By Surbhi JainReviewed by Susha Cheriyedath, M.Sc.Jun 23 2022
In an article recently published in the journal Additive Manufacturing, researchers discussed computer vision and deep learning for in-situ optimization of thermoset composite additive manufacturing (AM).
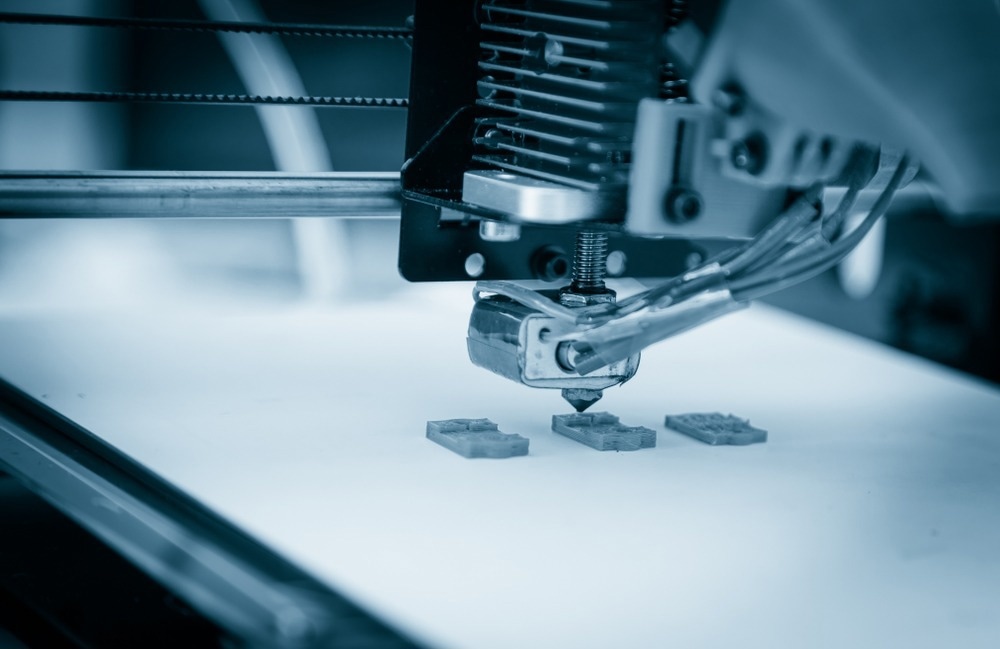
Study: In-Situ Optimization of Thermoset Composite Additive Manufacturing via Deep Learning and Computer Vision. Image Credit: Alex_Traksel/Shutterstock.com
Background
A new extrusion AM process called direct ink writing (DIW) offers unequaled design flexibility with a wide range of feedstock materials. Although composite DIW has made great strides, this technology still has a long way to go before it can be considered a cornerstone of contemporary composite manufacturing. To consistently produce high-quality prints, it is essential to comprehend the complex interactions between the DIW process, print quality, and material performance. Machine learning (ML) methods can be used to simulate the impacts of process parameters and autonomously optimize the AM as a solution to this issue.
Prior to layer-by-layer fabrication, the best parameters can be selected by extracting these correlations using machine learning (ML). Despite the enormous progress made in data-driven AM research, one area that still has to be studied is in-situ process parameter optimization.
Using ML to optimize analytical parameters would require a large data library with time-dependent and environmental features, which would make the task extremely time-consuming. The hierarchical architecture of convolution neural networks (CNNs), which draws inspiration from the brain, enables them to accurately predict future events by extracting highly complex patterns from raw pixel data. These studies concentrate on using CNNs to monitor processes; however, they do not take into account in-situ parameter tuning.
About the Study
In this study, the authors presented a novel optimization framework for thermoset composite AM calibration and printing that made use of computer vision and deep learning (DL). The suggested framework dynamically and autonomously adjusted the printing parameters during extrusion, in contrast to standard DL models where printing parameters were decided upon before printing. To identify the ideal printing parameters, such as road width, print speed, and layer height for a specific composite ink, a revolutionary DL integrated extrusion AM system was created.
