Given how imperative it is to support and sustain a Nation’s economy and quality of life, the structural health of its infrastructure demands constant attention. Key civil engineering components such as pipelines, bridges, buildings, and dams, together with other timber and steel structures, require continuous inspection, monitoring, and maintenance.
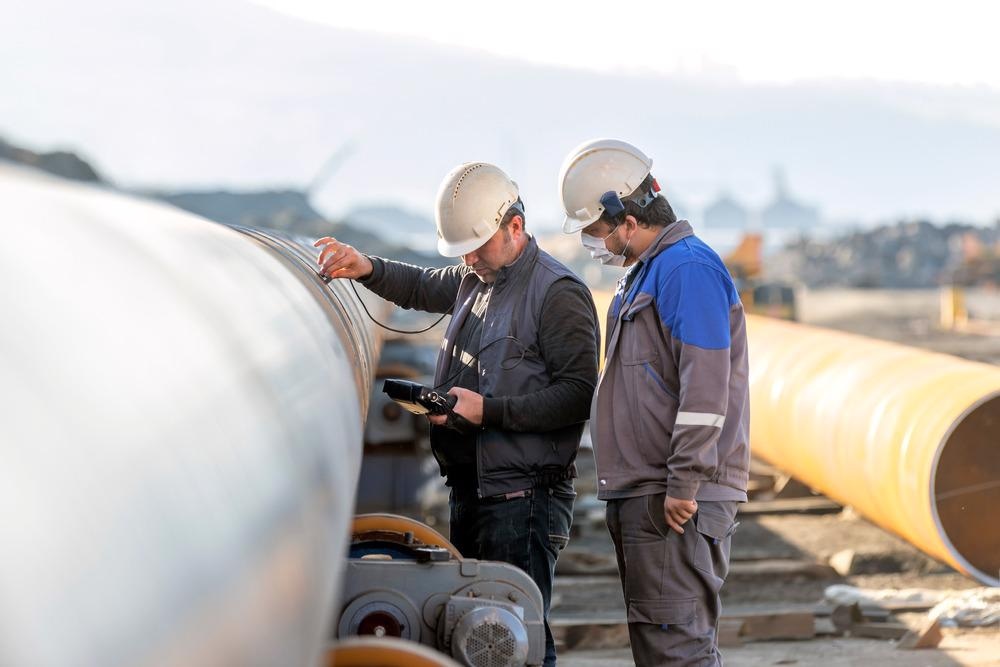
Image Credit: Funtay/Shutterstock.com
Structural Health Monitoring (SHM) is critical in ensuring safety and reliability, areas easily compromised if not monitored with a broad range of necessary tools and techniques. Locating concealed damage or mapping deterioration within a structure and the underlying cause usually involves non-destructive testing (NDT). These are remote, often contactless probes that cause no damage to the material integrity of the material under test (MUT).
Cracks, Moisture, and Strain
The measuring parameter, structure type, and physical properties dictate which NDT technique is selected in any given situation. Although, a combination of methods is often deployed. Utilizing multiple techniques enhances the measurement process with additional parameters, which can help to improve accuracy.
Parameters in SHM applications that share a degree of commonality tend to require monitoring. Those that receive the most attention include the presence of cracks, moisture, vibration, load, and the measure of strain.
In selecting and designing a NDT, consideration must be given to whether the technique is noisy and whether the contrast in physical properties between the target and its surroundings will have an impact. Planning resolution requirements for measurements, alongside the degree of penetration, is also necessary.
Regarding NDT-based SHM deployment, the most common challenge revolves around analysis and the ability to interpret the resulting data.
Laser Scanning with Sound
Focused pulses of coherent light underpin the remote sensing technology of laser scanning. In essence, distances are calculated from the transmission of light beams reflected back from a structure. Its innate processing capacity primarily drives the popularity of laser scanning in SHM.
Large and accurate quantities of data allow detailed documentation to be attained, especially in the context of monumental structures that need inspecting. 3D maps rich in detail emerge from the scans generated by what is known as “point clouds”. These clouds are composed of all collected points from the MUT. The maps can reveal much about its prevailing characteristics, exposing the extent of deterioration. Over time, this technique can easily provide visibility of damage progression.
