By Surbhi JainReviewed by Susha Cheriyedath, M.Sc.Oct 13 2022
In an article recently published in the open-access journal Materials, researchers discussed the artificial neural network (ANN) prediction of the compressive strength of waste-based concretes.
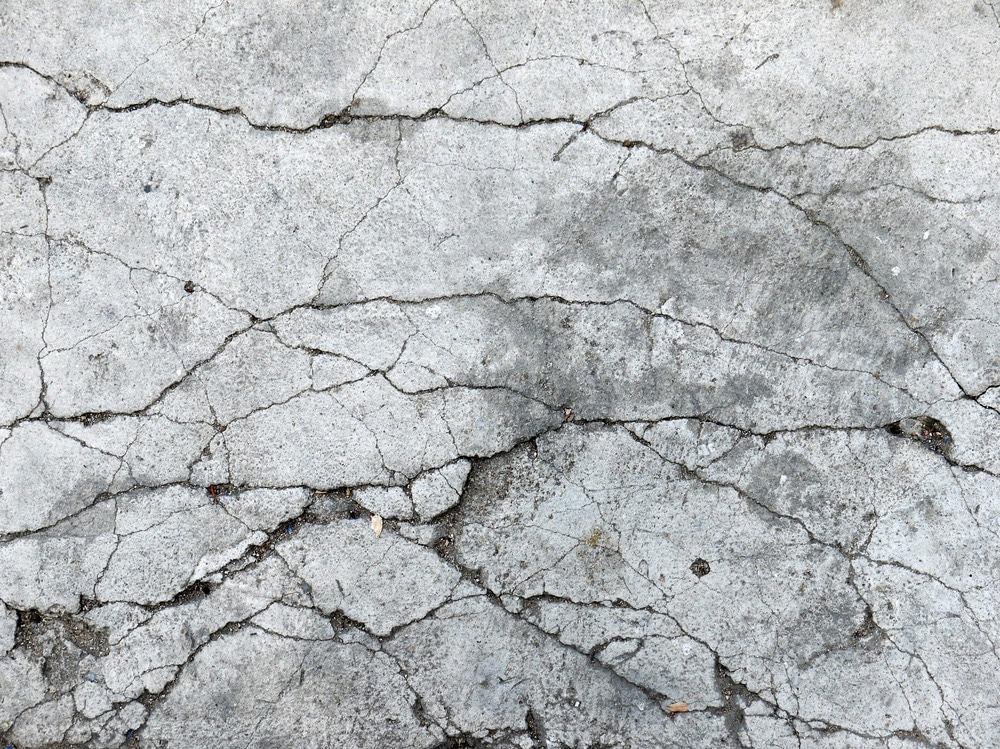
Study: Prediction of the Compressive Strength of Waste-Based Concretes Using Artificial Neural Network. Image Credit: komkrit Preechachanwate/Shutterstock.com
Background
The environment and climate are severely impacted by excessive material use and wasteful resource utilization. In terms of volume and turnover, concrete has overtaken all other building materials as the most significant. Over 8–12 billion tons of natural aggregates are consumed annually by the concrete industry worldwide.
Because of this, there is growing interest in using binders made from other waste materials, and their effectiveness has been established. Research on comprehensive techniques for forecasting the strength of concrete or mortar made from byproducts is still lacking. Many studies have recently used extrapolation techniques to predict the compressive strength of cementitious materials. ANN appears to be a pertinent and effective technique among these approaches.
ANNs can be utilized to tackle engineering difficulties, according to several recent studies. To estimate the compressive strength of concretes, the necessary data may be complicated or insufficient. Numerous research conducted in the early 2000s demonstrated the enormous potential for improving mix proportioning and predicting the properties of concrete.
One of the best options for protecting the environment and natural resources may be the usage of additional cementitious material. The substitution of clinker with other materials is one of the best-known methods for minimizing the environmental impact of cement. Additionally, natural fillers, including pozzolans, calcined clays, and limestone, have demonstrated their suitability for use in concrete.
