Alchemite™ advanced machine learning was used by Domino Printing Sciences to leverage historical data and obtain several novel ink formulations, a number of which were later validated via physical experimentation.
In this case study, Domino was able to:
- Make more efficient use of lab capacity by reducing the number of experiments.
- Optimize formulations by maximizing insight from carefully chosen experiments.
- Reduce time-to-market, and cut experimental timescales from months to minutes.
Challenge
Traditionally, the ink development process requires the formulation and testing of many different potential designs to find an optimum formulation. It should, in theory, be possible to lower this experimental burden by using historical data to guide experimentation along the most productive pathways. And Domino does, indeed have much well-characterized historical data on ink formulations.
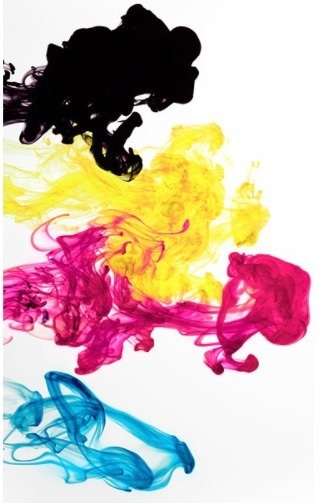
Image Credit: Intellegens Limited
The challenge is that, across the many projects through which this data was assembled, different properties have been measured for different formulations. The resulting data is ‘sparse’ (i.e., has many holes in it) which limits the value of most data analysis techniques — particularly many of those based on machine learning.
Intellegens has built advanced machine learning models that can deal with this data sparsity. The project aimed to apply this Alchemite™ technology to use Domino’s historical data to guide the search for new inks. The objective to reduce the amount of experimentation required was made more urgent by limited lab access during the COVID-19 pandemic.
Solution
Alchemite™, Intellegens’ unique machine learning technology, created a comprehensive model across multiple compositions and ink colors for 28 formulation properties. The flexibility of Alchemite™ facilitated the assignment of different relative weights for each target property.
The model exhibited a high coefficient of determination (accuracy), despite the sparse data, with 75% of the properties modeled either accurately or highly accurately. The model successfully established relationships between design variables (mixture compositions) and formulation properties.
Outcome
Alchemite™ was used to perform two rounds of formulation design. In Round 1, six conventional ink formulations were proposed. Once validated experimentally, the property predictions were found to be highly accurate.
Alchemite™ had identified during model training that the desired removal of one of the components was likely to negatively impact one of the key target properties.
Having recognized this important relationship between variables, Alchemite™ could then be used to find formulation options that mitigated the impact on the target property in Round 2.
A design of experiments campaign, driven by Alchemite™, could be used to ensure that the model has the data that will be most valuable in suggesting future formulations whose target properties can be predicted with a good level of accuracy.
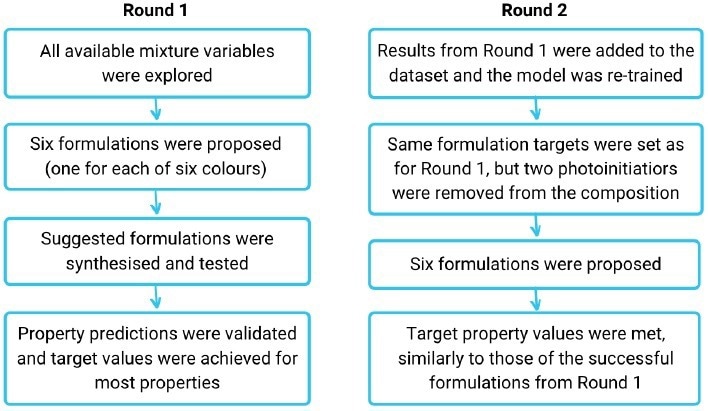
Image Credit: Intellegens Limited
We were impressed with the ability of Alchemite™ to identify novel formulations quickly and accurately. This enabled us to make the most of limited lab resources and continue innovating during the COVID-19 lockdown.”
Dr. Andrew Clifton, Director of Marking Materials and Test Engineering Team, Domino.
Future Opportunities
This case study demonstrated that Alchemite™ machine learning has the potential to revolutionize the way that new inks are designed. It establishes the potential of the system as a solid platform to accelerate the design of any industrial formulation.
There are three key opportunities offered by machine learning, which are:
- Continuous improvement of models (and thus scientists’ understanding of the ink systems) by including more historical data and/or new experimental results from quicker, lower-cost experiments.
- Alchemite™ predictions can be used in a design of experiments campaign to determine the next experimental measurements that will improve the model’s predictions the most for the least effort.
- Alchemite™ can be integrated with Domino’s robotic equipment to automatically formulate and test compositions based on the model’s predictions.
About Domino
Domino was founded in 1978, and since then has established a global reputation for both its worldwide aftermarket products and customer services as well as the development and manufacture of coding, marking, and printing technologies. This continued growth is underpinned by Domino’s unrivalled commitment to product development. Domino was honored in 2017 with its sixth Queen’s Award – widely recognized as the UK’s most prestigious awards for business performance.
About Intellegens
A unique deep learning engine, Alchemite™, has been developed by Intellegens for training neural networks from the sparse and noisy data typical of business challenges and real-world science .
First developed at the University of Cambridge, this technique has been used to develop aerospace alloys, guide the design of new drugs, and design next-generation battery technology.
The tool is now being used to solve a wide range of industrial customer problems, saving time and cost in discovery and development, optimizing products and processes, and ultimately facilitating breakthrough insights.

This information has been sourced, reviewed and adapted from materials provided by Intellegens Limited.
For more information on this source, please visit Intellegens Limited.