Two studies published in 2022 demonstrate machine learning techniques employed to reduce uncertainty in atomic force microscopy (AFM). Making AFM more accurate with artificial intelligence (AI) could make the technology more accessible around the world, as the algorithmic advantage such approaches provide can return reliable results from investigations with less mechanically accurate instrumentation.
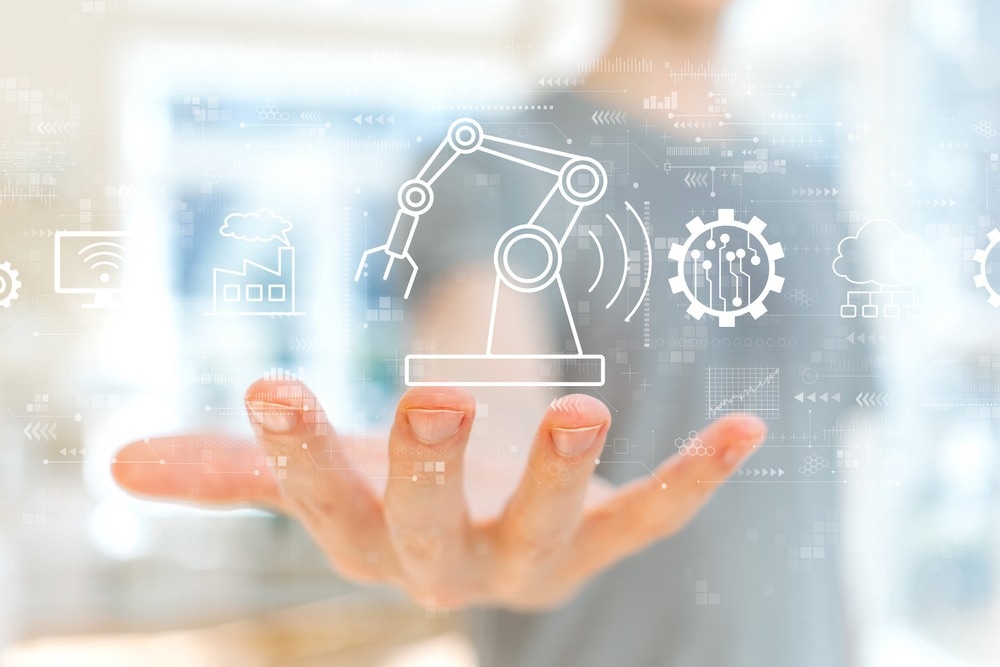
Image Credit: TierneyMJ/Shutterstock.com
AFM devices detect atomic forces between an extremely sharp pointed probe and the sample under investigation. The probe is attached to a cantilever, and at the other end is a highly sensitive sensor.
The sensor records nanoscale deviations in the cantilever that provide information about the surface nanostructure of the sample.
AI refers to computer processes that complete a task in a way that appears intelligent. They accomplish this with large amounts of data and processing power and algorithms that tell the computer what to do with the data at hand.
Machine learning is a classic example of AI. Machine learning algorithms can set their own subtasks and extra parameters to perform better the tasks originally set for them.
Machine learning may be a suitable way to tackle uncertainty in AFM. Uncertainty necessarily comes into AFM calculations, and simply refers to the margin of error that the instrumentation system is rated for.
Manufacturers and researchers report uncertainty as the mean and standard deviation of a number of replicated experiments.
Machine learning methods are well suited to the task of reducing uncertainty due to their ability to iteratively improve with multiple replicated data points to work with over time.
Two recent studies have explored machine learning approaches for reducing uncertainty in AFM.
Reducing AFM Nanomechanical Measurements’ Uncertainty with a Selection of Appropriate Contact Model
In an article published in the European Journal of Mechanics in 2022, nanofabrication and nano analysis engineers from the National Cheng Kung University, Tainan, Taiwan, proposed a supervised machine learning (SML) algorithmic framework to help reduce uncertainty in AFM measurements.
