By Taha KhanReviewed by Lexie CornerUpdated on Sep 13 2024
Traditional design strategies for synthetic polymers and organic molecules are experiment-based, guided by experience and intuition, and driven by application requirements. However, with the growing demand for new materials and the vast number of existing organic molecules, these methods face significant challenges.
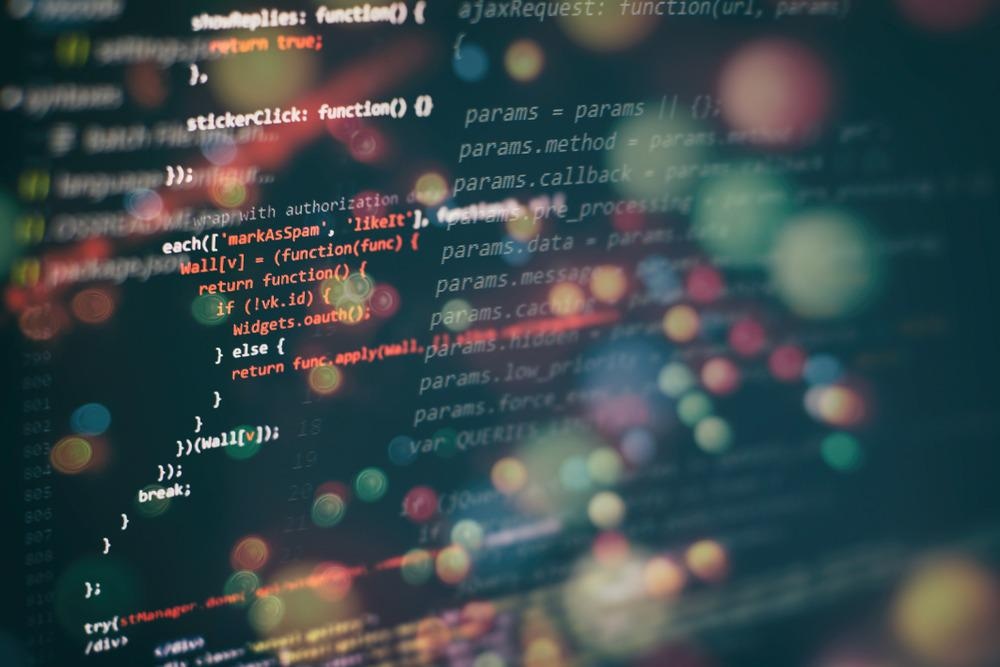
Image Credit: Zakharchuk/Shutterstock.com
With rapid advances in high-throughput computing, machine learning (ML), and artificial intelligence (AI) applications, polymer informatics is emerging as a promising tool to ensure breakthrough discoveries in polymer science.1, 2
Polymers are one of the most ubiquitous classes of materials in modern society. Their applications range from packaging, textiles, and consumer goods manufacturing to medicine, construction, and transportation. A polymer material consists of many repeating units, called monomers, assembled in long molecular chains.
These polymer chains can form different structures, resulting in polymer materials with highly diverse physical and chemical properties. Some polymer chains can include more than one type of monomer, thus creating even more complex topological structures on different length scales.
Traditional research methods based on intuition and trial and error have already demonstrated the significant potential of polymer materials. However, due to the vast macromolecular structural variety of polymers, new approaches are needed to identify and develop novel applications.
The emerging field of polymer informatics addresses this challenge by leveraging AI- and ML-based methods to enable data- and information-driven research.
How Can Polymer Informatics Help Polymer Research?
Building reliable empirical models based on fundamental physical and chemical properties can facilitate the prediction of polymer characteristics. Polymer Genome is an ML-based tool that can rapidly predict various polymer properties using models trained on polymer databases, experimental data, or first-principles computations.
In polymer informatics, a large pool of chemically or synthetically feasible polymers can be screened for potential candidates by applying predictive models relevant to the desired material properties.3, 4
Polymer synthesis is often costly and labor-intensive, making experimental design algorithms ideal for minimizing the number of experiments needed to achieve a design goal. These algorithms leverage existing data to identify candidates most likely to meet the desired specifications.
Key Developments in the Polymer Industry Using AI and ML
Polymer materials must meet specific property requirements to be suitable for particular applications. With the availability of extensive datasets, modern ML techniques can significantly accelerate the discovery of novel polymers.
Many research groups are adopting data-driven approaches to polymer design, enhancing productivity and enabling the development of new functional polymers to meet the growing demands of the expanding polymer materials market.
Polymer Dielectrics for Energy Storage
Polymer informatics can significantly accelerate the discovery of high-performance energy storage capacitors. By defining multiple desired properties, such as high glass transition temperature and dielectric strength, and combining these with computational and data-driven ML strategies, researchers have developed novel dielectric films that demonstrate excellent thermal stability at extreme temperatures.
For instance, a 2024 study used AI to discover high-temperature dielectrics for energy storage applications. The study identified new materials within the polynorbornene and polyimide families by integrating AI with established polymer chemistry and molecular engineering.5
These materials exhibit both high thermal stability and high energy density across a broad temperature range. One such polymer, PONB-2Me5Cl, achieves an energy density of 8.3 J cc-1 at 200°C, outperforming existing commercial alternatives.5
Polymer Electrolytes for Li-Ion Batteries
AI-based property prediction models and design algorithms have also proven successful in the development of safer solid polymer electrolyte (SPE) materials for rechargeable Li-ion batteries.
