Over the past years, there was a dramatic increase in the occurrence of allergies: the prevalence of allergic diseases across the world has doubled during the past 15-20 years.
It has been estimated that respiratory allergies (commonly called pollinosis) affect 15 to 25% of people in western countries. In many cases, these allergies are triggered when there is increased allergenic pollen in the atmosphere, particularly during the blooming season.
Pollen’s allergenicity varies according to the species and hence it is vital to identify, characterize, and quantify the airborne pollen to get a clear picture of the allergen exposure. Raman microspectroscopy offers a selective, rapid, and non-destructive identification of pollen particles.
Characterization of Pollen by Raman Spectroscopy
Pollen grains – ranging from 10 to 200 µm diameter based on the pollen type – are the male gametophytes of seed plants being produced as part of the sexual reproduction cycle. They are regarded as seasonal air pollutants, because pollen is only dispersed into the atmosphere at the time of flowering.
The pollen structure, the exine pattern (the grain’s outer wall), and morphology offer good taxonomic parameters. Presently these morphological parameters, acquired through electron or optical microscopy, are typically used for pollen identification. However, there are huge differences in the grains’ chemical composition level: depending on the floral origin, the amount of proteins – compounds largely responsible for the allergic disorders - may differ from 10 to 40% of the dry weight.
The Raman spectrum is affected by these changes in the molecular composition of the sample, thus making Raman spectroscopy an interesting tool for the identification and characterization of pollens.
As Raman spectroscopy is fast, highly chemically selective, non-destructive and able to quantify single grains, it provides an alternative to the purely optical-based technique of identification or to chemical characterization methods involving a purification step that may cause sample denaturation. The spectral differences of various pollen species are shown in Figure 1.
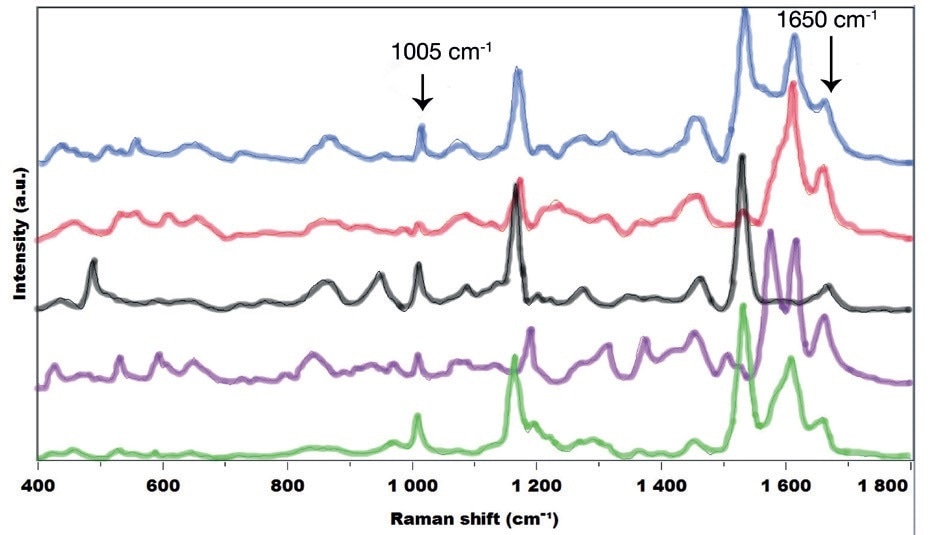
Figure 1. Raman spectra of 5 pollen from the species: Zea Mays (Corn, in blue), Quercus coccifera (Kermes Oak, in red), Lolium perenne (Ryegrass, in black), Parietaria judaica (Spreading Pellitory, in pink) and Salix atrocinerea (Grey Willow, in green).
An in-depth examination of the spectral features reveals the presence of proteins and amino-acids with the amide I band at 1650 cm-1 or the typical ring breathing mode at 1005 cm-1 characteristic from the phenylalanine, represented in every spectrum. In contrast, the carotenoids spectral features, indicated by the two intense resonance peaks at 1160 cm-1 and 1530 cm-1, are seen in the case of Lolium perenne, Salix atrocinerea, and Zea mays– and slightly for Quercus coccifera – while they are not present in the Parietaria judaica spectrum.
This demonstrates the diversity in the chemical composition of the different pollen species and the ability to capture these differences in the Raman spectra. Furthermore, the differences can be observed between various species of the same family as illustrated in Figure 2, which displays the spectra of pollen types belonging to the Oleaceae family. This implies that the identification can be done within a family at species level.
