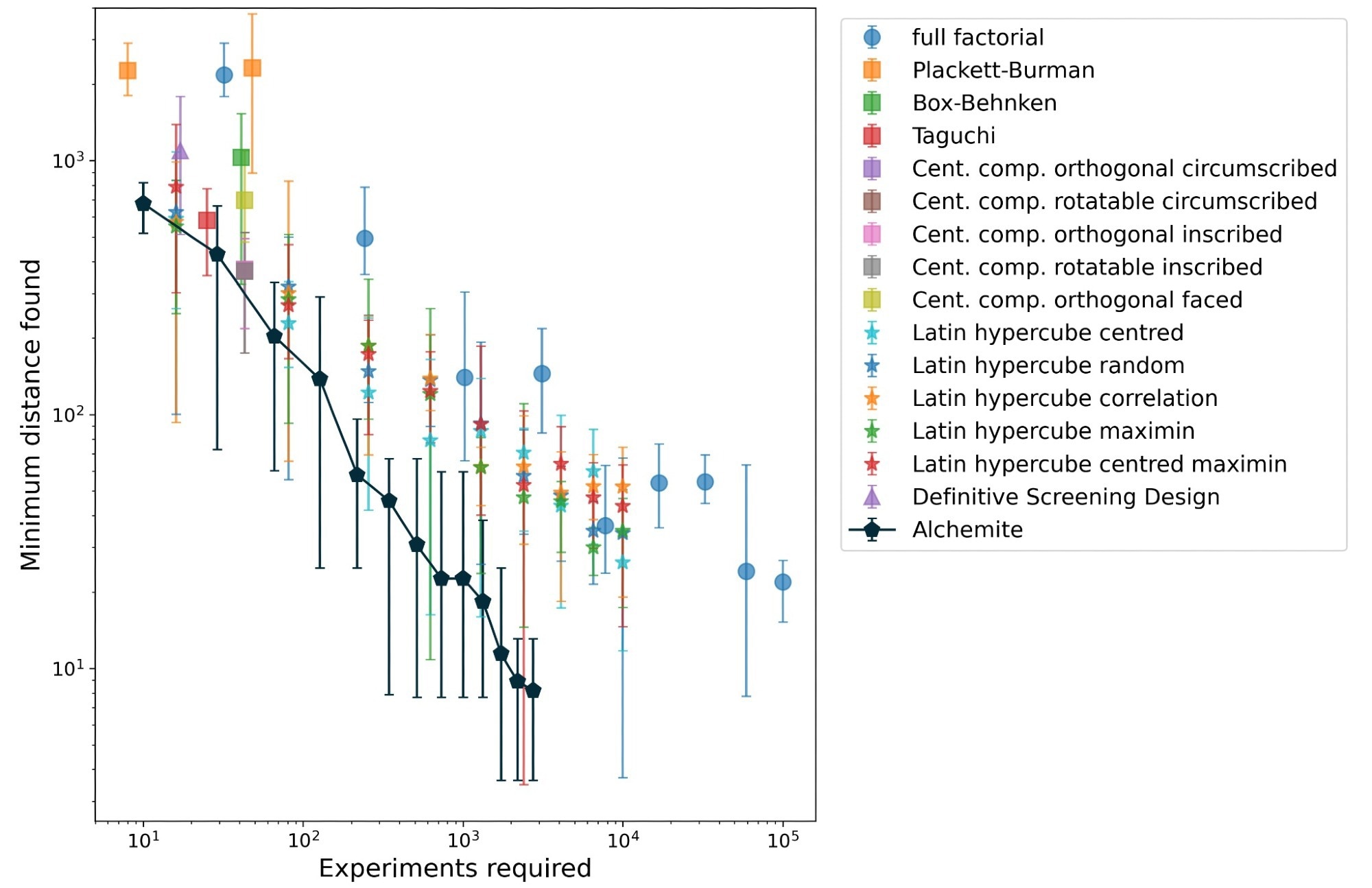
Figure 1. Comparing Alchemite™ with standard experimental design methods. Image Credit: Intellegens Limited
Outcomes
Phase 1 demonstrated that it was possible to learn from historic builds and apply the resulting model to new builds. To predict the results of a second cycle, the outcomes from a first experimental cycle were used, and close agreement was found between the predicted and experimental results.