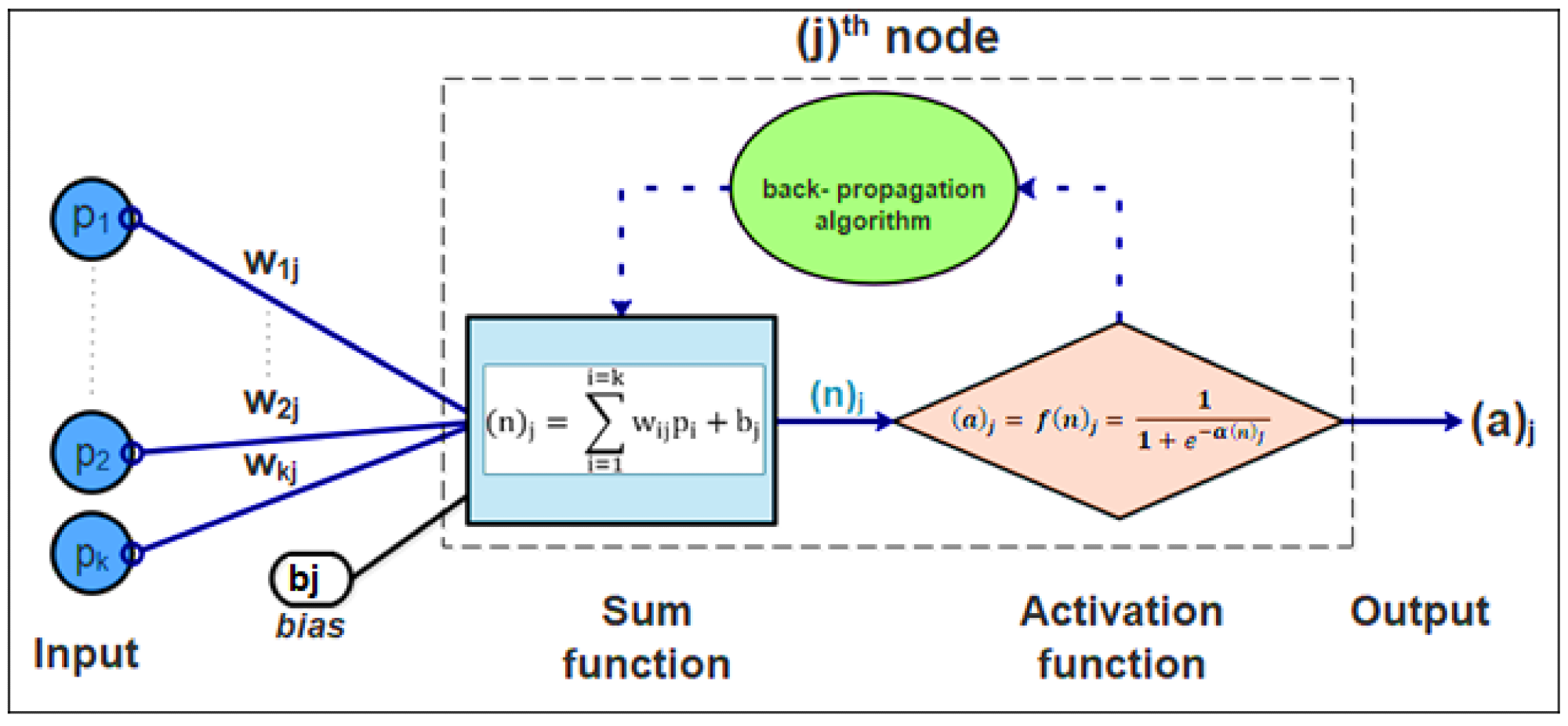
Architecture of artificial node and its interactions in the neural network. Image Credit: Amar, M et al., Materials
About the Study
In this study, the authors anticipated the compressive strength of several waste-based concretes using an ANN. Metakaolin, limestone filler, marble waste, silica fume, recycled aggregates, fly ash, and crushed, granulated blast furnace slag were a few of the mineral additives explored in the sample. The experimental data for 1303 distinct combinations, which were acquired from 22 bibliographic sources for the process of ANN learning, served as the basis for the proposed method.
The team organized and processed the data for training and testing the model using a multilayer feedforward neural network model. Following the kind of cement, water content, replacement ratio, water to binder ratio, amount of superplasticizer, etc., the model had 18 inputs. The neural network module of the MATLAB program was used to build and apply the ANN model. The ANN exhibited a great capacity for forecasting the compressive strength of concrete and was particularly precise with a satisfactory accuracy with R2 equal to 0.9888 and MAPE = 2.87%, according to the results predicted by the suggested neural network model.
