A group of researchers recently published a paper in the journal Communications Chemistry that reviewed the role of artificial intelligence (AI) in discovering materials for ion-selective membranes (ISMs).
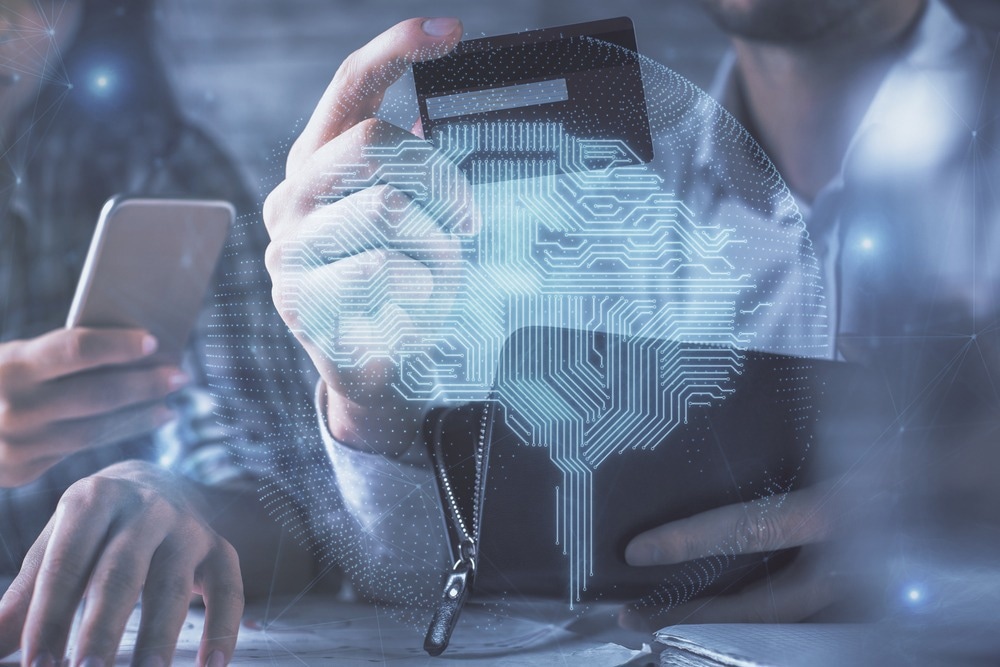
Study: Materials discovery of ion-selective membranes using artificial intelligence. Image Credit: Peshkova/Shutterstock.com
Background
Membrane technology plays a crucial role in several applications, such as fuel cells, batteries, pharmaceuticals, water treatment, and gas separation, owing to its scalability and simplicity. ISMs are used in different processes to recover raw materials from wastewater sources and natural resources.
Significant efforts have been made to increase the production of highly efficient ISMs in a cost-efficient manner. However, the limitations of traditional research and development (R&D) methods, such as time-consuming computer simulations and the high cost of experiments, have hindered the development of novel ISMs.
AI can eliminate the limitations of existing R&D methods and play a critical role in ISM engineering and materials discovery. AI-based output models can use both atomic properties and experimental data for materials discovery and ISMs engineering.
In this study, the authors comprehensively reviewed the role of AI in the materials discovery of ISMs.
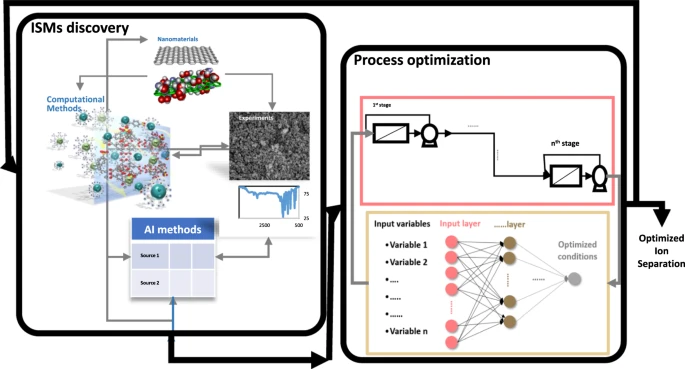
Schematic representation of the application of AI methods for the discovery and design of new ISMs. AI can optimize current ISMs or suggest new ISMs based on computational (or experimental) data. Optimization and discovery of new materials in this method are based on a model that AI builds from related computational (or experimental) datasets. Based on this model, AI can determine the optimal conditions for ion separation (including ISMs type). Image Credit: Maleki, R et al., Communications Chemistry
AI Feature Engineering and Data Preprocessing
The implementation of feature engineering and data preprocessing, including data cleaning and data collection, is necessary to optimize the material predicting models and process parameters and understand the relationships between material specifications to improve the extraction methods of metal ions.