Working with the Constellium powder and GE Additive machine, in Phase 2:
- With no expert knowledge of the powder, the AMRC engineers could apply the Alchemite™ machine learning tool to guide their work.
- It was found that the machine learning approach was applicable to multiple different machines, powders, and AM processing types.
- The Alchemite™ machine learning tool was able to accurately predict the UTS performance of parts in the second build after just one build (Figure 3).
- Stable, predictable performance was provided by the Aheadd® CP1 powder except for where insufficient laser power led to a lack of fusion.
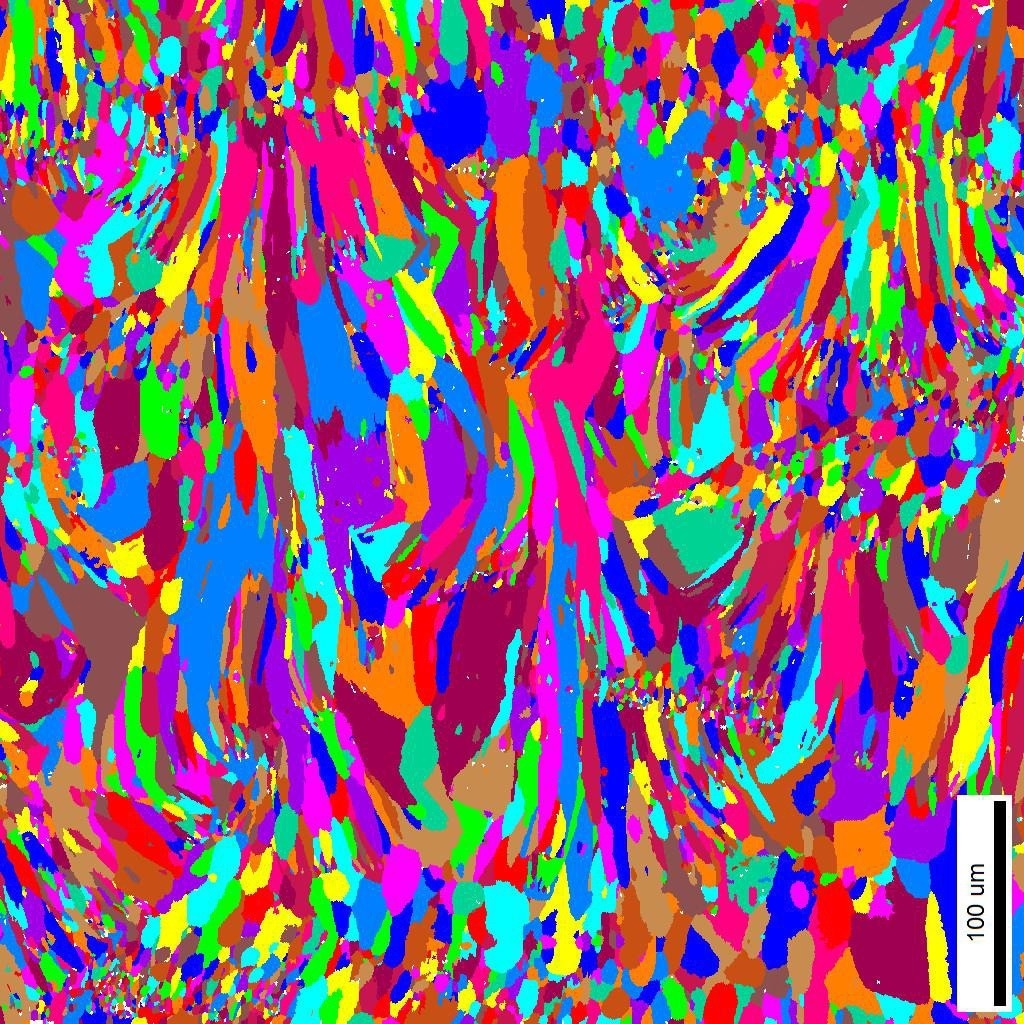
Figure 2. Grain structure of a sample printed using Constellium Aheadd® CP1 >. Image Credit: Intellegens Limited
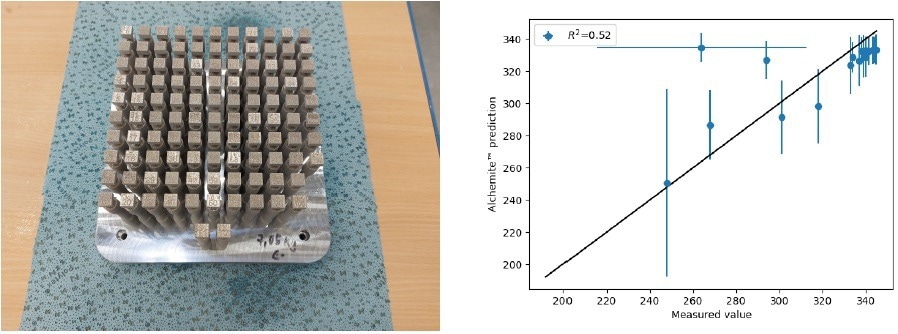
Figure 3. Test samples built during Phase 2 of the project (left) and a comparison of Alchemite™ predictions for the second build UTS with experimentally measured values, showing high agreement (right). Image Credit: Intellegens Limited
Conclusions
The effectiveness of Alchemite™ machine learning in accelerating process parameter optimization for a new AM process was demonstrated by the project.
