A new paper in the journal Energies has investigated different methods for estimating state of charge in lithium-ion batteries, a key technology in applications such as electric vehicles. The research has been conducted by scientists from Brazil.

Study: Method for SoC Estimation in Lithium-Ion Batteries Based on Multiple Linear Regression and Particle Swarm Optimization. Image Credit: cigdem/Shutterstock.com
The Rise of Electric and Hybrid Vehicles
The transportation sector is a well-recognized contributor to anthropogenic climate change and air pollution. Internal combustion engines utilize non-renewable petrochemical-based fuels, which are drivers of carbon emissions. To overcome the environmental and health challenges associated with ICE vehicles, companies have developed electric and hybrid vehicles in recent decades.
The market share of fully electric and hybrid vehicles has increased year on year, with multiple automobile manufacturers now offering options for customers. A report by Hazra and Reddy has estimated that by 2030, electric vehicles will have a 7% market share. Governments have introduced policies to significantly reduce and eventually completely phase out new ICE vehicles over the coming decades.
Batteries are a central element of electric and hybrid vehicles, with lithium-ion batteries the main types used by manufacturers. These battery variants possess long service lives, with 80% discharge over three thousand cycles, fast charge, high energy density, and high voltage compared to other commercially available battery types used in the automotive industry.
There are, however, some critical disadvantages to electric vehicles compared to conventional ICE technologies. Challenges with charging time, autonomy, charging infrastructure, and low improvement rates of battery technologies hinder the full commercialization of these alternative transportation options. The urgent need to commit the world to zero carbon emissions requires these challenges in electric transportation to be overcome post haste.
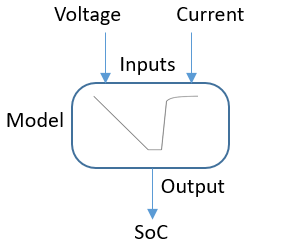
Battery SoC Model, based on the voltage and current information. Image Credit: Castanho, D et al., Energies
State of Charge Estimation
Improving the performance of lithium-ion batteries for EV applications is central to the commercialization of these alternative transportation options. Power management control strategies aim to enhance power distribution in EV systems amongst energy storage components and traction motors. Studies have indicated that power source degradation influences these strategies.
Efficient power management strategies help to mitigate critical performance issues and enhance battery life cycles. Amongst the various strategies developed in recent years, state of charge estimations are fundamental to power management control performance. Real-time monitoring of battery state of charge is essential. Establishing an accurate state of charge estimations is vital for the efficiency of control systems.
In recent years, several methods have been developed for battery state of charge estimation, with multiple computational models and algorithms explored in research. Data-driven deep learning estimation methods, adaptive filter algorithms, analytical battery models including the Shepherd model, particle swarm algorithms, and backpropagation neural networks have all shown promise as state of charge estimation methods.
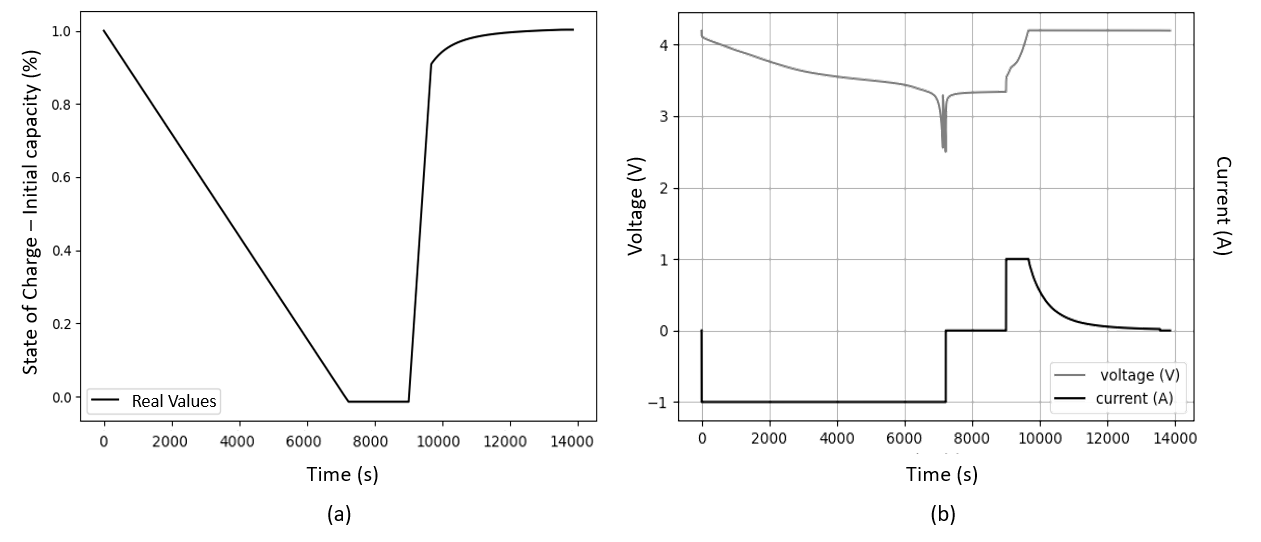
Initial capacity of the battery. (a) Battery SoC model. (b) Current and voltage profiles for the initial capacity. Image Credit: Castanho, D et al., Energies
The Study
The new study in Energies has investigated and compared different state of charge estimation methods for lithium-ion batteries which can be used in the electric vehicle sector. Four different conduction profiles were tested under different temperature conditions.
