In a paper recently published in the journal Additive Manufacturing, researchers proposed two surrogate models efficient for reproducing the nonlinearity of the heat equation with respect to laser powder bed fusion (LPBF), while comparing its performance in online execution time and accuracy.
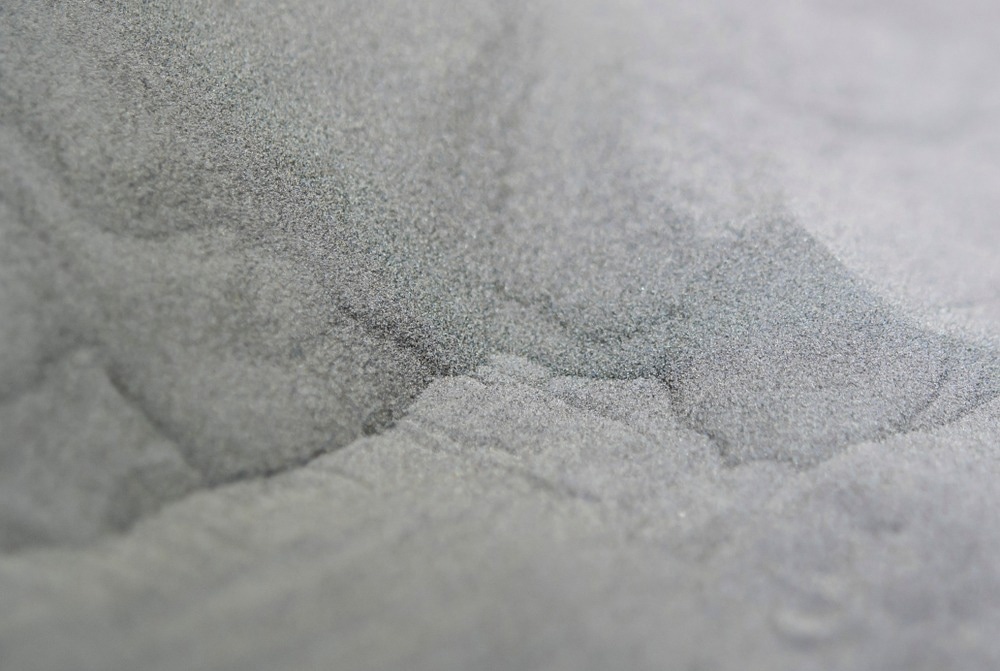
Study: Time-efficient surrogate models of thermal modeling in laser powder bed fusion. Image Credit: MarinaGrigorivna/Shutterstock.com
Background
In order to create physical objects from 3D geometric patterns, the additive manufacturing (AM) process known as LPBF sequentially spreads and melts tiny layers of powder. Digital twins of LPBF that help to approach the "first-time-right" goal are attracting increased attention as a result of the ongoing issue of quality assurance.
The numerical solver using finite element methods (FEM), such as the thermal model, has been the subject of extensive research with experimental validation. However, the high time cost prevents its use both prior to and during printing operations, making a quick yet precise heat transfer modeling of LPBF an important research topic.
About the Study
In this study, the team provided two distinct surrogate models (SMs) that emulate the FEM. A low-dimensional representation involving the relative distance between different temperature profiles was learned first by the SM, the reduced Gaussian process (GP) emulator, and then later extrapolated to the prediction of high-dimensional temperature. The second SM was a rapid numerical solution with FEM and used a sketched emulator with local projection.
The team created a simplified GP emulator that constructed a quick model of the distances between temperature profiles using data-driven computation. The researchers suggested low-dimensional local projections and randomized sketching in the sketched emulator with local projection to expedite FEM and develop an SM.
Furthermore, a subsampling method was created based on a comparative distance predictor that converted input parameters into relative distances to choose training temperature snapshots. Since it was a data-driven method, the predictive power of the heat simulator with FEM depended on locating representative training data sets. Controllable LPBF process parameters, such as beam size, laser power, scan speed, preheating temperature, time, and others, were chosen as the training inputs.
Observations
In the present study, the team developed a numerical experiment that involved a printing procedure evolving from one to three layers on a parallelepiped AlSi10Mg powder bed in an argon atmosphere. As a high-fidelity (ground-truth) heat simulation, the FEM-based numerical solver equipped with fine spatial-temporal discretization was used as a reference model. The reference simulator and the two SMs, the reduced GP emulator labeled as F1 and the sketched emulator with local projection denoted as F2, were compared for model correctness and online time costs.
Using a nonlinear dimension reduction technique, the number of GP decreased during the pre-processing of F1. The target of the dimension reduction (r) and neighborhood size (g) were two crucial values in this strategy. Additionally, it was noted that as more layers are stacked, the proportion of dimension reduction decreases. Although there was a downward trend, the reduction was not significant enough to have a negative impact on online time costs.
The study showed that the average total online execution time for the one to three layers domain was 41.50, 41.67, and 43.51 seconds, respectively. In contrast, the time required for training and data generation was similar for F1 and F2. The total online execution time was divided into projection, sketching, and prediction. This indicated that the online running time associated with F2 was less sensitive to more characteristics from the discretized domain than FEM. However, there was still a noticeable decrease in the online execution time, saving 82.17%, 83.50%, and 84.27% of the time cost for the one to three layers domain, respectively.
While F2 generated precise results in a longer execution time, F1 was able to forecast temperatures very efficiently but with significantly higher prediction inaccuracies. Thus, F1 was found to be more biased toward reducing time costs, while F2 was biased toward prediction accuracy. F1 heavily depended on finding a representative training data set since it is a data-driven approach with nonlinear dimension reduction, which ensured accuracy.
Although all subsampled temperature snapshots contributed equally to the generator of local projection bases, relative distance prediction had a lower accuracy requirement. In contrast, in F1, the order and value of relative distance prediction were important since they were used to determine the accurate weights in order to extrapolate the predictions of high-dimensional temperature. This distinction proved the comparatively higher robustness of F2 in a different training data sampling.
Conclusions
Overall, the study summarized two time-effective SMs along with a nonlinear thermal model of LPBF that utilized FEM. By changing the domain geometry, heat source model, and other factors, the two SMs developed for LPBF can be used for various thermal-driven AM. The focus of future research can be on understanding how to effectively integrate these two SMs into the thermal analysis of LPBF, including uncertainty management, defect prediction, parameter optimization, uncertainty management, and closed-loop controls.
More from AZoM: The Use of AI in Chemical Processes
Disclaimer: The views expressed here are those of the author expressed in their private capacity and do not necessarily represent the views of AZoM.com Limited T/A AZoNetwork the owner and operator of this website. This disclaimer forms part of the Terms and conditions of use of this website.
Source:
X. Li and N. Polydorides, Time-efficient surrogate models of thermal modeling in laser powder bed fusion, Additive Manufacturing (2022), doi: https://www.sciencedirect.com/science/article/pii/S2214860422005115?via%3Dihub