A paper recently published in the journal Sustainability demonstrated the feasibility of using a novel interval-energy forecasting method based on deep learning for sustainable building management.
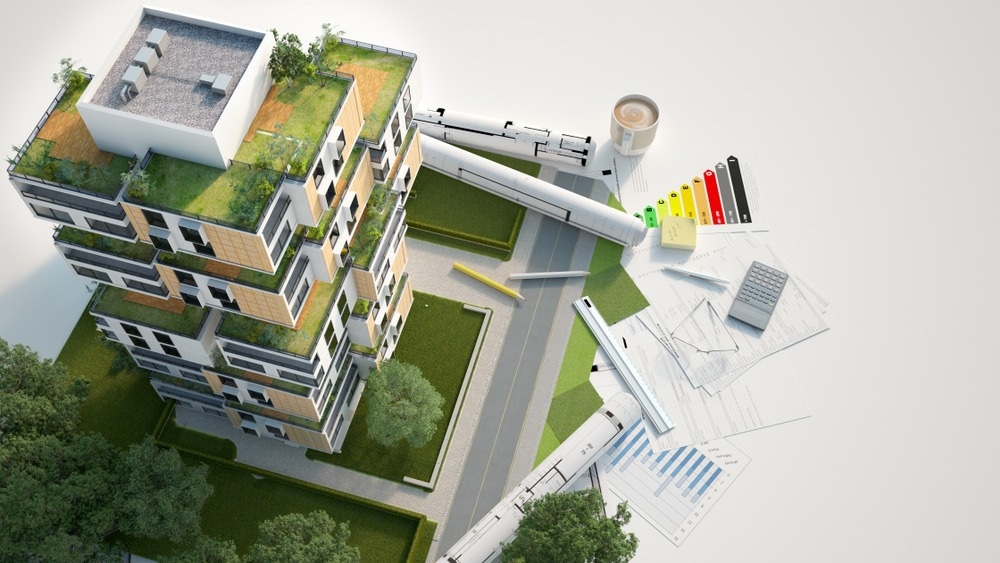
Study: A Novel Interval Energy-Forecasting Method for Sustainable Building Management Based on Deep Learning. Image Credit: Franck Boston/Shutterstock.com
Background
Energy consumption in the office building sector accounted for almost one-fifth of China’s overall energy consumption in 2015, which necessitated the study of energy use by office buildings to implement energy conservation policies in the country.
Data-driven methods can be used extensively to enhance building energy efficiency as advancements in information technology (IT) allow the recording and collection of data related to building operations on a wide scale.
Typically, the office building energy prediction time horizons can be categorized as long-term for one year, medium-term for one week to one month, and short-term for one day or less. Among these time horizons, short-term forecasting has attracted greater attention due to its applications in anomaly detection and real-time control.
Since long- and medium-term forecasting studies primarily involve the evaluation of building energy consumption and formulation of energy plans, ensuring the forecasting result accuracy has remained a priority for researchers. Probabilistic load forecasting is more suitable compared to deterministic load prediction for long-term planning and management of building energy consumption.
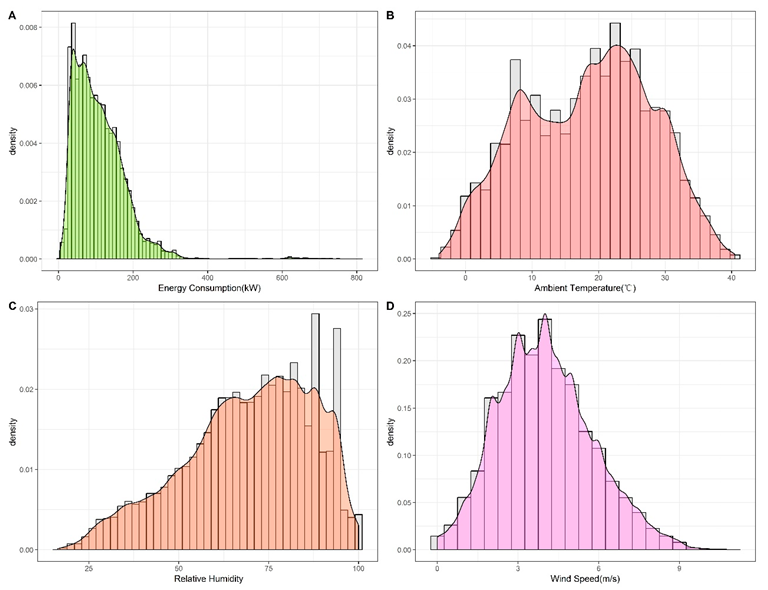
Probability distribution of the indoor load and weather data. Image Credit: Duan, Y, Sustainability
The Study
In this study, researchers analyzed the prediction performance of a novel probabilistic load-forecasting method based on the penalized quantile regression (PQR) and long short-term memory (LSTM) model (LSTM-PQR) for weekly and daily indoor load in an office building. LSTM, a deep learning method derived from recurrent neural networks (RNN), can manage the long-term dependency problems of RNN.
