By Surbhi JainReviewed by Susha Cheriyedath, M.Sc.May 16 2022
In an article recently published in the journal Additive Manufacturing, researchers discussed the computation designing of a crack-free aluminum alloy for additive manufacturing.
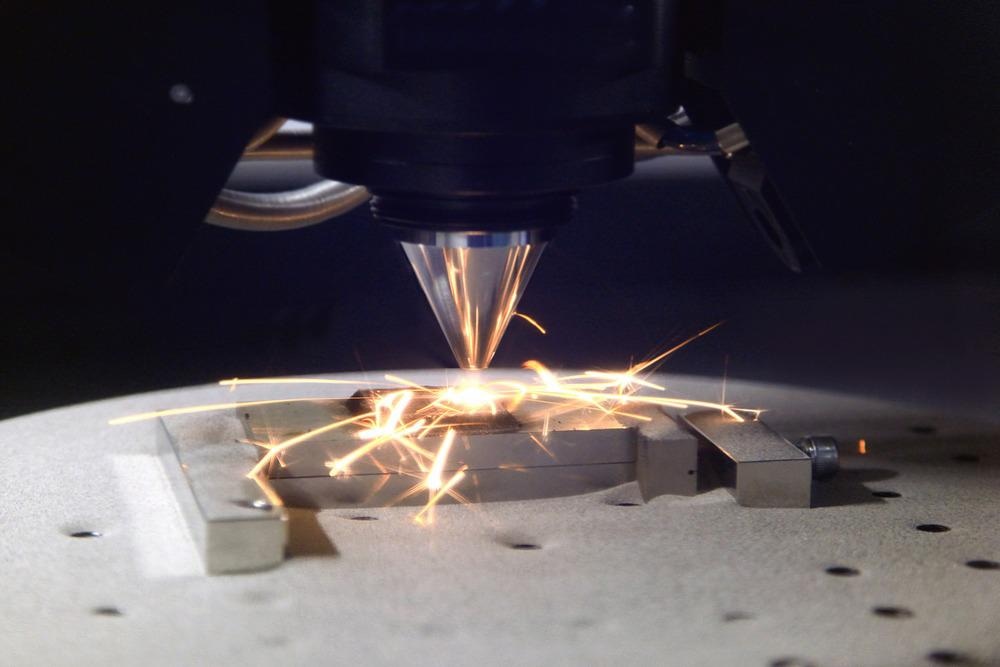
Study: Computational design of a crack-free aluminum alloy for additive manufacturing. Image Credit: sspopov/Shutterstock.com
Background
Because of their high specific strength and low density, aluminum alloys are becoming increasingly popular for laser additive manufacturing. However, various faults caused by the laser powder bed fusion (LPBF) process limit their application. The occurrence of hot cracking is a serious challenge for LPBF when producing aluminum.
As a result, there is an increasing interest in producing crack-free additive produced aluminum alloys with excellent mechanical qualities. Computational alloy design is gaining popularity as more tools are becoming available to improve the approach.
Numerical approaches, such as the CALPHAD method, have been effectively used to predict precipitation, microstructure, and phases, and they tend to expand the alloy design space greatly. Statistical learning approaches can be used to anticipate attributes based on data accessible in the literature when physically-based prediction is unattainable. These prediction models are then fed into an optimization algorithm, which reduces the number of computations required and leads to the creation of novel alloy compositions.
About the Study
In this study, the authors proposed a design technique based on the lowering of the brittle temperature range to reduce hot cracking, as well as additional optimization criteria for phase fractions and solid solution strengthening to maintain mechanical strength and ductility.
For a speedy and efficient identification of the ideal composition, the optimization functions were included in an aggregated genetic algorithm. After LPBF processing, the mechanical characteristics of this novel alloy were established.
The team used metaheuristic algorithms, such as mono-objective or multi-objective genetic algorithms for additive manufacturing of aluminum alloys. The ultimate goal was to develop new aluminum alloys with outstanding mechanical properties and to simultaneously prevent hot cracking during the LPBF process.
The proposed design guidelines were validated by testing them on standard alloys such as 6061, AlSi10Mg, and 7075. The Scheil-Gulliver model was used to calculate the evolution of the solid fraction during the cooling stage. The alloy was created, gas atomized, and put through LPBF tests.
The researchers performed the computation with the TC-Python application programming interface (API), and the difference in temperature between the lower and upper solid fraction limits was simply derived from the model output. At a constant temperature characteristic of typical aging treatment, the total fraction of the face-centered cubic (fFCC) phase was estimated at equilibrium. Thermo-Calc and the TC-Python API were used to calculate a single-point equilibrium. Further, an aggregated multi-objective genetic algorithm was also employed.
Observations
The proposed novel composition had similar solid solution precipitation and strengthening characteristics to the 6XXX and 7XXX series, but at a lower cost than AlSi10Mg. Once the process settings were determined, the powder demonstrated that it was suitable for the LPBF process, with no cracks and a small fraction of porosities. The design criterion relied on was both effective and valid in addressing the hot cracking problem. The alloy was made up of a main FCC-Al phase with intermetallic phases Al9FeNi and Mg2Si.
The order in which these phases form varied was dependent on the cooling speed. The phases were formed by the interdendritic segregation in the powder, although the phases formed concurrently in the LPBF process. The alloy had an elevated strength that was comparable to the 7075 grade produced in traditional methods, but it had a low ductility. This observed brittle behavior was linked to the presence of porosities and the creation of secondary phases, which resulted in low seam cohesiveness.
The proposed algorithm produced a new grade with a composition comprised of 6.7% of Al-Mg, 3% of Si, 1.4% of Ni, 1% of Zn, and 0.3% of Fe.
Conclusions
In conclusion, this study elucidated the development of novel design criteria for a new aluminum alloy to reduce the risk of hot cracking and improve the corresponding mechanical qualities. The Scheil model was used to calculate the brittle temperature range, which was then minimized to lower the hot cracking susceptibility.
To take advantage of the solid solution effect, the content of solute components was increased. To prevent the uncontrolled production of brittle phases, some criteria on the precipitation fraction were included. To find the best composition, these objective functions were combined in an aggregated genetic algorithm.
The algorithm identified a promising alloy with a brittle temperature range of only 9 °C and a 90% FCC phase composition, with some Al9FeNi and Mg2Si precipitates. The authors emphasized that to improve mechanical properties, future iterations of the algorithm should examine additional features such as the nature of the intermetallics generated after solidification and the capacity to precipitate or dissolve these phases during annealing or aging treatments. They also believe that these extra objective functions will improve the relevance of the chosen alloy while also increasing the multi-objective algorithm's complexity.
More from AZoM: What are Profile Roughness Parameters?
Disclaimer: The views expressed here are those of the author expressed in their private capacity and do not necessarily represent the views of AZoM.com Limited T/A AZoNetwork the owner and operator of this website. This disclaimer forms part of the Terms and conditions of use of this website.
Source:
Dreano, A., Favre, J., Desrayaud, C., et al. Computational design of a crack-free aluminum alloy for additive manufacturing. Additive Manufacturing 102876 (2022). https://www.sciencedirect.com/science/article/abs/pii/S2214860422002755