By Surbhi JainJun 8 2022Reviewed by Susha Cheriyedath, M.Sc.
In an article recently published in the open-access journal Energies, researchers discussed the estimation of energy consumption for electric buses using a physical and data-driven fusion model.

Study: Energy Consumption Estimation for Electric Buses Based on a Physical and Data-Driven Fusion Model. Image Credit: Scharfsinn/Shuterstock.com
Background
The global energy structure is progressively experiencing a shift as energy constraints and pollution problems become more pronounced. Because of their low emissions and excellent energy efficiency, electric vehicles are gaining popularity. Electric buses possess more advantages in the public transportation system than regular diesel buses.
However, several issues remain, such as challenges in evaluating charging needs, planning vehicle routes, and designing battery energy storage systems. As a result, the development of an accurate vehicle energy consumption prediction model can address the aforementioned issues which are critical for the widespread adoption of electric buses.
China's national monitoring and administration platform for new energy vehicles (NEVS) has allowed vast volumes of data about new energy vehicles to be gathered. For the research and modeling of vehicle energy usage, the platform can supply a vast volume of vehicle driving data. Previous studies have used physical modeling approaches or artificial intelligence algorithms to develop vehicle energy consumption models. The results of a single type of model or a single method for estimating energy use are less dependable. A more reliable energy consumption estimating model with few input features is required.
Electric bus operation data transmission and preprocessing results. (a) Driving route, (b) electric vehicle big data cloud platform and (c) preprocessing results of battery SOC. Image Credit: Li, X et al., Energies
About the Study
In this study, the authors discussed the development of a data-driven and physical fusion model for the estimation of electric bus energy consumption. A simplified physical model was used to model the electric bus's basic energy usage. The model considered the effects of brake use, rolling drag, and air conditioning consumption. A CatBoost decision tree model was built to account for the volatility in energy usage caused by many causes. After that, a fusion model was created.
The team tested the performance of the energy consumption model using data from electric buses analyzed on a big data platform. A data-driven and physical fusion model was proposed for vehicle energy usage. Physical formulas were used to express a portion of the energy used by the vehicle when driving, such as the energy used by the rolling resistance and air resistance. The model's complexity was reduced by using formula modeling directly.
