This article discusses the use of density functional theory (DFT) and machine learning (ML) to characterize and determine the optoelectronic properties of graphene.
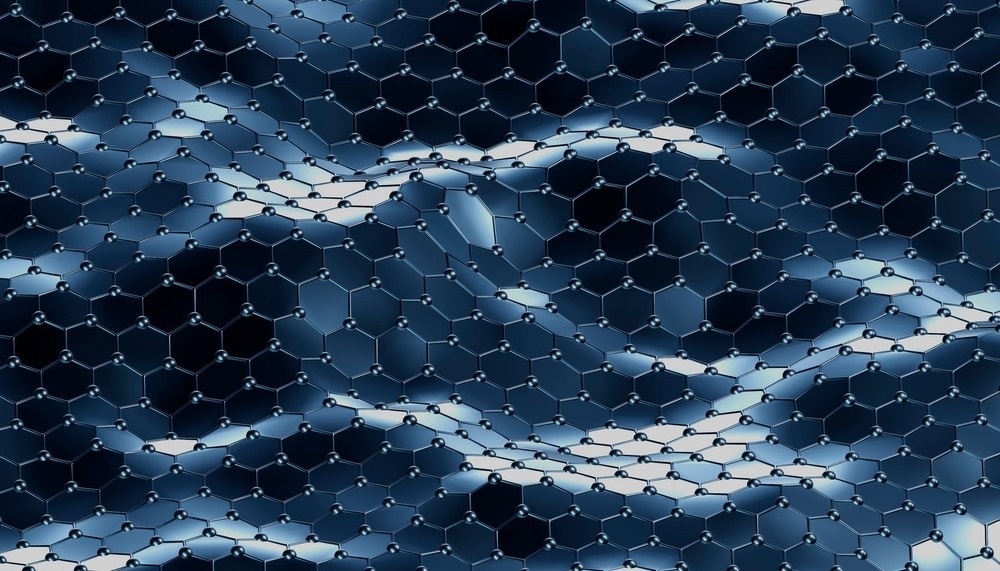
Image Credit: Icruci/Shutterstock.com
Importance of Graphene and its Properties
Due to its high electron mobilities at ambient temperature and peculiar electronic behaviors like the quantum hall effect, which imitates massless transportation and results in high superconductivity, graphene has attracted significant scientific attention for optoelectronic applications. However, due to its zero bandgap and relatively weak structural tunability, its use in optoelectronics is restricted.
To effectively modulate the intrinsic properties of graphene, several reports have established various approaches using stacking configuration, mechanical strain, electric and magnetic fields, and heteroatom chemical doping via DFT methods for next-generation electronics with designer functionalities. Additionally, to maximize the optical qualities for use in electrical devices with optimized stability and performance while expanding the possibilities for unique electronic designs, a sizable band gap must be developed for graphene to reach its full potential.
The graphite oxide's layered structure, which has been demonstrated to possess separate atomic planes, has only been briefly characterized by a few investigations. Moreover, while having a gapless band structure, graphene still has a low quantum efficiency and low total absorption. Many inconsistencies in their findings remain despite the capability of using ab initio research to chemically dope graphene, as stated in the literature, which may be due to the selection of the exchange-correlation functionals, basis set, and incorrect input parameters.
Utility of Density Functional Models for Predicting Properties of Graphene
Due to the highly complex concepts and computer modeling used in the computational density functional theory technique, the materials design process has been significantly enlarged, either independently or in collaboration with an experimental study. One of the most widely used computational methods in condensed matter physics and quantum chemistry, DFT is founded on the idea that electron density can be used as a fundamental variable to solve the problem of total electronic energy and the electronic structure of materials.
Converting the many body systems of interacting electrons into a more straightforward single-particle problem is based on the Hohenberg-Kohn theorem. It provides entirely quantum solutions to the Schrodinger equation. Due to its well-established theories, which have undergone comprehensive validation, and the growing number of papers in the literature, DFT is well-acknowledged for computing the electrical structure of graphene. This recognition has considerably benefited from its acceptance in the scientific community.
Recent Developments
In a study recently published in the journal Heliyon, researchers provided a thorough summary of numerous reports that have been published using DFT and newly developed ML techniques to investigate the electrical and optical properties of graphene as well as heteroatom-doped graphene. The various density functional theory models and the advantages and limitations of the machine learning potentials used in the enhancement of graphene prediction models were clarified.
One of the studies reported that due to their high electron mobilities and tunable band structures, graphene/hBN heterostructures could be used as a potential foundation for next-generation optoelectronics. Graphene-based heterostructures are tested in photodetectors, superfast electronics, and optical communication satellites. It was observed that reliable and portable DFT and ML models are needed to identify the strong anisotropic tensor in the overall optical response of graphene solid anisotropic to make them more practical for optical systems.