The Multiple Linear Regression model both with and without spline interpolation was employed in the research, along with the Generalized Linear Model. Three different optimization techniques (Differential Evolution, Particle Swarm Optimization, and Genetic Algorithm) were used to calibrate the models. The free coefficients of the models were calculated using these bio-inspired metaheuristics.
Study Conclusions
The aim of the study was to evaluate the performance of each model for state of charge estimations in order to find a low-computational cost and simple computational strategy with high assertiveness and accuracy.
Training and test set computational results indicated that a Multiple Linear Regression with Particle Swarm Optimization provides the best performance for state of charge estimation. This approach provides the best estimator in all case studies performed in the research.
Notably, this optimized model outperforms current methods reported in the literature. The model requires a relatively small computational cost, making it a competitive candidate for commercial applications such as electric vehicles.
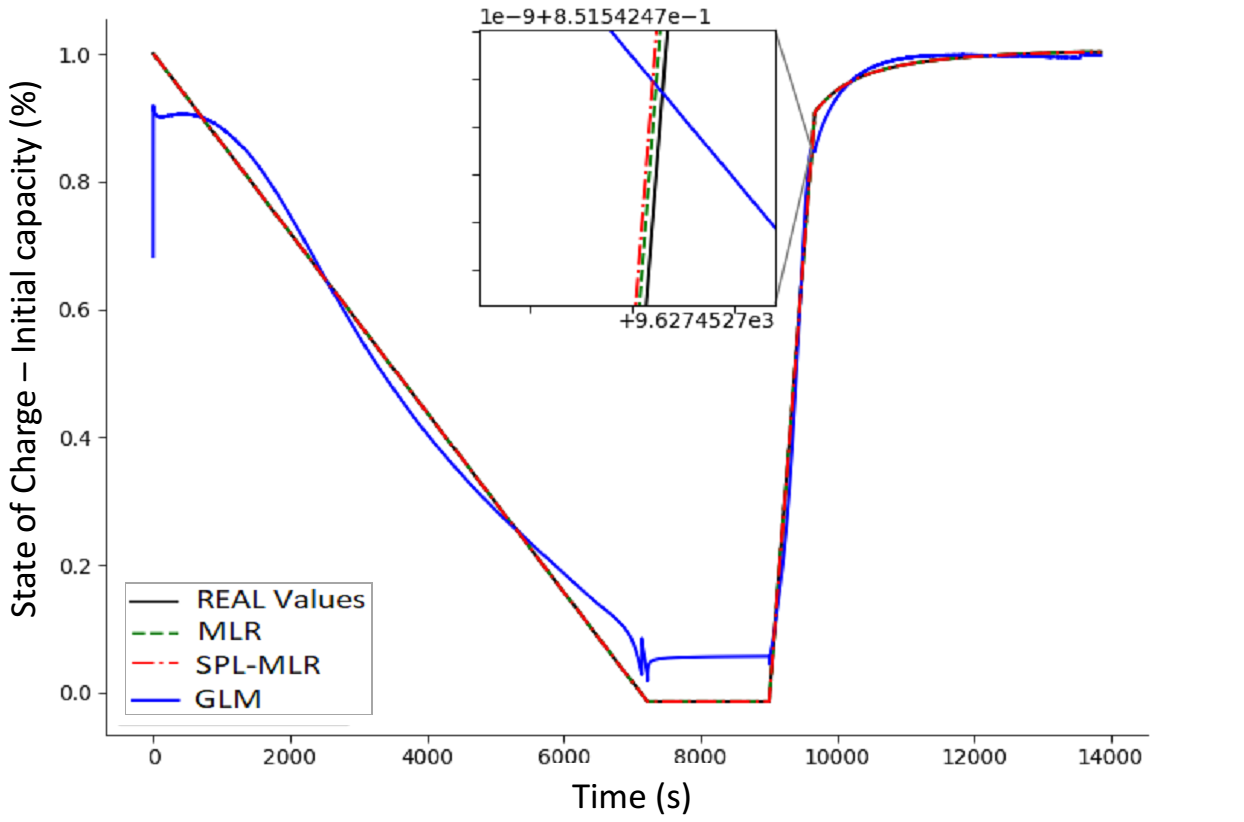
Initial Capacity Curve for PSO Training. Image Credit: Castanho, D et al., Energies
The paper has made a few recommendations for future research in this area. The authors have highlighted the need to conduct tests on different battery models and under different conditions. Additionally, different C rates should be tested. One approach which will aid these efforts is the development of new databases.
It has also been noted in the research that the current literature contains over a hundred different algorithms than the ones evaluated in the research, which provides significant opportunities for future researchers to evaluate their suitability for state of charge estimations. Further investigations will widen the knowledge base on this key research question.
Further Reading
Castanho, D et al. (2022) Method for SoC Estimation in Lithium-Ion Batteries Based on Multiple Linear Regression and Particle Swarm Optimization Energies 15(19) 6881 [online] mdpi.com. Available at: https://www.mdpi.com/1996-1073/15/19/6881
Disclaimer: The views expressed here are those of the author expressed in their private capacity and do not necessarily represent the views of AZoM.com Limited T/A AZoNetwork the owner and operator of this website. This disclaimer forms part of the Terms and conditions of use of this website.