Selecting and mining appropriate data is crucial for building a complete machine learning (ML) dataset. Open source databases, such as the Materials Project for inorganic materials, the Genome Database and Harvard Clean Energy Project for organic solar cell materials and organic molecules, NanoHUB for nanomaterials, and Cambridge structural Databases and Open Quantum Materials for material structural properties, can be used to obtain the sample input data for the ML methods.
Feature Selection of ISM Materials Discovery
Feature selection is an important tool in the AI-based predictive modeling process to identify and remove irrelevant data and select proper variables to enhance the model's accuracy. The appropriate features extracted from the raw datasets using the feature engineering process are referred to as descriptors. Developing a set of meaningful descriptors can improve the predictive model's quality and reliability.
ISM Descriptors
Nanochannel size, nanochannel surface charge, nanochannel morphology, and the driving force are the key descriptors for ISMs. These descriptors can be used to predict the performance of ISMs using the ML approach.
For instance, the nanochannel thickness and size, driving forces, surface charge density, and pH are the important descriptors that must be used to achieve optimized ion selectivity and ion transport rate in polyethylene terephthalate (PET) membranes using AI methods.
Energy-Based Descriptors
Energy-based descriptors implement the interaction behavior between the ions and membranes for a specific framework. These descriptors improve the performance of AI models using density functional theory (DFT) calculations and molecular dynamics (MD) simulations.
Simulations can generate critical characteristics of materials without performing physical experiments. For instance, channel dimension, different water layers, and ionic charge are used as energy descriptors to obtain the ion transport rate and ion selectivity in graphene oxide (GO) membranes using MD simulations.
AI Tools for ISM Modeling for Ion Recovery
AI is considered a suitable approach for material discovery, design, and associated challenges. AI tools can screen large material designs, process the material’s characterization, reduce the simulation prediction time, analyze the characterization dataset, predict complex material system properties, accurately map the multidimensional material synthesis recipes, handle substantial amounts of data, and extract significant intrinsic information and scientific principles from various material designs.
Moreover, the application of suitable ML methods can enable the analysis of all valuable information of a single data point in a considerably lesser time. However, the accuracy of AI models is dependent on the input data quantity and quality. Several AI tools have been used for ISM modeling, including artificial neural network (ANN), support vector machine (SVM), linear regression, and DFT-ML framework.
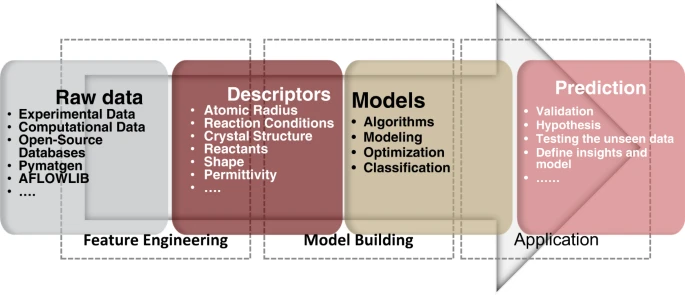
Stages of AI-based ISM discovery. The main descriptors should be selected from the raw datasets. This step is performed using feature engineering. Data screening, determination of input, output and, communicated descriptors are done in this step. After that, AI can train models that can predict the performance of different ISMs. The accuracy and reliability of the model are crucially dependent on feature engineering. Image Credit: Maleki, R et al., Communications Chemistry
ML Capabilities for ISM Analysis
ML and its subclasses can predict and discover new materials with better performance. ML is used for ISM modeling, optimization, computational cost reduction, prediction, and cross-validation. Different ML methods enclose several algorithms, including reinforcement learning algorithms such as the Markov decision process, unsupervised algorithms such as principal component analysis, and supervised algorithms such as classification and regression for the learning process.
ISM Modeling,
Quantitative structure-property relationship (QSPR) modeling was used to accelerate the new ligand discovery in ISMs for lithium ion/sodium ion and magnesium ion/calcium ion selectivities. Similarly, a multilayer perceptrons-based supervised ML algorithm trained using computational fluid dynamics (CFD) simulation and experimental results accelerated the parameter fitting process for understanding the thermodynamic and kinetic parameters of ISMs.
Optimization
Optimization of a membrane process implies a trade-off between the permeability and selectivity to realize a high separation ratio. For instance, the optimal solid polymer electrolyte conductivity for lithium ions was determined using the results from the Bayesian optimization (BO) method coupled with coarse-grained MD simulation.
Computational Cost Reduction
ML-driven atomic and molecular simulations can discover new ISMs quickly. ML techniques assist the MD simulations while the DFT provides predictors and initial screening to reduce the number of simulations.