The researchers statistically analyzed the data from electric buses. To acquire continuous data in the car charging and driving cycle process, the original data was pre-processed and recreated. The model for estimating energy usage was also created. Based on the electric bus's powertrain dynamic performance, a physical vehicle energy consumption model was constructed.
The least-squares method was used to calibrate the model parameters first. Furthermore, the elements that influence vehicle energy consumption changes, such as driving patterns and environmental factors, were summarized and examined. The impacts were described using the CatBoost decision tree model, and finally, the two models were combined to obtain the final vehicle energy consumption estimation result. The model for estimating energy usage was examined and validated.
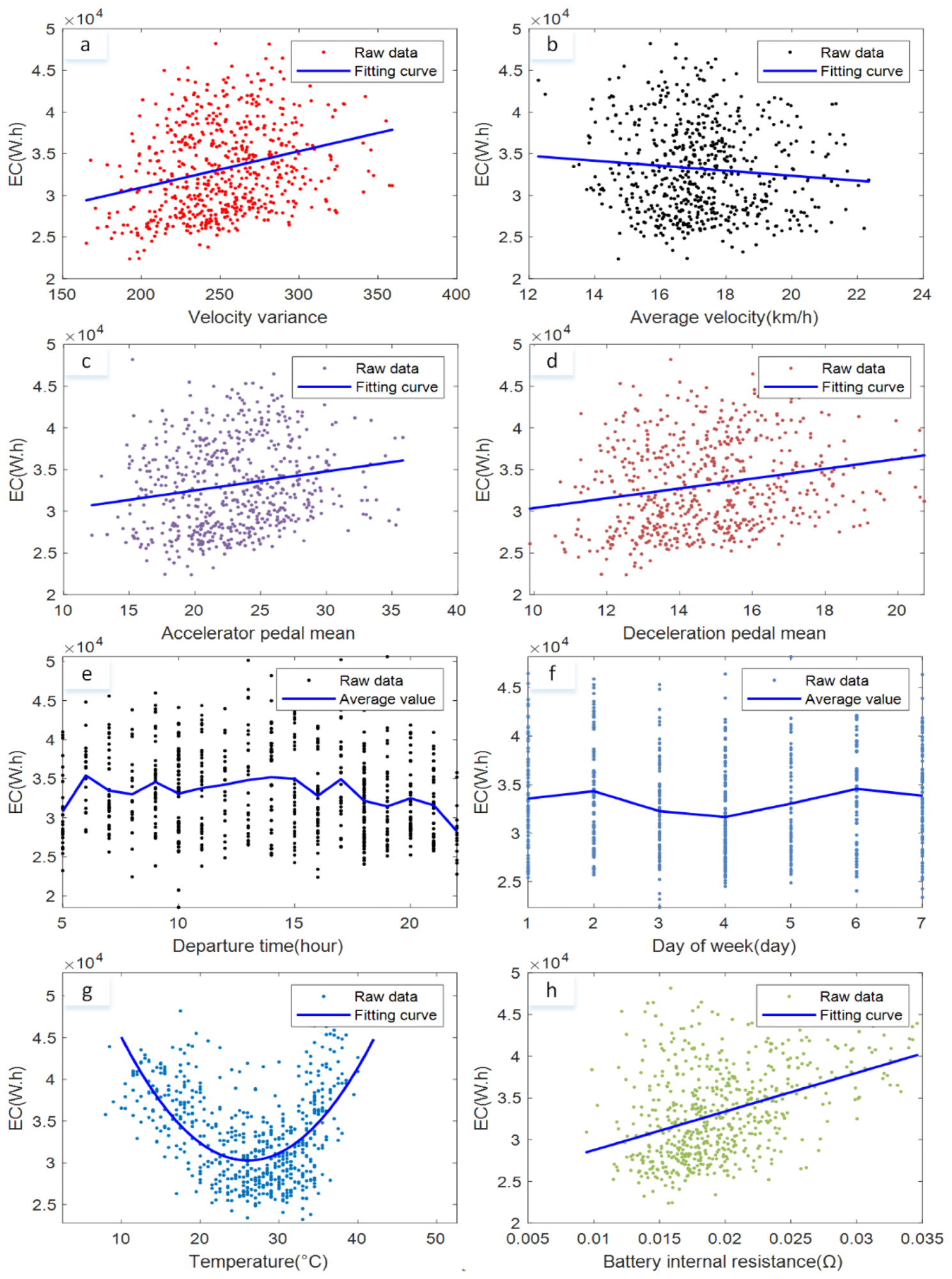
The statistical results of the impact of various energy consumption fluctuation factors on vehicle energy consumption. (a–h) The relationships between the velocity variance, average velocity, number of accelerator pedal presses, number of deceleration pedal presses, departure time, departure date, ambient temperature, internal resistance of the battery pack and energy consumption, respectively. Image Credit: Li, X et al., Energies
Observations
The time taken by data processing was six seconds, while the time consumed for model training was only 0.9 seconds. The results revealed that for many vehicles, the energy consumption estimation errors were within 8.1%. The findings of the vehicle energy consumption estimation had an average inaccuracy of 7.5%. On the Bus 1 dataset, the fusion model's relative error was 4.8%.
The proposed model had a high level of accuracy, with an average relative error of 6.1%. The fusion model was a useful tool for vehicle scheduling, optimizing electric bus energy usage, and the rational organization of charging stations. Other driving factors, such as vehicle departure time, ambient temperature, and so on, had an impact on vehicle driving energy usage. The correlation coefficient was 0.79. With the two indicators, the fusion model outperformed conventional energy consumption modeling methods. The proposed fusion model served as a foundation for vehicle scheduling, optimizing electric bus energy use, and charging station layout. The majority of the points with substantial inaccuracies were concentrated in severe weather.
