By Surbhi JainReviewed by Susha Cheriyedath, M.Sc.Oct 3 2022
In an article recently published in the journal Additive Manufacturing, researchers discussed the utility of computer vision to monitor 3D concrete printing continuously.
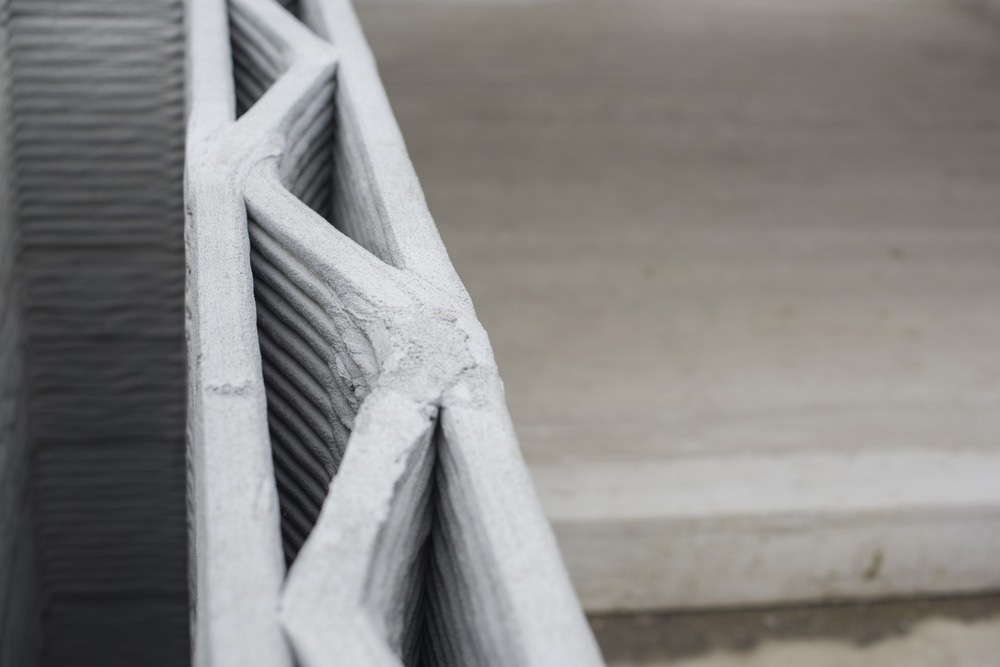
Study: Inline monitoring of 3D concrete printing using computer vision. Image Credit: Matjazz/Shutterstock.com
Background
With real-world instances of 3D concrete printing (3DCP) at the building scale, construction based on additive manufacturing is now approaching its maturity. To make this technology repeatable and certifiable, there is still work to be done on material development, construction methods, and process control despite these high-profile applications. The 3DCP extrusion is influenced by a wide range of process variables. As a result, numerous monitoring techniques have been put forth at various points.
Inline monitoring's goal is to give operators the ability to take corrective action in the event of unusual behavior. As demonstrated in the literature for manufacturing processes at various size scales, this idea can be applied to automatic control systems. Vision systems can also calculate the thickness of the layers. Vision systems can also analyze the viewed surfaces in terms of attributes like texture, allowing them to go beyond simple geometrical property monitoring.
About the Study
In this study, the authors discussed a computer vision-based methodology for anomaly identification. Three different modules, namely, picture acquisition, interlayer layer and line segmentation, characterization of the local texture and geometry of the layers, and anomaly detection, made up this methodology. A camera that was mounted to the printing nozzle was used for the picture acquisition process. The lines dividing the printed layers were recognized and located by the suggested layer segmentation approach with F-score = 91%. The third module analyzed the geometry of the layers and the material's texture using the segmentation and the original image as input.
The team used the results to identify the geometric abnormalities when the values were outside the predicted range. Four different quality levels were assigned to the material texture. The study discussed the findings to demonstrate how well the proposed system worked for the automatic location of anomalies on photos captured during a printing session. The interlayer lines in an image were determined using a model based on deep Learning (DL), and then the independent printed layers were segmented using these lines as a reference.
The researchers applied image processing to establish the local geometry in terms of orientation, layer thickness, curvature, and proximity to the nozzle based on the interlayer lines. These metrics were employed to identify and pinpoint anomalies in the measured layers relative to the expected values. A monitoring technique based on layer textural characterization was discussed.
Observations
Fluid scored 100% across all folds, which was the best result of the four classes. On the other hand, dry had the highest average difficulty of 90.2% across the five folds. The averages for the good and tearing classes were a little higher, at 93.7 and 92.2%, respectively. On the testing loss plateau with a tolerance of 5 epochs, the learning rate was reduced by a factor of 2.
The interlayer lines that separated neighboring extruded layers could be segmented in line with the assistance of deep learning. An F-score of 91% was obtained for the suggested model. The detection of interlayer lines in the images under analysis enabled additional segmentation of the independent layers. Multiple geometrical parameters of the printed object could be measured at once by a study of the segmented interlayer lines. Measurement of these characteristics enabled the detection of anomalies in the printed item. When the water content of the extruded material exhibited anomalies, it could be determined by analyzing the texture of the independent layers. The tests demonstrated that a single red-green-blue (RGB) camera's input could be used to perform both geometrical and textural analysis simultaneously. This could increase the number of geometrical measurements that could be made by computer vision.
Conclusions
In conclusion, this study discussed a methodology for inline computer vision-based 3D concrete printing monitoring. A study of texture for 3D printing concrete, influenced by techniques currently employed in small-scale and extruded-based additive manufacturing, was presented. The authors discussed how the proposed methodology provided the operator with the precise location and type of abnormalities that were found. They mentioned that if the outcomes are documented, they could be used as a quality report once printing is complete. It could also be used to reject the item when serious irregularities are found. The operator could be informed of the type and severity of any anomalies found to make necessary corrections. This monitoring could be expanded in a subsequent study to include closed-loop control.
The team stated that an automated decision system could use the kind and severity of anomalies as input before issuing a remedial action for the printing machine. It could come from supervised machine learning models, vector-to-action dictionaries, rule-based expert systems, etc.
More from AZoM: How Far Can Semiconductor Manufacturing Be Automated?
References
Rill-Garc´ıa, R., Dokladalova, E., Dokl´adal, P., et al. (2022) Inline monitoring of 3D concrete printing using computer vision. Additive Manufacturing, 103175. https://www.sciencedirect.com/science/article/abs/pii/S2214860422005644
Disclaimer: The views expressed here are those of the author expressed in their private capacity and do not necessarily represent the views of AZoM.com Limited T/A AZoNetwork the owner and operator of this website. This disclaimer forms part of the Terms and conditions of use of this website.