Prediction
ML techniques can predict different aspects of ISMs, such as membrane lifetime and membrane fouling. For instance, the random forest (RF) algorithm was used to predict the performance of polyvinylidene fluoride/ hydrophilic polyethersulfone (PES)/polysulfone (PSF) membranes in terms of basic materials, membrane fabrication, membrane structure, and operation. The study findings demonstrated that the fabrication of membranes for macromolecules is less challenging compared to membranes for salts.
Cross-Validation
The use of cross-validation methods is necessary to improve the ML model training quality to ensure that these models make accurate predictions and results delivered by these models are reliable. Cross-validation is also used for model parameter optimization. K-fold cross-validation is used extensively for the cross-validation of models.
Limitations of AI in ISM Designing
Lack of Big Data for ISM Modeling
Large educational datasets are extremely significant in AI algorithms as they are utilized to test and train the algorithms. Thus, AI algorithms can make significant errors while making predictions about the properties of new compounds when the input data is insufficient. This issue can be resolved using various publicly available datasets containing properties of other materials while designing and building ISMs.
Lack of Atomistic and Quantum Properties Data
AI algorithms can make erroneous and unrealistic predictions if they do not consider the quantum and atomistic properties of different materials during the design and construction of ISMs.
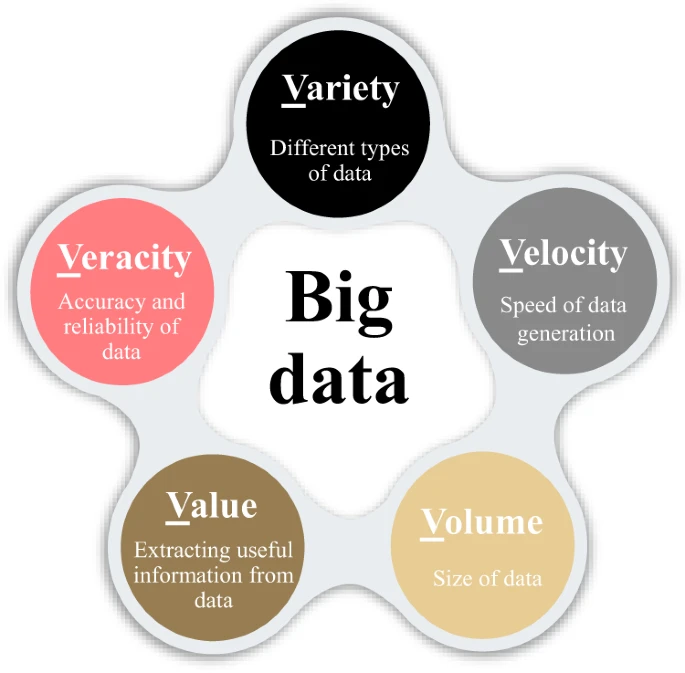
Five main features of big data. These features, are known as the ‘Five V’s’. Big data is described by ‘Five V’s’ including: Value, Volume (size of data), variety (diversity of data), and veracity (Data accuracy and reliability (, and velocity (speed of the data gathering). Image Credit: Maleki, R et al., Communications Chemistry
The Role of Computational Chemistry in AI-Based ISM Engineering
Advanced computational methods, such as DFT calculations and MD simulations, can improve membrane design as they provide atomic property-related molecular information that cannot be availed through AI or experimental tests.
ML methods can be used to improve traditional DFT methods. For instance, the issues associated with conventional DFT methods, including high costs and computational complexity, can be addressed by combining ML with DFT methods. However, the ML-DFT framework has considerably lower accuracy.
The inverse design method can be used to achieve customized components. In this method, the desired properties of the target material are used as input, and the corresponding products or substances with similar properties are explored. Computer vision in the AI system can be used to extract data from membrane images.
In ISMs, big data and high throughput screening must be used for material selection and designing during the fabrication of the target membrane. High throughput screening identifies the material properties and the target material design by performing computational work using a large amount of data, while big data extract a large volume of complex data.
Conclusion
To summarize, AI can play a crucial role in materials discovery and ISMs engineering. AI algorithms can be used to successfully create predictive models with good accuracy based on computational and experimental datasets.
One part of the dataset can be used to train the model, and another part to validate and test the AI-based model. Validated models that typically demonstrate good accuracy can be used for the discovery of ISMs.
Disclaimer: The views expressed here are those of the author expressed in their private capacity and do not necessarily represent the views of AZoM.com Limited T/A AZoNetwork the owner and operator of this website. This disclaimer forms part of the Terms and conditions of use of this website.
Source:
Maleki, R., Shams, S.M., Chellehbari, Y.M. et al. Materials discovery of ion-selective membranes using artificial intelligence. Communications Chemistry 2022. https://www.nature.com/articles/s42004-022-00744-x