A growing number of academic and industrial research groups regard the integration of artificial intelligence-based algorithms into the 3D printing process as a promising approach to improve the quality and efficiency of 3D printing technology.
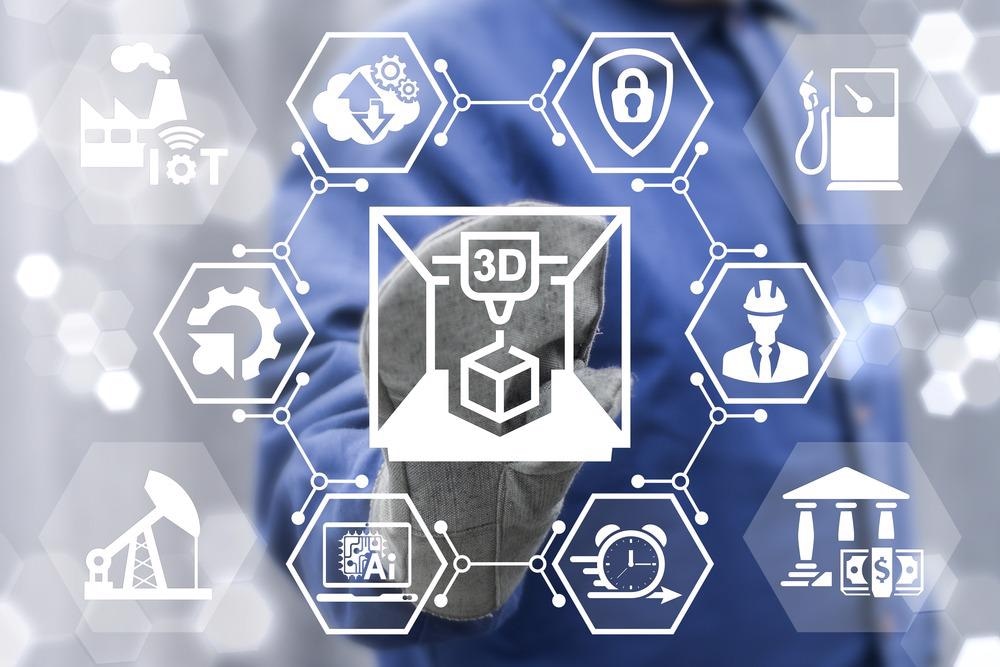
Image Credit: Panchenko Vladimir/Shutterstock.com
While industrial additive manufacturing, popularly known as 3D printing, has advanced to technological levels never seen before, quality control and monitoring of manufacturing defects still present a big challenge.
Additive manufacturing plays an important role in a wide range of industrial sectors, such as automotive, aerospace, biomedical, and others, by enabling the manufacturing of parts with complex geometry, internal structural details, and individually customized designs at low cost and in small production batches.
However, at the moment, the ability of additive manufacturing technology to produce components with complex shapes with minimal material waste cannot be fully exploited when manufacturing high-quality parts required to meet demanding standards. Depending on the type of 3D printing process involved in the production cycle, different manufacturing defects can compromise the consistency, reliability, and performance of the 3D-printed materials.
The factors that govern the quality of the 3D printing process are diverse. These can be related to the quality of the used source materials (plastic filaments, metal powder, or liquid photopolymers) or process-related, such as over- and under-extrusion, gas pockets in the sintered material, layer separation (lack of adhesion), and others. In most cases, these defects result in increased porosity and inferior mechanical properties of the manufactured part.
Establishing Process Control
In the first place, ensuring that 3D-printed components meet the required standards depends on the quality of the material used. Source material quality control is an ongoing challenge for most additive manufacturing companies.
In addition, a wide range of variables that can affect the quality of the final product span the entire additive manufacturing workflow, from the initial design to 3D printing and postprocessing. For example, these can be the path and intensity of the sintering laser (in a direct metal sintering process) or the filament extrusion speed (in a fused filament fabrication process). Other factors might include the design of the support structures or even the number of times the metal powder has been recovered and reused.
Currently, trial and error is the most common approach for the optimization of the 3D printing process to achieve a consistent and reproducible component quality. This approach usually involves repeating manufacturing steps multiple times and extensive testing of the final part.
The result is an expensive and inefficient manufacturing process that negates the primary benefits of additive manufacturing – the cost-efficient manufacture of small batches.