By significantly reducing the amount of experiment involved, this capability allows AM process teams to decrease the time it takes to bring new processes to market.
The project was led by Ian Brooks, who was at the time a Technical Fellow at AMRC North West. Ian commented:
“Alchemite™ converged on the optimum solution with far fewer experiments. The opportunity for this project is to provide end-users with a validated, economically viable method of developing their own powder and parameter combinations.”
A suite of graphical analysis tools in the Alchemite™ Analytics platform can be used to analyze the predictions made by the ML model - for instance, to find out which input parameters drive key outputs.
As they pursue reliable, repeatable AM processes, AM teams can obtain the most from material and machine innovations, like understanding process/property relationships. Lukas Jiranek of Boeing Research & Technology, quoted in Metal Additive Manufacturing3, explained the company’s involvement:
“There are currently over 70,000 AM components flying on Boeing platforms. With these multiple efforts and the data-rich AM process chain, machine learning has the potential to be a key technology in accelerating the further development and adoption of AM. Project MEDAL is a valuable step in creating and proving-out standardized approaches to machine learning for data-driven AM process parameter development.”
There is huge potential for alloys designed specifically for laser powder bed fusion. This project has confirmed the robust performance of Aheadd® CP1 on the GE Additive platform, and the approach demonstrates we can reach predictable mechanical performance very quickly. Machine learning will allow us to reach optimized AM solutions faster in multiple application areas for Aheadd® CP1, including heat exchange, satellite components, high-end automotive parts and semiconductor industry equipment.
Ravi Shahani, Additive Manufacturing Strategic Partnership Manager, Constellium.
About the project participants
Deep learning software by the name of Alchemite™ is provided by Intellegens. Intellegens’ focus is on simplifying the application of machine learning to accelerate innovation in materials, chemicals, manufacturing, and beyond.
A network of world-leading research and innovation centers, The University of Sheffield Advanced Manufacturing Research Centre (AMRC) works with companies involved in manufacturing of all sizes from around the globe.
Part of GE, GE Additive is a world leader in metal additive design and manufacturing, a pioneering process that can truly transform businesses. A global leader in the development and manufacturing of high value-added aluminium products and solutions, Constellium’s advanced alloys and engineered solutions support a range of applications, such as beverage cans, cars, airplanes and more.
The world's largest aerospace company, Boeing is a leading manufacturer of commercial jetliners, defense, space and security systems, and service providers of aftermarket support.
References
- “Alchemite™ deep learning - solving complex problems with real-world data”, Intellegens white paper (2021)
- Press Release: “Machine learning making light work of additive manufacturing aerospace alloys”, The University of Sheffield Additive Manufacturing Centre, Jan 2021.
- Warde S., “The inestimable value of AI: How Machine Learning can help AM project teams achieve their goals and beyond”, Metal Additive Manufacturing Vol 7 No. 4 (2021)

This information has been sourced, reviewed and adapted from materials provided by Intellegens Limited.
For more information on this source, please visit Intellegens Limited.