Researchers also performed a comprehensive analysis for one year using the LSTM model and verified the advantages of deep learning for long- and medium-term forecasting by comparing the model with other commonly used machine-learning (ML) models, including random forest (RF), support vector machine (SVM), and back propagation neural networks (BPNN).
The data utilized in this study to calculate the indoor load was composed of humidity, temperature, and the flow rate of the air outlet and inlet of all air-conditioning (AC) units in an office building located in Shanghai, China. Additionally, the meteorological data of the nearby airport located within seven km from the target building was obtained using the worldmet package using the open source R software and utilized as the actual meteorological data of the building.
All datasets were recorded from 17 April 2017 to 3 May 2018 with 15 min resolution. Researchers ignored the date information of input variables considering that LSTM can learn the context needed to make predictions in time-series forecasting problems and the order dependence between items present in a sequence.
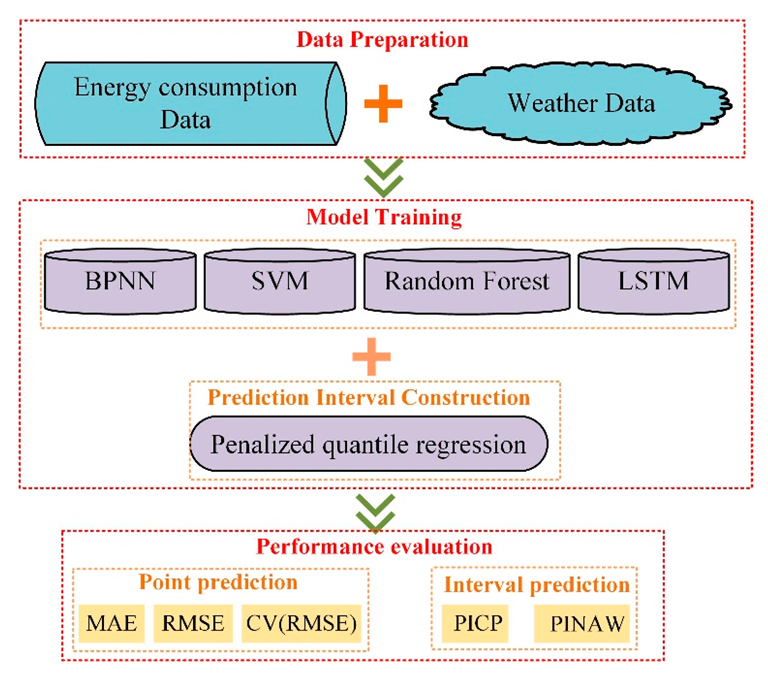
Research outline. Image Credit: Duan, Y, Sustainability
RF, SVM, BPNN, and the proposed LSTM were used for model training. LSTM was selected as the basic point estimation model, while RF, SVM, and BPNN were used as reference models. PQR was selected to construct the prediction intervals depending on the prediction results of all point estimation algorithms.
The weekly and daily indoor-load-forecasting performance of each point estimation model was assessed using the traditional point forecast evaluation criteria, including coefficient of variation of root mean square error CV(RMSE), root mean square error (RMSE), and mean absolute error (MAE).
The prediction interval normalized average width (PINAW) and prediction interval coverage probability (PICP) were used for quantitative comparison to evaluate the interval forecasting results of the models. All interval forecasts were within the 95% prediction interval.
The PICP investigated whether the actual energy consumption value is within the predicted interval, while PINAW was utilized to measure the predicted interval width. Different evaluation metrics were compared using the entropy weights method (EWM), an information-weighting method used extensively in decision making.
Grid search, a commonly used optimization algorithm, was used to identify the optimal hyperparameters for the ML methods. In the hyperparametric optimization process, the model performance was tested by comparing the actual energy data with the predicted energy consumption. The parameters in the LSTM, RF, SVM, and BPNN were optimized to increase the accuracy of the energy prediction models.
Observations
The point prediction performance results of the models were evaluated on the test data containing complete and continuous data for 112 days. The results of 112 days were obtained for one day ahead forecasting, with each day containing 96 predicted values. Similarly, results of 16 weeks were obtained for one week ahead forecasting, with each week containing 672 predicted values.
