Recent developments in informatics, particularly data sciences, machine learning, and language models, have significantly increased its implementation in other fields. Materials scientists readily utilize these tools for efficient and fast materials design, discovery, and prediction of properties.
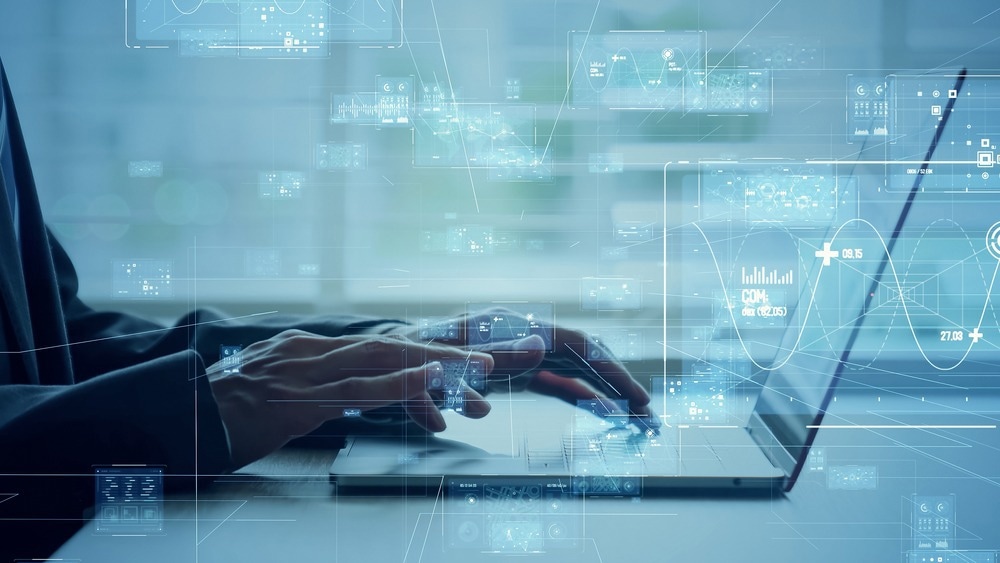
Image Credit: metamorworks/Shutterstock.com
Data-driven science represents a transformative paradigm in materials science. Both data-driven materials science and informatics encompass systematic knowledge extraction from materials datasets. This approach distinguishes itself from conventional materials research by prioritizing extensive data processing and enhancing the automation of information retrieval.
Data-driven Design of Metamaterials
Metamaterials are engineered to exhibit unique material properties beyond those found in nature, involving the intricate design of multiscale structures within an infinite-dimensional topological design space.
The development of data-driven methods has addressed these complexities by facilitating the high-throughput prediction of properties, accelerating design space exploration and optimization, and providing swift solutions to challenging inverse design problems.
According to a recent article published in Advanced Materials, the design of data-driven metamaterials generally comprises three modules: data acquisition, machine learning (ML)-based metamaterial unit cell design, and multiscale design.
The data acquisition module involves efficiently obtaining a precomputed dataset of unit cells using data frameworks. Following this, ML algorithms are employed to gain insights from data and facilitate unit cell designs. Finally, in the multiscale design module, the unit-cell database and the ML models are utilized to develop efficient designs at the system level.
Practical applications may involve integrating all these modules into a unified framework or selectively focusing on specific modules based on the design requirements.
In the design of unit-cell-level metamaterials, the focus is typically on material properties. Data-driven models excel in learning intricate structure-property relationships, serving as surrogates for time-consuming simulations or experiments.
These models accelerate the design process by enabling high-throughput property evaluation, particularly when combined with downstream design space exploration methods like sampling, screening, and optimization.
An Overview of 2D Materials Small Data Informatics
Applying data-scientific approaches to materials informatics (MI) has garnered significant interest in data-driven research. However, the availability of sufficient big data is not always guaranteed for these data-oriented models. Utilizing a data-scientific approach with limited data can accelerate research endeavors, minimizing energy, resource, and cost consumption.
Findings from an article published in the Bulletin of the Chemical Society of Japan reveal that sparse modeling, a specific type of ML data-driven modeling, is extensively applied for 2D materials. Feature selection involves choosing a limited set of critical variables related to the target variable from a dataset containing numerous explanatory variables.
The research team utilized a small data-driven model based on experimental data to predict and enhance the yield of transition-metal-oxide nanosheets. Traditionally, these nanosheets are produced by exfoliating precursor layered materials; however, achieving high yield and controlling lateral size can be challenging with this approach.
Applying materials informatics to small data helped transform uncontrolled processes into controlled outcomes. The team collected experimental yield data, consisting of 104 observations, for the training data set. After thorough screening, this was reduced to 60 data points. Utilizing sparse modeling, potential descriptors were extracted. Then, leveraging expert chemical insights, the most critical descriptors were selected.
The prediction model, developed from this training dataset, was then employed to predict yields under unknown experimental conditions for the high-yield synthesis of 2D materials.
The model achieved an accuracy rate of up to 85 %, providing valuable insights for enhancing both process efficiency and sheet control. While it did not reach extremely high accuracy, the model's ability to provide reasonable estimations proves beneficial in significantly reducing the number of experiments.