The researchers examined the effectiveness of ANN modeling in foretelling concrete qualities. The goal was to create and implement artificial intelligence (AI)-based tools that could forecast the characteristics of concretes that contained byproducts that were employed as additional cementitious ingredients. This study emphasized the potential of utilizing an ANN to forecast the properties of environmentally friendly concretes with satisfying and dependable results. The proposed method used machine learning to examine the simultaneous set of numerous supplemental cementitious materials.
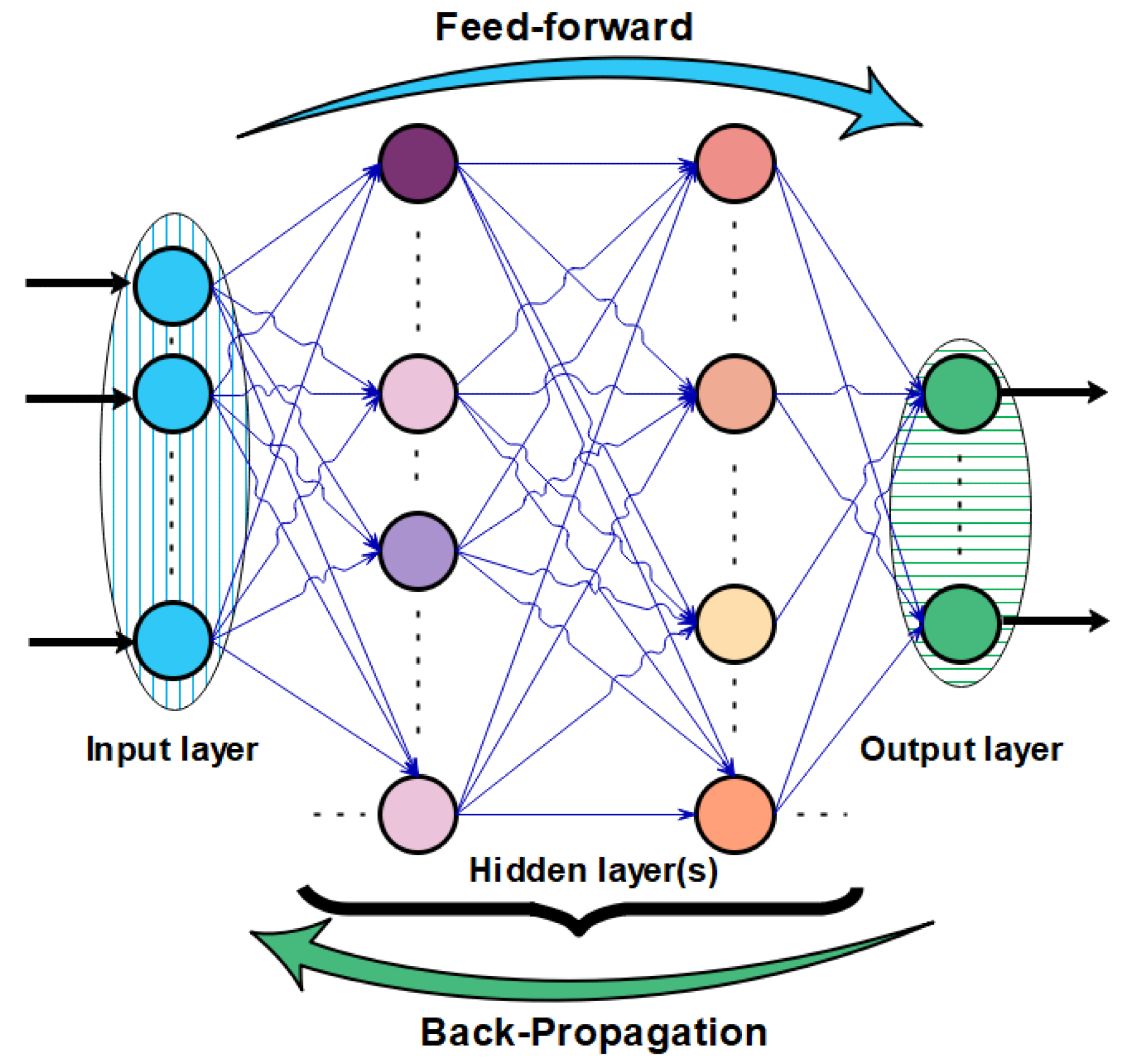
Architecture of applied neural network and learning process principle. Image Credit: Amar, M et al., Materials
Observations
For validation, training, and testing, respectively, the coefficients of determination R2 are 0.9763, 0.9982, and 0.9566. The difference between predicted and experimental values was relatively minor, as indicated by the root mean square (RMSE) value of 2.91 MPa. According to MAPE, the anticipated compressive strength varied from the experimental data by an average of 2.87%. This demonstrated that there was little variation between expected and actual results. It was observed that the data must lie on a 45° line where the network outputs and targets were equal for the fit to be ideal.