Notable differences were observed between the MAE results of the test models for weekly and daily load forecasting. Specifically, RF and SVM displayed the worst performance for one day ahead forecasting and one week ahead forecasting, respectively, in terms of MAE.
No considerable performance difference in the MAE results was observed between BPNN and LSTM. However, the median MAE of LSTM was lower than BPNN. These observations indicated that the LSTM model was more reliable and advantageous compared to other models.
The RMSE results of the models for weekly and daily load forecasting demonstrated that the performance of the LSTM was better than the three reference models. However, several extremely high values were observed in the LSTM and RF models owing to the failure of these in certain situations due to an increase in the predicted error with the number of iterations.
A significantly higher difference was observed between the RSME of the reference models and LSTM, indicating that the LSTM model outperformed other models owing to the smaller dispersion degree of RMSE distribution.
In the CV(RMSE) results, considerable differences were observed between the models. SVM demonstrated the worst performance in one day ahead load forecasting as the CV(RMSE) of SVM was higher than other models.
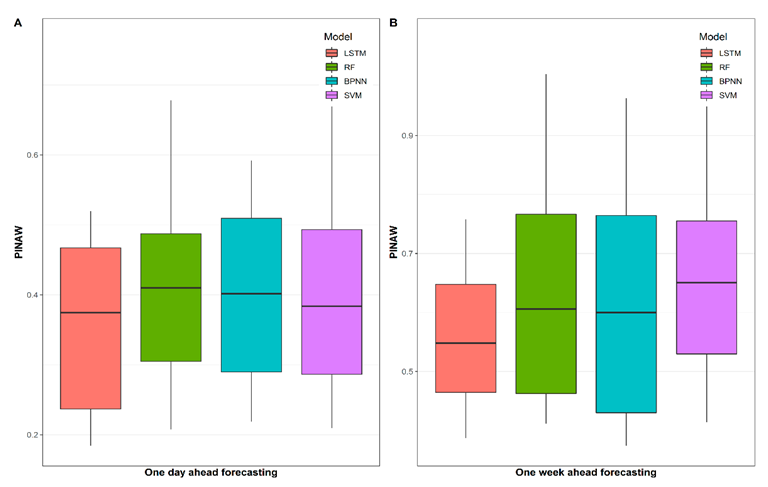
PINAW results of proposed models for daily and weekly interval load forecasting with 95% prediction interval. Image Credit: Duan, Y, Sustainability
The overall distribution of CV(RMSE) in one week ahead load forecasting was lower than that of one day ahead load forecasting, indicating that the energy consumption trend prediction was relatively accurate in the short term.
Thus, the LSTM model performed the best among the commonly used prediction models based on the point prediction results as the model learned the energy consumption data periodic characteristics in the time domain. However, the LSTM model displayed a large performance gap during short-time load forecasting due to the accumulation of prediction errors with the increasing number of prediction steps.
The interval prediction results of 16 weeks were obtained by the point estimation models integrated with PQR. The largest and smallest prediction interval widths were observed in RF and SVM, respectively. The LSTM obtained the lowest PINAW and the highest PICP among all models, which indicated the suitability of the model for weekly interval load forecasting. The prediction interval of RF and LSTM covered almost all the actual load in several weeks' predictions as the highest PICP of these models was close to 1.
The prediction performance of all models improved for daily load in terms of PICP due to the relative simplicity of the short-term time horizon. LSTM-PQR maintained the best predictive performance for daily load, while RF and SVM demonstrated the highest PINAW value and lowest PICP value, respectively. The mean PICP of LSTM was 20.9% and 6.4% higher than SVM and RF, respectively.
Conclusion
To summarize, the findings of this study demonstrated that the novel LSTM-PQR model could accurately predict the indoor load of a building within a specific time horizon. The LSTM-PQR model led to reliable and accurate results in daily and weekly interval load forecasting, with the highest composite score of 99.60 obtained for both daily and weekly predictions.
Thus, the method is compatible with probabilistic load forecasting, which could guide the management of building sustainability in a carbon-neutral scenario in the future.
Disclaimer: The views expressed here are those of the author expressed in their private capacity and do not necessarily represent the views of AZoM.com Limited T/A AZoNetwork the owner and operator of this website. This disclaimer forms part of the Terms and conditions of use of this website.
Source:
Duan, Y. A Novel Interval Energy-Forecasting Method for Sustainable Building Management Based on Deep Learning. Sustainability 2022. https://www.mdpi.com/2071-1050/14/14/8584