Warp deformation is a common issue in 3D printing. A new paper, currently in the pre-proof stages in the journal Additive Manufacturing, has presented a novel hybrid approach to identify and counteract this problem, providing a new way to improve 3D printed product quality.
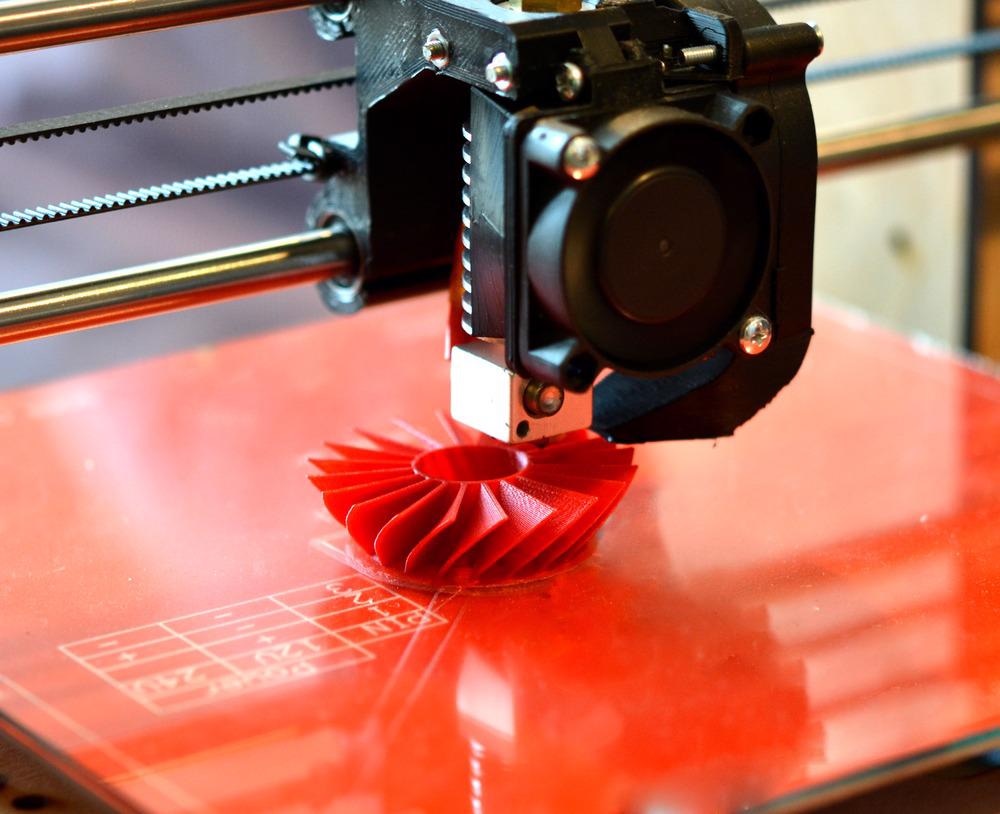
Study: Automated recognition and correction of warp deformation in extrusion additive manufacturing. Image Credit: MarinaGrigorivna/Shutterstock.com
What is Warp Deformation?
In additive manufacturing, the accurate production of materials is crucial to ensure their functionality. Warp deformation is a common error in 3D printing processes, caused by residual internal stresses as the material cools. These issues are only visible after time has passed since initial extrusion, making them difficult to predict or counteract.
These errors limit the functionality and quality of printed devices and components. This is particularly problematic in structural components and safety-critical products. Warping occurs in several additive printing methodologies such as material extrusion, selective laser sintering, and solvent-based extrusion. Related contractile mechanisms govern the induction of warping in printed materials.
Non-uniform cooling rates after material deposition generate residual thermal stresses in additive manufacturing processes. This is a typical cause of warping. Stresses increase as more layers are deposited, leading to distortion of parts, especially when there is insufficient adhesion between parts and build plates that cannot overcome the induced pulling force of the residual stresses. Warping is a continuous process that typically starts at the material’s corners and eventually causes the part to peel away from the base plate.
Most additive manufacturing processes are open loop, and machines cannot accurately detect the current printing state. This makes these processes vulnerable to warping. Successful printing requires multiple iterations of a printed part, which can lead to wasted time, materials, and energy.
Another problem is that many commonly used high-performance thermoplastics require elevated temperatures, making them more prone to warping. Experienced human operators are needed to assess the error, reset parameters, and re-start the process. Automated processes have been researched in recent years to overcome these issues and improve the accuracy and reliability of error-free printing.
Automatic Error Detection Processes
Automatic error detection processes include current, acoustic, and inertial sensors. These methods have been proven to be highly accurate. Other processes have taken advantage of neural networks and vision-based sensing techniques. Vision-based techniques offer more in-depth information and can identify a broader set of errors in additive manufacturing processes. Computer vision and deep learning approaches have both been explored in vision-based error detection methodologies.
Deep learning offers a more generalizable approach to error detection. Additionally, they possess improved robustness under different conditions than conventional detectors, and recent advances in neural networks have vastly improved the image classification capabilities of deep learning techniques. However, many processes merely detect warping and do not prevent it or correct it when it occurs, and there has been little work using cutting-edge vision techniques for in situ warp detection.
Detecting, preventing, and correcting warping errors are highly challenging, particular during the printing process itself. Moreover, warping can be impacted by several factors such as the number of layers, model size, chamber and bed temperatures, the linear shrink rate of materials, and stacking selection length.
The Study
The researchers behind the study have proposed a novel hybrid system for warp detection, prevention, and correction during printing. The system incorporates computer vision, expert heuristics, and deep learning technologies. A convolutional neural network was trained to recognize warp in real-time using diverse labeled images of real warp errors. The system introduced in the paper is highly sophisticated and improves upon conventional warp detection approaches.
A novel characteristic of the approach presented in the research is the ability to extract a suite of metrics from available detection images and estimate warp deformity severity. Metrics were then combined with expert heuristics to slow warp growth during printing and limit its severity. This combined deep neural network/heuristics approach reduces or eliminates the instances of warping in future prints by automatically correcting slicing and printing parameters.
One of the main advantages of this novel hybrid system is the ability to correct warping in situ and dramatically reduce its severity. This improves the functionality of otherwise unusable parts and saves time, material, and energy. Additionally, maximum optimization of parameters can be achieved without causing warp errors to occur.
The paper has demonstrated the first automated warp detection and correction system. The system can be generalized to hardware, materials, unseen geometries, and colors without requiring manual setup or pre-processing steps. Applying the presented methodology to other additive manufacturing processes and functional materials is an exciting research direction, and this process could be used in the simultaneous operation of multiple printers.
Future research opportunities have been identified in the paper, including increasing image sampling rate and metrics sensitivity in software and developing a more in-depth understanding of the influence of different parameters on warp errors. Furthermore, there is scope for creating a full PID controller using the system. Overall, the paper has demonstrated a highly interesting and beneficial automated error detection system that will improve the efficiency and reliability of 3D printing.
More from AZoM: What Multi-Analytical Techniques are Used to Assess Paintings?
Further Reading
Brion, D.A.J, Shen, M & Pattinson, S.W (2022) Automated recognition and correction of warp deformation in extrusion additive manufacturing Additive Manufacturing 102838 [online, pre-proof] sciencedirect.com. Available at: https://www.sciencedirect.com/science/article/pii/S2214860422002378
Disclaimer: The views expressed here are those of the author expressed in their private capacity and do not necessarily represent the views of AZoM.com Limited T/A AZoNetwork the owner and operator of this website. This disclaimer forms part of the Terms and conditions of use of this website.