The straightforward prediction models can be adapted for the controlled synthesis of various 2D materials by incorporating additional training data.
How are Deep Language Models Transforming Predictive Materials Sciences?
In materials science, the significance of artificial intelligence (AI) and informatics has grown due to its ability to predict material properties. The effectiveness of AI and informatics models heavily relies on the availability of sufficient and high-quality data.
While numerous materials databases are available, researchers often need to extract relevant data from a multitude of studies and databases. Here, text processing techniques emerge as efficient alternatives to manual data extraction.
Natural Language Processing (NLP), as highlighted by an article in APL Machine Learning, has demonstrated its ability to effectively encode materials science knowledge found in published literature and transform unstructured raw text into structured database entries. This enables programmatic querying and enhances the extraction of valuable insights from the existing literature.
Language models can also contribute to learning valuable information from images. This proves particularly beneficial for data labeling in experiments, where the interpretive capabilities of language models extend to visual data, aiding in the extraction of meaningful insights from images.
Through integration into AI frameworks, language models are enhancing the prediction of material structures and properties. For example, recurrent neural networks have exhibited remarkable capabilities in addressing complex challenges such as protein folding, material property prediction, and conducting failure analysis of complex nonlinear architected materials.
Recent breakthroughs have led to the development of an AI-based multiscale model featuring convolutional Long Short-Term Memory (LSTM) networks for predicting fracture patterns in crystalline solids using molecular simulations. This innovative approach has demonstrated excellent agreement with computed fracture patterns. It also accurately predicts fracture toughness values.
The introduction of a data-driven model capable of predicting the brittle fracture of polycrystalline graphene under tensile loading with remarkable accuracy showcases the potential of language models in enhancing predictive performance in materials science.
FEANN: A Novel Data-driven Multiscale Approach for Materials
A research article published in Computational Mechanics introduces a novel data-driven multiscale framework named FEANN. This framework is characterized by two principal components: the utilization of physics-constrained artificial neural networks (ANNs) as surrogate models at the macroscopic level and an autonomous data mining process. This innovative approach enables the efficient simulation of materials featuring intricate microstructures, unveiling overall anisotropic and nonlinear behavior on the macroscale.
The model has been implemented for the solution of three illustrative examples: a cuboid under tension, a torsional sample, and the Cook membrane. In these cases, the macroscopic bodies are composed of a fiber-reinforced composite, showcasing the highly nonlinear behavior of individual phases.
The integration of physical knowledge into the ANN-based surrogate model significantly reduces the need for numerous computationally demanding Representative Volume Element (RVE) simulations to solve macroscopic problems.
The data-driven approach effectively tackles complex multiscale problems at finite strains, exhibiting universality across macroscopic geometries and boundary conditions due to the implemented unsupervised data mining.
Future extensions may involve incorporating additional material symmetry groups by integrating appropriate invariant sets into the implementation. Despite potential extensions, the current model stands out for its high effectiveness and efficiency.
The emergence of informatics strategies within materials science, driven in part by initiatives like the Materials Genome Initiative, is gaining momentum. This shift is influenced by both advancements in algorithms and the notable successes of data-driven approaches in various domains.
The incorporation of machine learning in materials science relies on the availability of past reliable data, yet the field is advancing at a steady pace. Algorithms like multi-fidelity learning, already commonplace in engineering disciplines, are making inroads into materials informatics.
Informatics is undoubtedly revolutionizing materials science, empowering the development of efficient and dependable methodologies for designing and modeling novel materials.
More from AZoM: Applications of Artificial Intelligence in Materials Science
References and Further Reading
Lee, D., et al. (2023). Data‐Driven Design for Metamaterials and Multiscale Systems: A Review. Advanced Materials. doi.org/10.1002/adma.202305254.
Yuya, O., et al. (2022). Materials Informatics for 2D Materials Combined with Sparse Modeling and Chemical Perspective: Toward Small-Data-Driven Chemistry and Materials Science. Bulletin of the Chemical Society of Japan. doi.org/10.1246/bcsj.20210253.
Hu, Y., Buehler, MJ. (2023). Deep language models for interpretative and predictive materials science. APL Machine Learning. doi.org/10.1063/5.0134317.
Kalina, K., et al. (2023). FE ANN: An efficient data-driven multiscale approach based on physics-constrained neural networks and automated data mining. Computational Mechanics. doi.org/10.1007/s00466-022-02260-0.
Hitachi High-Tech. (2023). What is Materials Informatics? [Online] Hitachi High-Tech. Available on: https://www.hitachi-hightech.com/global/en/products/ict-solution/randd/ci/or-mi01.html. [Accessed: 22 February 2024].
Zhang, N. (2021). Materials Informatics: A Data-based Approach to Materials Discovery. [Online] Mewburn Ellis. Available on: https://www.mewburn.com/news-insights/materials-informatics-a-data-based-approach-to-materials-discovery [Accessed: 21 February 2024].
Disclaimer: The views expressed here are those of the author expressed in their private capacity and do not necessarily represent the views of AZoM.com Limited T/A AZoNetwork the owner and operator of this website. This disclaimer forms part of the Terms and conditions of use of this website.