The machine learning method worked by selecting an appropriate contact model; in other words, establishing the best parameters to evaluate AFM measurement accuracy with.
The SML technique used the contact model it selected to evaluate elastic modulus depending on features of the force curve recorded in the AFM instrument. They extracted these features for the SML’s classifier algorithm. This algorithm then learns the appropriate contact model by analyzing these outputs.
Five separate classifiers were used alongside four contact models. These were trained on (that is, the algorithms scraped information and learned from) samples of polyvinyl alcohol, polymethyl methacrylate, polydimethylsiloxane, and gold.
One of the classifiers, for linear discriminant analysis (LDA), stood out as the best performer in terms of providing good quality predictions with its contact models for the materials tested.
The team processed Staphylococcus aureus bacteria to gain previously unseen data in order to demonstrate their framework’s practical viability. With the LDA classifier, they obtained a testing accuracy of 96.8%.
The researchers concluded that the machine learning classifier they developed was a potentially powerful tool for appropriate contact model selection to reduce uncertainty in AFM investigations, even when no prior knowledge is available.
Predicting Electrochemical Impedance Spectra with AI-Enabled AFM
Another article published in 2022, in the journal Scientific Reports, demonstrated a convolutional neural network (CNN) developed to decrease uncertainty in AFM investigations of supported bilayers.
AFM analysis of tethered bilayer membranes (tBLMs), for example, helps scientists understand how pore-forming proteins damage the nanoscale membranes. This data can then be used to predict these objects’ electrochemical impedance spectroscopy (EIS).
EIS is a complicated technique that is used for analyzing bio recognition events that take place at the surface of electrodes. EIS analyzes interfacial properties related to events like antibody-antigen recognition, whole-cell capturing, and substrate enzyme interaction. It has potentially impactful applications in environmental sciences as well as biomedicine.
The recent paper, authored by an interdisciplinary team of computer scientists and biochemists working at Vilnius University, Lithuania, introduced a CNN that predicted tBLMs’ EIS response with a finite element analysis (FEA) modeling technique.
CNN is a deep learning technique and a type of artificial neural network (ANN). FEA is a method for solving differential equations.
The team found that their method predicted electrochemical impedance spectra sufficiently well.
Data, Automation, and Nanoscale Instrumentation
Progress toward smaller, more efficient, and more powerful electronic devices – with nanoelectronics, spintronics, and nanoscale manufacturing techniques all beginning to mature – will continue to generate more data from more accurate and applicable sensors on nanoscale instruments like AFM devices.
When this is combined with another trend in technology – toward more automation – the increase in data sources is compounded. More automation leads to more measurements being taken, more records being noted and shared, and more sets analyzed for trends.
Ultimately, this will continue to lead to reduced uncertainty in measurements for nanoscale investigation instruments.
References and Further Reading
Magar, H.S., R.Y.A. Hassan, and A. Mulchandi (2021). Electrochemical Impedance Spectroscopy (EIS): Principles, Construction, and Biosensing Applications. Sensors. doi.org/10.3390/s21196578.
Nguyen, L.T.P., and B.H. Liu (2022). Machine learning approach for reducing uncertainty in AFM nanomechanical measurements through selection of appropriate contact model. European Journal of Mechanics - A/Solids. https://www.sciencedirect.com/science/article/pii/S0997753822000626?via%3Dihub
Raila, T., et al. (2022). AI-based atomic force microscopy image analysis allows to predict electrochemical impedance spectra of defects in tethered bilayer membranes. Scientific Reports. https://www.nature.com/articles/s41598-022-04853-4.
Disclaimer: The views expressed here are those of the author expressed in their private capacity and do not necessarily represent the views of AZoM.com Limited T/A AZoNetwork the owner and operator of this website. This disclaimer forms part of the Terms and conditions of use of this website.