The team used a computer that communicated with an extrusion AM system, a camera for in-situ imaging, and many highly accurate convolution neural networks (CNNs) that used the best process parameters for composite AM made up the closed-loop system. In order to autonomously optimize road width, print speed, and layer height in-situ for thermoset carbon fiber composites, this study created a unique DL-DIW framework that combined computer vision and DL models into a DIW system.
The researchers included DL algorithms into a DIW system and created an innovative in-situ parameter optimization framework for DIW printed short carbon fiber composites that could help users save time and effort. This study developed multiple high-accuracy CNNs that would establish the road width, ideal print speed, and layer height in order to accomplish these goals.
Computer vision analysis of single road and layered samples yielded the ideal parameters, and CNN models would afterward update the proper parameters in-situ. The caliber of the composite parts produced using the chosen parameters served as a gauge of the validity of the decision-making models and their precision. Engineers could calibrate their DIW systems without user participation, which effectively demonstrated how DL could have a substantial impact on the composite DIW.
Observations
According to the outcomes, the parameters for a carbon fiber-composite ink could be determined by the suggested process optimization framework on its own. As a result, complex geometries might be produced into specimens with no visible flaws and optimum fiber alignment, which improved the mechanical performance of the specimen's composite material. Additionally, by optimizing the extrusion process without user input, the proposed framework reduced the labor-intensive process necessary to additively construct thermoset composites.
Printing parameters in thermoset composite DIW had a direct impact on print quality and material performance. Therefore, it was crucial to optimize these settings before each printing session. Traditional methods for optimizing printing settings for composite DIW included time- and resource-wasting, laborious trial-and-error trials, which impeded the widespread adoption of this revolutionary technology.
The DL-DIW framework could analyze the defectiveness and quality of the images of both layered and single-road composite ink samples and forecast the ideal printing parameters for the maximum fiber alignment and the least number of visible defects.This 3D printing process was totally autonomous, with its printing parameters modified in situ. Several CNNs were created to detect faults with high accuracy. The CNN created for print speed optimization had an average accuracy of 95.5% in identifying single-road problems.
Both of the binary classification CNNs utilized in the DL-DIW architecture attained at least 85% precision and accuracy across classes of images by enlarging and restructuring the original image collection. The suggested DL-DIW framework determined that the ideal road width, print speed, and layer height for an SFRC ink having 12% carbon fibers by volume were 0.4 mm, 26.67 mm/s, and 0.58 mm, respectively. This confirmed the efficacy of the developed CNNs.
Conclusions
In conclusion, this study elucidated the significance of the proposed DL-DIW system for in-situ printing parameter optimization. This framework could be used with all DIW-based systems to consistently create complicated, high-quality geometries utilizing any composite ink, allowing engineers to calibrate themselves independently.
The authors stated that the proposed DL-DIW framework should be further optimized in the future. They suggested that even though the suggested framework was successful in identifying the ideal printing parameters, more work might be done to enhance the CNNs' performance and switch the tandem binary classification CNN system for a single DL model.
They also believe that these new developments would strengthen the foundational role of the DL integrated DIW system in contemporary composite manufacturing.
More from AZoM: Building Durable and Sustainable Futures with Graphene@Manchester
Disclaimer: The views expressed here are those of the author expressed in their private capacity and do not necessarily represent the views of AZoM.com Limited T/A AZoNetwork the owner and operator of this website. This disclaimer forms part of the Terms and conditions of use of this website.
Source:
Wright, W. J., Darville, J., Celik, N., et al. In-Situ Optimization of Thermoset Composite Additive Manufacturing via Deep Learning and Computer Vision. Additive Manufacturing 102985 (2022). https://www.sciencedirect.com/science/article/abs/pii/S2214860422003785