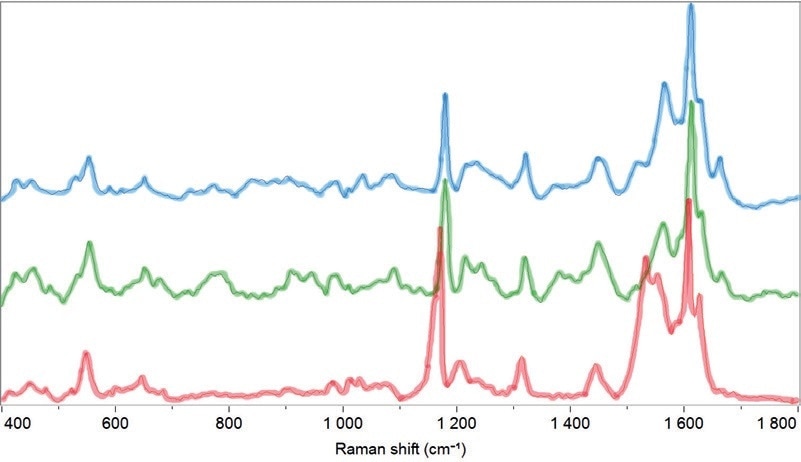
Figure 2. Raman spectra of Fraxinus floribunda (in blue), Ligustrum lucidum (in green), Olea europaea (in red) pollen, all belonging to the Oleaceae family.
Identification of Airborne Samples by Library Search
Since the spectroscopic and chemical examination of the pollen has become more and more important, the construction of Raman spectral libraries which include the different pollen types found in the atmosphere should be executed.
For this purpose, Raman spectra of 34 pollen types were recorded and collected in a spectral library. The list of the pollen types comprises of different families of shrubs, trees as well as weeds and grasses found in the atmosphere.
In order to test the ability of spectral identification by library search, a set of 10 airborne pollen spectra collected in summer and spring 2012 was tested against this spectral library. The identification to the right species is accurate for all of these 10 samples, as represented in Table 1, where the highest ranked reference spectrum matches the query spectrum. If the Hit Quality is higher, the match between the query spectrum and the library one becomes better.
Query spectrum |
Hit |
Name (Hit Quality) |
Query spectrum |
Hit |
Name (Hit Quality) |
1- Acer negundo_air (april) |
1st |
Acer negundo (888.2) |
6- Acer negundo_air (may) |
1st |
Acer negundo (795.4) |
2nd |
Acer negundo (888.2) |
2nd |
Parietaria judaica (746.9) |
3rd |
Quercus coccifera (821.6) |
3rd |
Rumex spp. (732.3) |
2- Betula pendula_air (april) |
1st |
Betula pendula (876.1) |
7- Acer negundo_air (may) |
1st |
Acer negundo (892.5) |
2nd |
Castanea sativa (851.1) |
2nd |
Quercus coccifera (827.4) |
3rd |
Corylus avellana (819.8) |
3rd |
Parietaria judaica (793.1) |
3- Platanus x acerifolia_air (april) |
1st |
Platanus x acerifolia (906.2) |
8- Platanus x acerifolia_air (may) |
1st |
Platanus x acerifolia (756.8) |
2nd |
Acer negundo (742.9) |
2nd |
Plantago lanceolata (728.5) |
3rd |
Parietaria judaica (710.1) |
3rd |
Acer negundo (692.4) |
4- Plantago lanceolata_air (april) |
1st |
Plantago lanceolata (909.2) |
9- Platanus x acerifolia_air (may) |
1st |
Platanus x acerifolia (726.3) |
2nd |
Platanus x acerifolia (720.9) |
2nd |
Parietaria judaica (718.5) |
3rd |
Parietaria judaica (675.8) |
3rd Castanea sativa (957.1) |
5- Acer negundo_air (may) |
1st |
Acer negundo (891.7) |
10- Castanea sativa_air (august) |
1st |
Castanea sativa (957.1) |
2nd |
Quercus coccifera (830.8) |
2nd |
Betula pendula (893.3) |
3rd |
Castanea sativa (828.8) |
3rd |
Quercus coccifera (853.2) |
Conclusion
The presented examples show that Raman microspectroscopy can be successfully applied to the identification and analysis of airborne pollen. Raman microspectroscopy does not require sample preparation, is rapid, and highly chemically selective, and hence it provides an alternative to the optical microscopic methods that depend only on morphological parameters. Furthermore, other spectroscopic techniques (such as SPRi) offer complementary analysis for the detection of allergens. The demand for such identification methods is increasing as pollinosis has become a major health issue, needing advanced analytical characterization tools.
Sources and Further Reading
- A. Guedes, H. Ribeiro, M. Fernandez-Gonzalez, M.J. Aira, I. Abreu, Talanta, 119, 473-478 (2014)
- F. Schulte, J. Lingott, U. Panne, J. Kneipp, Anal. Chem, 80, 9551-9556 (2008).
- Detection of birch pollen allergen in the air, HORIBA application note.

This information has been sourced, reviewed and adapted from materials provided by HORIBA.
For more information on this source, please visit HORIBA.