In a 2023 study, researchers developed an ML model to accelerate the discovery of high-ionic-conductivity SPEs, which are crucial for improving the performance and safety of lithium-ion batteries.6
This model incorporated the Arrhenius equation within a message-passing neural network and was trained on a comprehensive dataset of SPE ionic conductivity from numerous experimental publications.
This chemistry-informed approach significantly enhanced prediction accuracy by accounting for temperature-dependent processes. The study screened over 20,000 potential SPE formulations, identifying promising candidates, and demonstrated the model's capability to predict ionic conductivity across different polymers and salts.6
Conducting Polymers for Electronic Applications
Although most polymers are insulators, a specific class of polymers known as conjugated polymers is extensively used in electronic applications due to their intrinsic conductivity.
In a 2024 study, researchers utilized ML to enhance the discovery of doped conjugated polymers with optimized electrical conductivity. They developed an ML-based classification model that accurately identifies samples with conductivities ranging from ~25 to 100 S/cm, achieving a 100 % accuracy rate.
For highly conductive samples, a regression model was employed to predict conductivities, yielding a remarkable R² value of 0.984. This ML-assisted approach significantly accelerates the measurement process, improving efficiency by up to 89 % compared to traditional methods.7
Moreover, the study's use of spectral data for model training has enhanced the understanding of the relationship between absorbance spectra and conductivity, addressing common challenges in material science with improved explainability and reduced reliance on manual expertise.7
Polymer Membranes for Fluid Separation
Polymers with high surface area show significant potential as membrane materials for fluid separation applications. Identifying polymer candidates with high intrinsic microporosity and permeability for specific fluids can be challenging, but a 2023 study offered a promising solution.
Researchers developed a framework integrating ML algorithms with mass transport simulations to predict permeation behaviors in polymer membranes. This approach combines physics-informed ML models with data on the diffusion and sorption properties of organic solvents.8
Using these models, the researchers could accurately forecast the separation of complex mixtures, such as crude oils, with high precision. This method facilitates rapid screening of polymer membranes while significantly reducing the need for extensive physical testing.8
Discovery of Novel Biodegradable Polymers
Traditionally, understanding the relationship between polymer chemical structures and their biodegradability was limited by slow and expensive testing methods. Researchers have advanced the field of biodegradable polymers by applying ML to a comprehensive dataset of polymers.
In a 2023 study, high-throughput techniques were developed to synthesize and test a diverse library of 642 polyesters and polycarbonates. The researchers utilized a rapid clear-zone assay for biodegradation testing, which, combined with ML algorithms, enabled the development of predictive models for polymer biodegradability.9
This approach allowed them to build predictive models with over 82 % accuracy, identifying key structural features that influence biodegradability, such as aliphatic chain length and ether groups. The methodology accelerates testing and provides valuable insights into structure-property relationships, facilitating the discovery of novel biodegradable polymers.9
Polymer Informatics: Challenges and Future Prospects
The availability of large, open databases is crucial for ML applications in polymer informatics. However, creating these databases presents several challenges. Inconsistencies in the history of polymer science, along with the lack of data sharing due to proprietary research, hinder the development of a comprehensive polymer database. Additionally, encoding data related to the hierarchical structure of polymers for ML purposes can be complex.
Despite these obstacles, academic and industrial research groups worldwide are making strides toward establishing fully data-driven research and development processes for polymers. Future advancements will likely include more sophisticated AI techniques and the integration of larger, more diverse datasets, improving the accuracy and reliability of polymer property predictions. This progress will facilitate the rapid discovery of novel polymers with tailored characteristics for specific applications.
Discover More: Using AI-Powered Optical Inspection to Detect Nanoscale PCB Defects
References and Further Reading
- Chen, L., et al. (2021). Polymer informatics: Current status and critical next steps. Materials Science and Engineering: R: Reports. https://doi.org/10.1016/j.mser.2020.100595
- Kuenneth, C., et al. (2021). Polymer informatics with multi-task learning. Patterns. https://doi.org/10.1016/j.patter.2021.100238
- Tran, D., et al. (2020). Machine-learning predictions of polymer properties with Polymer Genome. Journal of Applied Physics. https://doi.org/10.1063/5.0023759
- Kim, C., et al. (2018). Polymer genome: a data-powered polymer informatics platform for property predictions. The Journal of Physical Chemistry C. https://doi.org/10.1021/acs.jpcc.8b02913
- Gurnani, R., et al. (2024). AI-assisted discovery of high-temperature dielectrics for energy storage. Nature communications. https://doi.org/10.1038/s41467-024-50413-x
- Bradford, G., et al. (2023). Chemistry-informed machine learning for polymer electrolyte discovery. ACS Central Science. https://doi.org/10.1021/acscentsci.2c01123
- Yoon, JW., et al. (2023). Explainable machine learning to enable high-throughput electrical conductivity optimization of doped conjugated polymers. arXiv preprint arXiv. https://doi.org/10.48550/arXiv.2308.04103
- Lee, YJ., et al. (2023). Data-driven predictions of complex organic mixture permeation in polymer membranes. Nature Communications. https://doi.org/10.1038/s41467-023-40257-2
- Fransen, KA., et al. (2023). High-throughput experimentation for discovery of biodegradable polyesters. Proceedings of the National Academy of Sciences. https://doi.org/10.1073/pnas.2220021120
Disclaimer: The views expressed here are those of the author expressed in their private capacity and do not necessarily represent the views of AZoM.com Limited T/A AZoNetwork the owner and operator of this website. This disclaimer forms part of the Terms and conditions of use of this website.