Engineers and scientists are interested in innovation and discovery. They rarely want to be lawyers. But, with so much focus on environmental objectives, they are increasingly having to understand and respond to regulatory constraints.
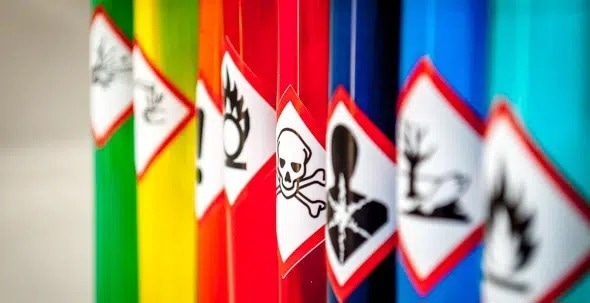
Image Credit: Intellegens Limited
Over the past three decades, regulations, legislation, and directives that impact the use of chemicals, materials, and related processes have multiplied rapidly. California’s Proposition 65 set precedents in 1986 by requiring companies to declare any known risks of exposure to harmful chemicals.
One of the most widely-known examples is the European Union’s REACH regulation, which was codified in 2006 and which restricts the use of chemicals that could harm either human health or the environment.
A different but similar issue was tackled by the Dodd-Frank Act of 2010, which placed reporting requirements on the use of ‘conflict minerals.' Globally, there are hundreds of laws on similar topics - impacting not only companies based in the relevant regions but the sale of products to or within those markets.
Despite the clear benefits of maintaining human safety and tackling climate catastrophe, the risk from a product development perspective is that such a web of regulation may disrupt a product or process that has been working well.
The exact reason for disruption may differ and; therefore, the required response will differ too. For instance, product development may be interrupted if a particular chemical (perhaps used as a fire retardant, an additive, or coating) is added to a list of restricted substances or materials of concern and has to be removed from the product chain.
The required change may, however, be more subtle – for instance, if legislation is introduced which requires the used substance to be present below a certain threshold amount, or if guidelines that require changes to a secondary process ultimately reduce its efficiency.
Such guidelines or legislation can alter market dynamics, meaning that a chemical which is still safe to use for an application becomes scarce or much more expensive. Changing market perceptions may mean that certain materials, even if not directly harmful themselves, can become unpopular.
Resilience and speed are the key factors here. Manufacturers need to be able to respond rapidly to changes required by law. However, most chemical, materials, and formulations development processes are not well-configured to work in this way. Instead, they rely on systematic studies of the candidate solutions, based on often-slow and costly experimental programs.
For a business that has perhaps been working in the same way for years and suddenly needs to change production, the good news is that they are likely to have a great deal of historical experimental data. If that data can be mined, vital clues about how to respond to new regulatory challenges could be revealed.
This is where machine learning (ML) comes in. ML can learn from the legacy data to build models that can then be used to predict how changing ingredients and process parameters will impact the properties of their products or processes.
Once built, organizations can use these models to explore a range of new alternatives, such as how changing material might affect the economics of their process, potential alternatives to an ingredient, or how to modify a formulation to achieve target properties while using no more than a specified amount of an additive.
Legacy data used in this way causes one potential issue: it is likely to be collated from different projects run at different times and with different objectives. A collation of such data is unlikely to form a coherent set; it will have ‘noise’ and gaps. This means that conventional machine learning runs into difficulties when it attempts to generate good models from such data.
To work with this real-world experimental and process data, Intellegens has developed the Alchemite™ machine learning technology. This technology is focused on deriving reliable and accurate results from sparse, noisy data, meaning that these sorts of formulation and reformulation problems can be tackled.
Domino Printing Sciences, for example, used Alchemite™ to cut the amount of experimentation required when reformulating inks.
Machine learning could be a useful tool in an R&D toolbox if the given formulation, material, or chemical development process needs to be set up for a resilient and fast response to regulatory challenges.

This information has been sourced, reviewed and adapted from materials provided by Intellegens Limited.
For more information on this source, please visit Intellegens Limited.