By learning the intricate details of the water-cement ratio, the age of the concrete, the cement and admixture quantity, etc., the proposed ANN model could forecast compressive strength with high accuracy. The outcomes showed that multilayer feedforward ANNs were useful tools for predicting concrete's compressive strength. The R2, MAPE, MSE, and MAE model errors demonstrated slight discrepancies between experimental and predicted values. The findings implied that the use of ANN for concrete compressive strength prediction was appropriate.
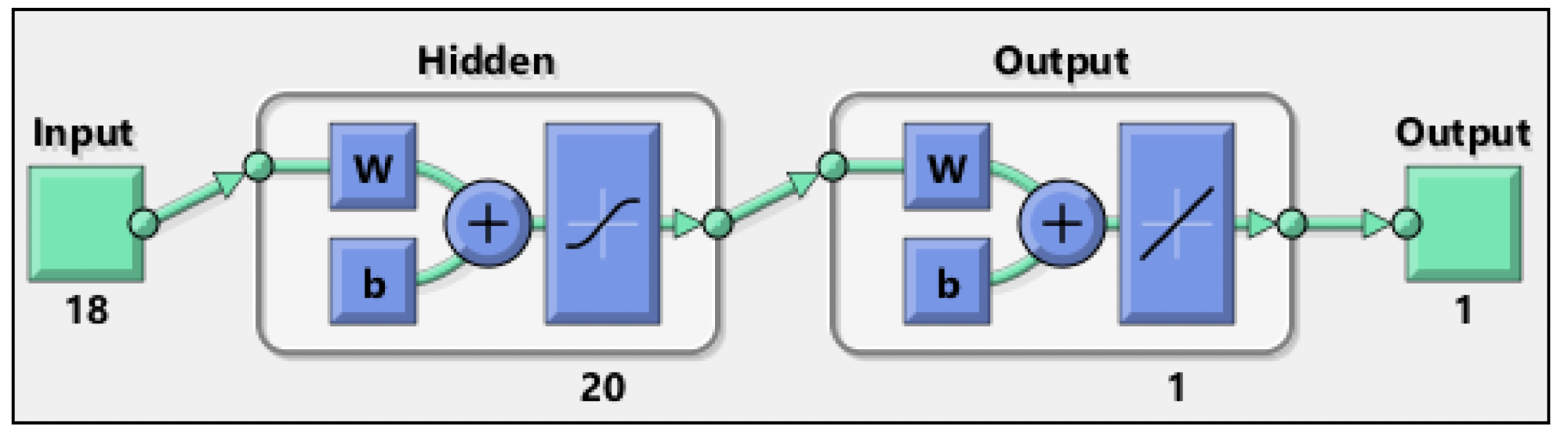
Artificial neural network architecture from MATLAB software (R2022a). Image Credit: Amar, M et al., Materials
Conclusions
In conclusion, this study forecasted the compressive strength of waste-based concretes using an artificial neural network. The learning methodologies, architecture, and feedforward and backpropagation approaches were all explained. Then, 1303 concrete formulations from 22 separate investigations were included in a bibliographic dataset produced from the literature.
The authors mentioned that they will examine the application of decision tree regression (DTR) to the prediction of concrete qualities in a future study. They stated that the proposed machine-learning technique is an effective strategy.
References
Amar, M., Benzerzour, M., Zentar, R., et al. Prediction of the Compressive Strength of Waste-Based Concretes Using Artificial Neural Network. Materials, 15(20), 7045 (2022). https://www.mdpi.com/1996-1944/15/20/7045
Disclaimer: The views expressed here are those of the author expressed in their private capacity and do not necessarily represent the views of AZoM.com Limited T/A AZoNetwork the owner and operator of this website. This disclaimer forms part of the Terms and conditions of use of this website.