Another study reported that graphene could naturally exhibit superconducting and nonconducting behavior by combining two graphene layers into a superlattice and rotating them at a magic angle of 1.1 degrees. To build and manufacture nano-superconducting quantum optical devices and nano-superconducting transistors, it would be crucial in the near future to use traditional DFT and ML-DFT to precisely anticipate the atypical superconducting and insulating characteristics of huge and complex graphene.
Moreover, graphene-based coatings with distinct electronic properties might use electricity to generate thermal energy and transport it to the external surface. They could also improve conductivity and electromagnetic shielding to guard against lightning strikes. Therefore, the optimization of the DFT and ML-DFT frameworks in future studies to modify graphene's structural and electronic properties is critical for designing and manufacturing these graphene-based devices.
The authors explained that to fully realize graphene's potential, future research should focus on several gray areas, including its thermal properties, the modeling of graphene heterostructures, its superconducting behavior, and the optimization of the DFT models. They also observed that the kinds of dopant atoms, their proportions, and the doping sites play a significant role in these changes in graphene's characteristics. Also, depending on the number of valence electrons in the dopant atom, these heteroatoms could be electron acceptor (p-type) or electron donor (n-type) systems when substituted into graphene.
In conclusion, the study examined the electrical and optical characteristics of the chemically doped graphene systems utilizing the DFT approach, focusing on their possibilities, doping type, and known gaps in the literature. It was determined that these gaps could be linked to incorrect parameter inputs, basis sets, and exchange-correlation functional selections, all of which impact how well the model predicts. The literature thoroughly lists the bond lengths, band gaps, and formation energies of several doped graphene systems that affect their thermodynamic stability.
Limitations of DFT and ML in Graphene Research
Graphene's electrical structure at absolute temperature and optical excitations are profoundly understood by density functional theory and the newly developed ML-DFT technique, yet specific unanswered questions still exist.
As a result of imbalances in delocalization, static correlation errors, and incorrect parameter selection, the band gap calculation of graphene structures is a contentious topic in the literature. Different exchange-correlation functionals used in ab initio studies predict varying values. This makes the selection of the XC functional system dependent. Furthermore, the use of ML models to forecast the electrical characteristics of graphene requires more training data for these models.
XC functionals utilized in the ab initio research cannot represent long-range electron correlations for the characteristics of graphene. Hence, one of the most significant issues with first principles computations is the absence of dispersion forces. Additionally, as the non-local ML potential requires system-specific training, a significant amount of training data is needed to define the non-local functional with various parameters adequately.
The effects of the vibrations from DFT calculations are not described for both the band structure and spectroscopy level in graphene. For optoelectronic applications that commonly depend on temperature, this reduces the role of polaronic effects, especially the impact of temperature. The proper modeling of graphene-based heterostructures, including the graphene-hBN layer and graphene silicon heterostructures, has some constraints. This results from their systems' complexity, brought about by the interaction of numerous parameters.
Due to the limitations on the modeling of these heterostructures' interactions and physical characteristics, there are inconsistencies in the calculations of their electrical and optical properties. Furthermore, ML potentials need a considerable time series of data to predict these accurately.
In conclusion, DFT predictions of long-range electron correlations of graphene properties have shown significant potential for speeding up and enhancing accuracy, similar to earlier advancements in machine learning. However, there has yet to be any experimental validation of the ML-DFT results. Therefore, it is envisaged that combining these two approaches would be essential for bridging the knowledge gaps in graphene research and ensuring that it achieves its full potential.
More from AZoM: CT Scans Allow for Easier Security Screenings at UK Airports
References and Further Reading
Olatomiwa, A. L., et al. (2023). Recent advances in density functional theory approach for optoelectronics properties of graphene. Heliyon, e14279. https://doi.org/10.1016/j.heliyon.2023.e14279
Naseri, M., et al. (2023). Two-Dimensional δ-Be2C with Hepta-Coordinated Carbons: A Highly Stable Direct-Band-Gap Semiconductor Predicted by First-Principles Calculations. Journal of Physical Chemistry C, 127(3), 1687-1696. https://doi.org/10.1021/acs.jpcc.2c07834
Cassiano, T. A., et al. (2022). Charge transport in cove-type graphene nanoribbons: The role of quasiparticles. Synthetic Metals, 287, 117056.
https://doi.org/10.1016/j.synthmet.2022.117056
Disclaimer: The views expressed here are those of the author expressed in their private capacity and do not necessarily represent the views of AZoM.com Limited T/A AZoNetwork the owner and operator of this website. This disclaimer forms part of the Terms and conditions of use of this website.