By Surbhi JainReviewed by Susha Cheriyedath, M.Sc.May 3 2022
In an article recently published in the open-access journal Materials, researchers discussed the design of a real-time automobile corrosion detection and quantification protocol.
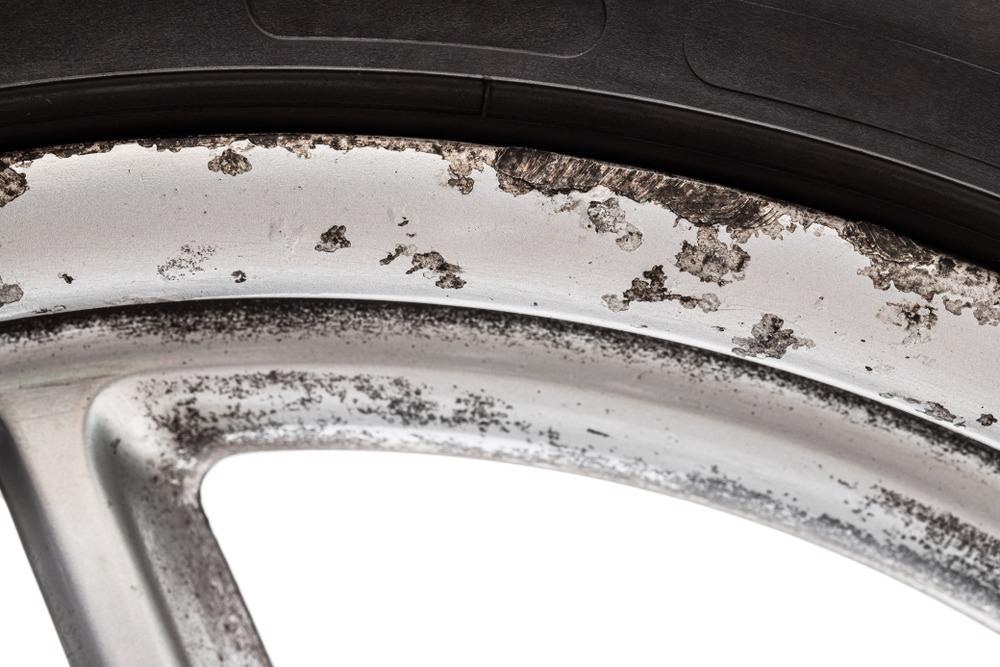
Study: Design of a Real-Time Corrosion Detection and Quantification Protocol for Automobiles. Image Credit: Elena Zajchikova/Shutterstock.com
Background
Gravel, snow, de-icing salts, and humid weather are all part of the driving environment for automobiles. Although there have been considerable advances and a higher knowledge of issues connected to vehicle performance, emissions, and safety, corrosion's effects are still dangerous and can cause both short- and long-term problems. As a result, it's critical to think about car maintenance.
There is, however, a slew of after-market corrosion prevention and treatment products on the market right now. There is no single approach for measuring corrosion that is utilized consistently throughout the vehicle industry at the consumer/aftermarket level. Visual inspection, the simplest and most popular way of identifying rust, is typically used by a technician or the vehicle's owner at an automobile facility. However, visual inspection is prone to human error.
As a result, if at all possible, machines should be used to complete the task. Texture analysis is a prominent, attribute-based method for detecting corrosion and monitoring corrosion in non-destructive constructions. Despite this, no single texture analysis approach has been proven to detect all different types of corrosion patterns and colors.
About the Study
In this study, the authors presented an automated approach for the identification and surface analysis of the rust on vehicles, as well as the assessment of its severity. To study corrosion and promote future in-field use, an automated corrosion quantification and detection method was described.
The researchers analyzed the response time and preciseness of the results produced by the proposed approach. The process for detecting and quantifying vehicle corrosion was also described. In this study, 369 automobiles were sampled to produce a dataset. The dataset was then used to validate and test the approach.
The team referred to all pixels which contributed to non-rusty parts of the vehicle, such as the fiber-made and wheel parts, the equipment of the garage facility such as the hoist, and items present at the facility. Every component of the dataset was grouped into sub-divisions in order to measure the corrosion on each car, such as treated or untreated, as well as vehicle age and model. From a distance of 1.0 m to 1.5 m, the photographs were taken with two cameras: a Nikon Coolpix P7000 and a Canon PowerShot G5X. The photographs were captured using a yellow-colored custom-made "T-scale" ruler to manually evaluate the size of the car element.
Observations
The method was shown to be around 98% efficient with medium noise level photos and 93 to 96% efficient with very high noise level images. The gap between the efficiency of the masked and unmasked datasets was significant. The results also showed that simply focusing on the damaged area in the photos could enhance corrosion detection accuracy.
According to the findings, the proposed method had the ability to detect common car corrosion types such as blisters, cracks, perforations, and surface rust. The results demonstrated that the estimate was 96% accurate among the 369 automobiles tested, with small-scale noise variation.
It was also observed that when light variations were exceedingly significant and image quality was severely compromised, the calculation could not produce effective results. This could be due to the fact that the light reflectance value (LRV) was very close to 100% and the optical sensor had a hard time distinguishing between color tones. The highest feasible accuracy in this scenario was found to be about 93%. The procedure was more efficient when the visual content was more focused on the corroded area, according to the results.
Conclusions
In conclusion, this study elucidated that the proposed method is low-cost, very effective, and computationally simple. The focus was on the detection of vehicle deterioration. Color resolution variance, illuminance heterogeneity, a dirty car body, unfocused areas, and elements of the surroundings in which the photographs were recorded were all present in the raw dataset images. Perceptions and findings suggested that the approach detects corrosion in metals such as aluminum, iron, and steel successfully, though not perfectly.
The authors attempted to improve the efficiency by automating the masking of unwanted pixels in the image. They emphasized that this will make life easier for in-field technicians/users and reduce concerns like lack of focus and poor image quality.
They mentioned that the proposed technology might be transferred to a variety of modern devices, such as smartphones, to reduce errors, assess corrosion, and increase measuring accuracy. They believe that the method's low implementation cost and great dependability contribute to its simplicity of use in the field, making it more accessible to automotive specialists who want to diagnose and monitor corrosion levels without human error. They also stated that the algorithm holds the potential to be a practical tool for the detection of corrosion and further applications in the automotive industry.
More from AZoM: Laser Ablation for Elemental Analysis of Forensic Samples
Disclaimer: The views expressed here are those of the author expressed in their private capacity and do not necessarily represent the views of AZoM.com Limited T/A AZoNetwork the owner and operator of this website. This disclaimer forms part of the Terms and conditions of use of this website.
Source:
Bahadoran, A., Liu, Q., Ramakrishna, S., et al. Design of a Real-Time Corrosion Detection and Quantification Protocol for Automobiles. Materials 15(9) 3211 (2022). https://www.mdpi.com/1996-1073/15/9/3222