3D printing has been making a big impact in the world of technology, providing a cost-effective and efficient way to manufacture a wide range of products. However, like any other technology, it still has room for improvement. One area where 3D printing can be improved is its efficiency.
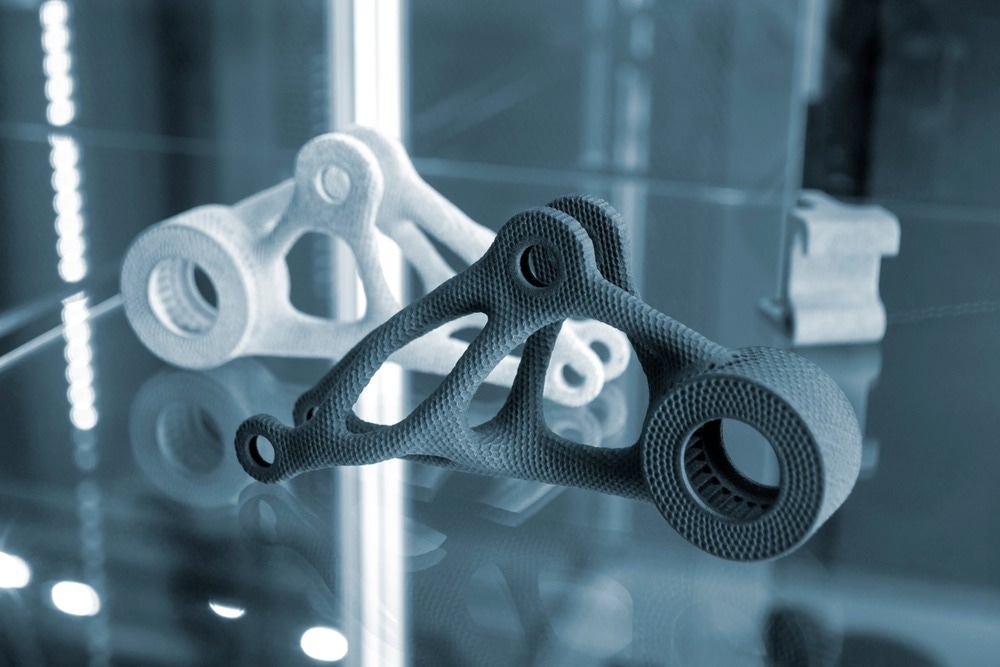
Image Credit: MarinaGrigorivna/Shutterstock.com
A recent breakthrough in the field of Artificial Intelligence provides a promising solution: the Variational Scale-aware Transformer (VST) network. In this article, we will discuss traditional 3D printing and its challenges, the VST network, the solutions it brings, and how it could potentially change 3D manufacturing forever.
The Promise of 3D Printing
The use of traditional subtractive manufacturing methods can be wasteful in terms of energy and materials, leading to a negative impact on the environment.
3D printing or additive manufacturing has been a game-changer in many industries, from manufacturing and architecture to medicine and space exploration. It allows for the production of complex shapes and structures that would be impossible to create using traditional manufacturing methods.
Additive manufacturing is more cost-effective and has the potential to reduce energy consumption and material waste, making it a more sustainable option.
The Limitations of 3D Printing
Despite 3D printing’s many advantages, there is still room for improvement, especially when it comes to efficiency. With the rapid growth in the industry, the need for energy efficiency has become increasingly important.
Another limitation of 3D printing is that it can be slow, especially for larger and more complex objects. This is because the process involves layering materials one at a time, which can take a significant amount of time, especially for intricate designs. In addition, print quality can also be affected by factors such as the temperature of the printing environment, the type of material used, and the speed of the printing process.
The Importance of Machine Learning in 3D Printing
Machine learning is increasingly being used in the 3D printing industry to optimize the design process and improve energy efficiency. By using machine learning algorithms, designers can estimate energy consumption in the design stage and choose the optimal part geometry and process planning for a cleaner production process.
