In a pioneering move, Tokyo University of Science has made a remarkable stride in material science. Their latest development involves a deep learning model adept at pinpointing new quasicrystalline phases in multiphase crystalline materials, a feat previously mired in complexity and time constraints.
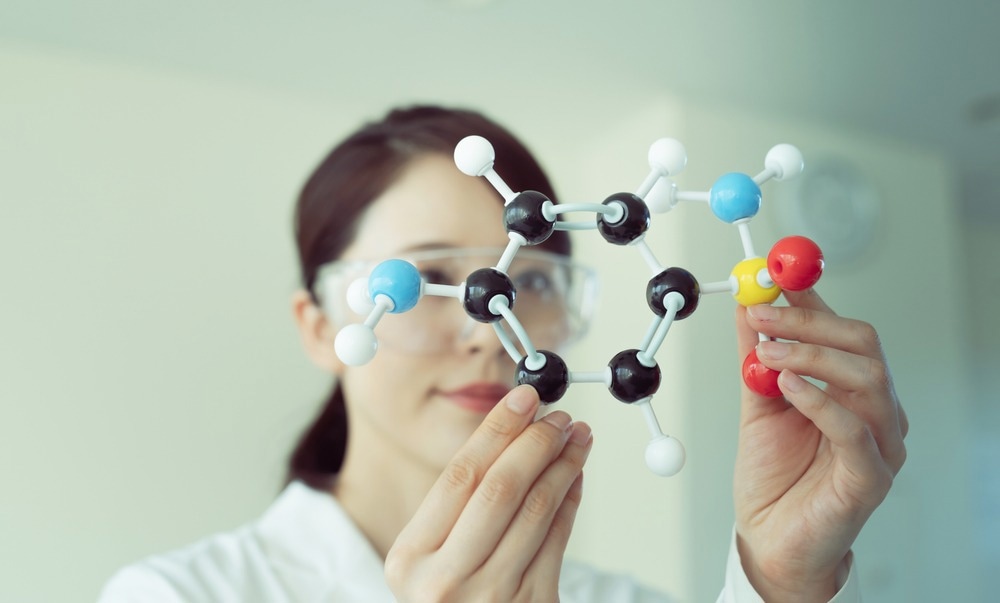
Image Credit: metamorworks/Shutterstock.com
Emerging Challenges in Crystalline Material Identification
The current landscape of identifying crystalline structures, integral in sectors like pharmaceuticals and electronics, hinges on powder X-ray diffraction. This process, however, grapples with challenges when it comes to multiphase samples that incorporate various crystal types. Such complexities necessitate a novel approach for swift and accurate identification.
Deep Learning's Breakthrough
In this context, the research team at Tokyo University of Science, under the guidance of Junior Associate Professor Tsunetomo Yamada, unveiled a machine learning 'binary classifier' model. This model, remarkable for its application of convolutional neural networks, is trained to recognize icosahedral quasicrystal (i-QC) phases in multiphase samples. This phase is notable for its long-range order and unique diffraction patterns.
Achievements and Potential
The model has shown an impressive over 92% prediction accuracy, successfully identifying an unknown i-QC phase in Al-Si-Ru alloys. This opens a plethora of possibilities in identifying new quasicrystalline phases, including decagonal and dodecagonal quasicrystals, across a wide array of materials.
This innovation not only streamlines the phase identification process but also paves the way for discovering new materials vital for addressing future challenges in energy, electronics, and environmental sustainability. The collaboration and interdisciplinary approach of this research underscore the transformative potential of integrating AI and deep learning in scientific exploration.
Source
Accelerating the phase identification of multiphase mixtures with deep learning (no date) Tokyo University of Science. Available at: https://www.tus.ac.jp/en/mediarelations/archive/20231102_6238.html (Accessed: 20 November 2023).