Reducing Human Error
Most of the additive manufacturing processes still require additional human intervention. This can involve the initial design of the component, postprocessing (removal of supports and finishing), and manual testing of the final products' compliance with the requirements. Thus, reducing the likelihood of human error is of critical importance to the elimination of 3D printing defects.
Additive manufacturing experts recognize the need for more sustainable and feasible methods to process and quality control. One of the most promising ways to achieve this is by using artificial intelligence (AI) algorithms to automate the most critical steps in the 3D printing process.
Efficiency in the Prefabrication Stage
AI-based software packages, like Netfabb from Autodesk and Agile Metal Technology from Sculpteo (a BASF subsidiary), can evaluate and optimize design files for 3D printing by using machine learning algorithms in the so-called generative design approach. Manufacturers can input the desired design parameters and the AI analyzes the design requirements to find the most efficient production pathway.
Automated Defect Detection and Closed-Loop Control
The development of closed-loop control systems has been a long-standing key goal for additive manufacturing engineers, becoming a possibility in the last few years owing to the rapid development of advanced AI applications.
Researchers at General Electric's Additive Research Lab in Niskayuna, New York, developed a proprietary machine-learning platform that uses high-resolution cameras to monitor the printing process layer-by-layer and detect streaks, pits, voids, and other defects often invisible to the naked eye.
The data is matched in real-time against a defects database pre-recorded by using computer tomography (CT) imaging. With the help of high-resolution imaging and CT scan data, the AI system can be trained to predict problems and detect defects during the printing process.
A similar integrative machine learning approach is employed by Ai Build, a London-based company specializing in the development of automated AI-based 3D printing technology, to create a smart extruder for additive manufacturing.
It is a high-precision attachment to industrial robotic arms capable of 3D printing large objects at high speed with great accuracy. By combining advanced AI algorithms with sensor data processing in real-time, the smart 3D printing extruder can detect any problems and makes autonomous decisions to achieve the best possible print quality.
AI Creates Novel 3D Printing Materials
A University of Cambridge spin-off company called Intellegens employed machine learning algorithms in its Alchemite platform to develop new materials for 3D printing. The company has successfully used the AI platform to create a novel nickel-based alloy suitable for the direct laser deposition manufacturing process. The deep learning capabilities of Alchemite enable the use of a large database of material properties to pinpoint the optimal alloy composition for the best processability and quality of the final product.
So far, the focus of using AI in additive manufacturing is on enhancing design, improving the efficiency of the 3D printing processes, and enabling autonomous manufacturing. Soon, advanced AI solutions can help to reduce design complexity, lower the knowledge threshold for additive manufacturing industries, and improve cybersecurity in the field.
References and Further Reading
Zhu, Z., et al. (2021) 3D-printed multifunctional materials enabled by artificial-intelligence-assisted fabrication technologies. Nat Rev Mater 6, 27–47. Available at: https://www.nature.com/articles/s41578-020-00235-2
Paraskevoudis, K., et al. (2020) Real-Time 3D Printing Remote Defect Detection (Stringing) with Computer Vision and Artificial Intelligence. Processes 8, 1464. Available at: https://www.mdpi.com/2227-9717/8/11/1464
K. Sertoglu (2020) Argonne scientists use machine learning to predict defects in 3D printed parts[Online] www.3dprintingindustry.com Available at: https://3dprintingindustry.com/news/argonne-scientists-use-machine-learning-to-predict-defects-in-3d-printed-parts-174544 (Accessed on 23 November 2021)
C. Valdivieso (2020) Why combine artificial intelligence and 3D printing? [Online] www.3dnatives.com Available at: https://www.3dnatives.com/en/artificial-intelligence-and-3d-printing-060120204 (Accessed on 23 November 2021)
C. Valdivieso (2019) Ai Build implements AI to detect and correct 3D printing errors in real time [Online] www.3dnatives.com Available at: https://www.3dnatives.com/en/ai-build-3d-printing-errors-110620195 (Accessed on 23 November 2021)
Disclaimer: The views expressed here are those of the author expressed in their private capacity and do not necessarily represent the views of AZoM.com Limited T/A AZoNetwork the owner and operator of this website. This disclaimer forms part of the Terms and conditions of use of this website.