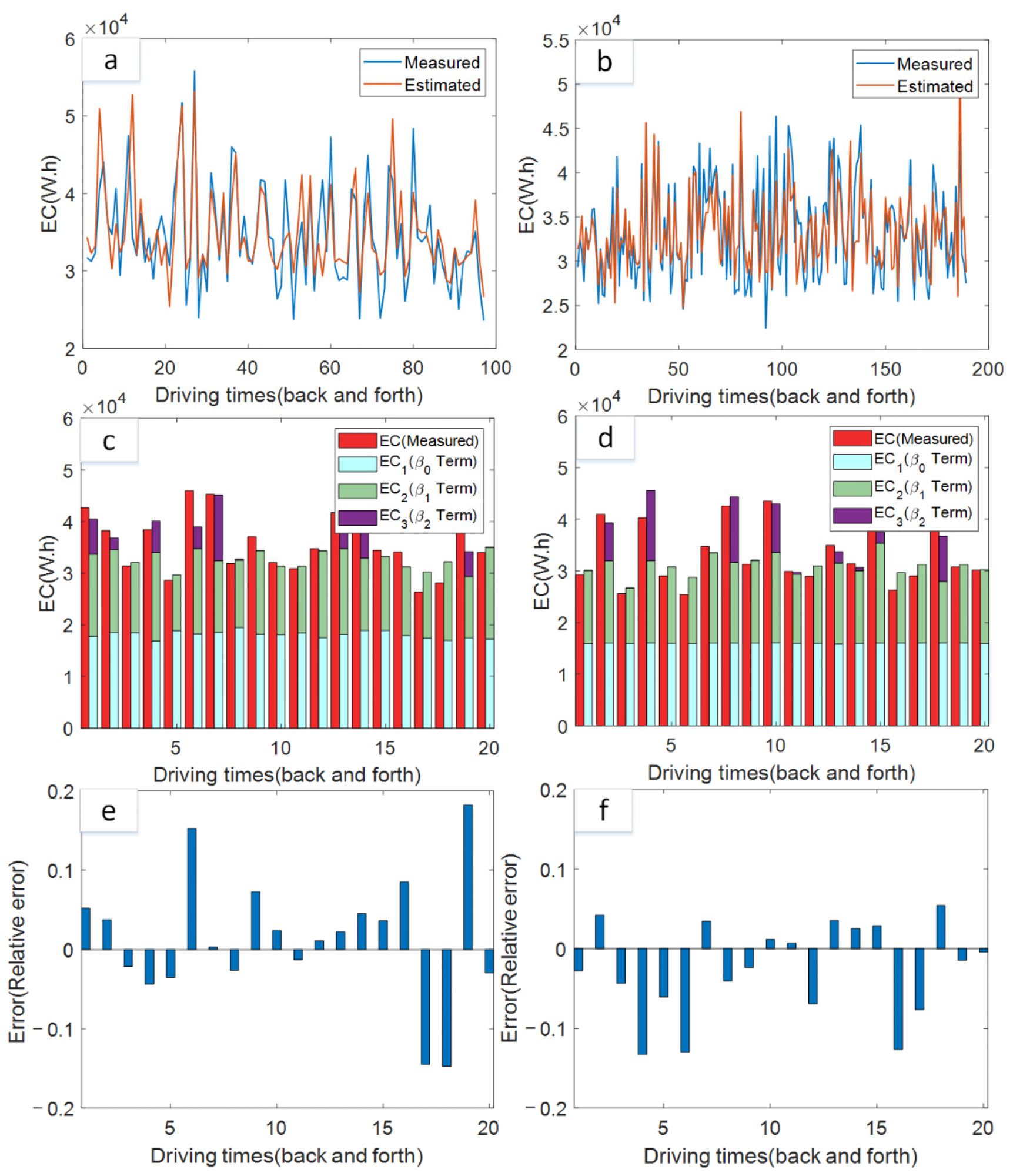
The basic energy consumption estimation results. (a,b) The fitting curves of two electric buses. (c,d) The energy consumption fitting results for each part. (e,f) The fitting errors. Image Credit: Li, X et al., Energies
Conclusions
In conclusion, this study estimated the energy consumption of electric buses using a data-driven and physical fusion model. A rudimentary energy consumption model was built in terms of physical modeling. The effects of kinetic energy consumption, rolling drag, and air conditioning were all taken into account. The primary factors determining the variability of vehicle energy usage were investigated using data-driven modeling. The model's input characteristics were simplified so that the model's input could be built before the car was driven. The model for assessing variable energy use was built using the CatBoost decision tree modeling approach. The concept of integrated learning was used to optimize the model in a hierarchical iteration during the model training process.
The average relative inaccuracy of the vehicle energy consumption estimation result was 6.1%. Furthermore, during the driving process, vehicle mass was treated as a constant value, which contributed to the model error. The authors mentioned that weather parameters could be added to the model in the future to improve its accuracy even further.
Source
Li, X., Wang, T., Li, J., et al. Energy Consumption Estimation for Electric Buses Based on a Physical and Data-Driven Fusion Model. Energies 15(11) 4160 (2022). https://www.mdpi.com/1996-1073/15/11/4160
Disclaimer: The views expressed here are those of the author expressed in their private capacity and do not necessarily represent the views of AZoM.com Limited T/A AZoNetwork the owner and operator of this website. This disclaimer forms part of the Terms and conditions of use of this website.