Postnova Analytics has published an application note that describes a new method to characterize and quantify Multi-Walled Carbon Nanotubes (MWCNTs) in soil samples using Asymmetrical Flow Field-Flow Fractionation coupled with MALS detection (AF4-MALS).
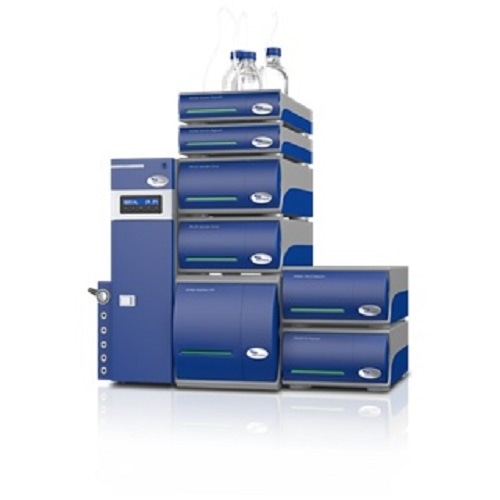
Effective methods to characterise and quantify MWCNTs from soil samples are rare. Quantification, depending on the method used, may be biased by soot particles commonly found in soils and sediments. Differentiating between MWCNTs and soot particles is a big challenge as they are physically and chemically very similar.
The new application note** describes an investigation into how shape could be used as a contrasting parameter for the detection of MWCNTs in pure soot or even soil. In this work the researchers evaluated a shape factor p, derived from AF4-MALS measurements, for its capabilities to detect MWCNTs in such matrices.
The Postnova AF2000 Multiflow platform employed in this research uses a unique crossflow field design in which samples are separated by their dynamic diffusion on the basis of molar mass or particle size. Because of this design and the absence of any stationary phase, field-flow fractionation on the AF2000 Multiflow can be performed without exerting shear forces and stress on the molecules being separated.
The AF2000 can be run with different eluents, wide ranging temperature conditions and is widely proven to be the perfect system for running AF4 experiments. Consequently the AF2000 Multiflow is an ideal platform for the characterization of nanoparticles in complex matrices.
For determination of the hydrodynamic radius (rh) of the MWCNTs in soot and soil samples the researchers used retention time calibration with different certified latex standards. The radius of gyration (rg) was determined using a PN3621 21-angle MALS detector. The application note describes how generation of a shape factor p from rg and rh values over time enables differentiation of MWCNTs and soot particles.
Data is shown that demonstrates how shape factor increment is dependent on the ratio between MWCNTs and soot in a mixture. The researchers conclude that soil particles extracted by the described method are contrasting to MWCNTs in terms of p, allowing specific detection of MWCNTs in these complex matrices. For a copy of this application note please contact Postnova Analytics on +44-1885-475007 or [email protected].
Founded in 1997, Postnova Analytics is the inventor and leading international supplier of Field-Flow Fractionation (FFF) systems for markets including biopharmaceuticals, polymers, materials, nanotechnology and environmental sciences. Leveraging its unique and patented modular FFF - Light Scattering Platform, Postnova has been able to provide high performance solutions to a wide range of applications. Today the company's growing product portfolio includes Flow FFF, Centrifugal FFF, Thermal FFF, Size Exclusion Chromatography (SEC), MALS and DLS Light Scattering. Postnova (www.postnova.com) is located and headquartered in Landsberg am Lech (Germany) and has subsidiaries in the USA, UK and Finland. These offices in conjunction with a highly qualified and trained distribution network provides informed local support to customers worldwide.