However, traditional machine learning methods also have limitations, such as the need for large annotated datasets and a lack of consideration for dimensional variations in products.
The Variational Scale-aware Transformer (VST) Network: A Solution to 3D Printing Efficiency
The VST network is a new solution that addresses the limitations of traditional machine learning methods. The VST network can precisely predict energy consumption in the additive manufacturing process with modest computational and experimental costs, making it an attractive option for low-budget energy efficiency design in additive manufacturing.
The VST network uses a non-linear regression problem to model the energy consumption prediction task and generates a sliced, layered image as the input, taking into account the layer-wise manufacturing principle in additive manufacturing. It also leverages its unique multi-stage architecture to adapt to increasing part dimension and geometry complexity.
Additionally, the VST network can also adjust the parameters of the 3D printer in real-time to ensure that the final product is of high quality.
In a recent paper published in the Journal of Cleaner Production, a case study was conducted to show how the VST network can be used for low-budget energy efficiency design in additive manufacturing.
The study focused on finding the optimal infill pattern for an aircraft engine mount with the lowest energy consumption. The engine mount contained complex structures and was input into a well-trained VST network to predict its energy consumption.
The engine mount was then printed on a 3D printer to obtain the measured energy consumption values. The results showed that the VST network correctly predicted the energy consumption of the engine mount and that the concentric infill pattern had the lowest energy consumption.
The results confirmed the effectiveness and robustness of the VST for energy efficiency design and demonstrated that the VST could predict energy consumption with a low budget even when the training and testing parts have different dimensional scales and structures.
What are the Benefits of the VST Network
The benefits of using the VST network in 3D printing are numerous. Firstly, it can significantly reduce the time required to complete the process, making it more efficient. Secondly, it can also improve the quality of the final product by ensuring that each layer is laid down with the correct thickness and in the right order.
Thirdly, its ability to predict energy consumption with modest computational and experimental costs makes it an attractive option for low-budget energy efficiency design. Lastly, it can also help to reduce waste by optimizing the use of materials and reducing the need for manual adjustments during the printing process.
Conclusions
The use of machine learning and artificial intelligence in 3D printing is becoming increasingly common, and the VST network is a promising solution that has the potential to revolutionize the industry. By improving energy efficiency in the production process, the 3D printing industry can become even more sustainable and have a positive impact on the environment.
As a machine learning framework, the VST network can be modified to take into account various factors such as the type of material used, the temperature of the printing environment, and the size of the object being printed. It could then predict the optimal scale of each layer in the 3D printing process, which can significantly reduce the time required to complete the process.
This technology has the potential to make 3D printing even more cost-effective and efficient, opening up new possibilities for the growth of the approximately $14 billion industry. The future of 3D printing is looking bright, and the VST network is an important step forward in its development.
More from AZoM: The Future of Technical Ceramics in Manufacturing
References and Further Reading
Goh, G.D., Sing, S.L., Yeong, W.Y. (2021). A review on machine learning in 3D printing: applications, potential, and challenges. Artificial Intelligence Review 54, 63–94. https://doi.org/10.1007/s10462-020-09876-9
Sarah M. (2022) The Untapped Potential of 3D Printing in Advanced Materials [Online], AZoM.com. URL https://www.azom.com/article.aspx?ArticleID=21825 (accessed February 2nd, 2023).
Sarah M. (2022) The Global 3D Printing Market - Growth, Trends, and Applications [Online], AZoM.com. URL https://www.azom.com/article.aspx?ArticleID=22152 ( accessed February 2nd, 2023)
Wang, K., Xu, J., Zhang, S., Tan, J., Qin, J., 2023. Towards low-budget energy efficiency design in additive manufacturing based on variational scale-aware transformer. Journal of Cleaner Production 136168. https://doi.org/10.1016/j.jclepro.2023.13616
Disclaimer: The views expressed here are those of the author expressed in their private capacity and do not necessarily represent the views of AZoM.com Limited T/A AZoNetwork the owner and operator of this website. This disclaimer forms part of the Terms and conditions of use of this website.