In terms of hybrid techniques, Qiu Q et al. have shown that an economical detection scheme can be derived from combining laser reflection and acoustic-laser methods. This enables instant identification of defects in concrete systems that have fiber-reinforced polymer incorporated into them, with the added capability of determining their size.
Going Underground
With attractive features such as low cost and rapid monitoring, ground-penetrating radar (GPR) is frequently used in civil engineering SHM applications, especially in defect inspections where fractures or voids might undermine concrete and masonry stability.
In this method, penetration depth has to be balanced with the required solution. Lower penetration depth, for example, is the payoff for a higher operating frequency and hence higher resolution. Reflected energy differences from an altered signal can determine the progression of corrosion within structures. Steel rods that tend to be inserted into reinforced concrete are a good example, as their geophysical nature makes it an ideal sub-surface for technology that utilizes microwaves.
Deep Machine Learning
As previously mentioned, the ability to interpret data arising from NDT can be challenging. However, the introduction of artificial intelligence (AI) has helped to address these issues in recent years.
Jang et al. explored automating the concrete crack detection process using deep machine learning (DL), a subsection of AI. Machine learning possesses the ability to project future outcomes utilizing the development of trainable algorithms that learn from newly presented data. In this study, a hybrid of infrared (IR) thermography and image scanning was adopted. The working principle of the IR method exploits surface temperature change measurements that result from warping, compression, or tension.
Thermal images are from data captured from emitted heat at any temperature. Newly inputted data is then processed into layers that are stacked into deep neural networks (DNN), which can produce precise, multiple crack visualizations.
In essence, researchers created a system that minimized false results, automatically establishing a broad range of cracks from micro to macro scale.
Future developments of NDT-based SHM
Future research and development into NDT-based SHM will be driven by robotics, and any methodologies can eliminate the current limitations to DL application within the context of structural health.
Remotely operated vehicles (ROVs), for instance, will experience greater uptake as previously prohibited testing becomes possible with their adoption. These, in combination with aerial vehicles (UAVs,) will facilitate, for example, probing remote areas of potentially inaccessible structural vulnerability.
Existing trained models have performance limitations, a direct consequence of the small number of structural system image databases for SHM purposes. Expansion in this area is essential and requires ongoing development. With regards to new or emerging conditions, NDT-based SHM suffers from poor agility. Accommodating factors such as rising pollution levels, for example, presents a challenge and the basis for future development.
Furthermore, in-situ DL-based SHM involving UAVs for vision-based measurements suffers from external disruption, experiencing environmental factors, and extreme weather conditions.
Perfect simulations are a challenge. When incremental changes occur and cause progressive damage due to adverse conditions or strong winds, structures can oscillate. Wide-spanning suspension bridges are particularly susceptible to this problem. Future research is likely to focus on developing solutions to these issues.
References and Further Reading
Qiu, Q. and Lau, D., (2017) A novel approach for near-surface defect detection in FRP-bonded concrete systems using laser reflection and acoustic-laser techniques. [online] Available at: http://dx.doi.org/10.1016/j.conbuildmat.2017.03.024 [Accessed August 2021].
Jang, K., Kim, N. and An, Y., (2019) Deep learning–based autonomous concrete crack evaluation through hybrid image scanning. Structural Health Monitoring, 18(5-6), pp.1722-1737. Available at: https://doi.org/10.1177/1475921718821719 [Accessed August 2021].
Feng, D. and Feng, M., (2018) Computer vision for SHM of civil infrastructure: From dynamic response measurement to damage detection – A review. Engineering Structures, 156, pp.105-117. Available at: https://doi.org/10.1016/j.engstruct.2017.11.018 [Accessed August 2021].
Disclaimer: The views expressed here are those of the author expressed in their private capacity and do not necessarily represent the views of AZoM.com Limited T/A AZoNetwork the owner and operator of this website. This disclaimer forms part of the Terms and conditions of use of this website.