By Surbhi JainNov 18 2022Reviewed by Susha Cheriyedath, M.Sc.
In an article recently published in the journal ACS Energy Letters, researchers discussed the advanced characterization of battery electrodes driven by machine learning.
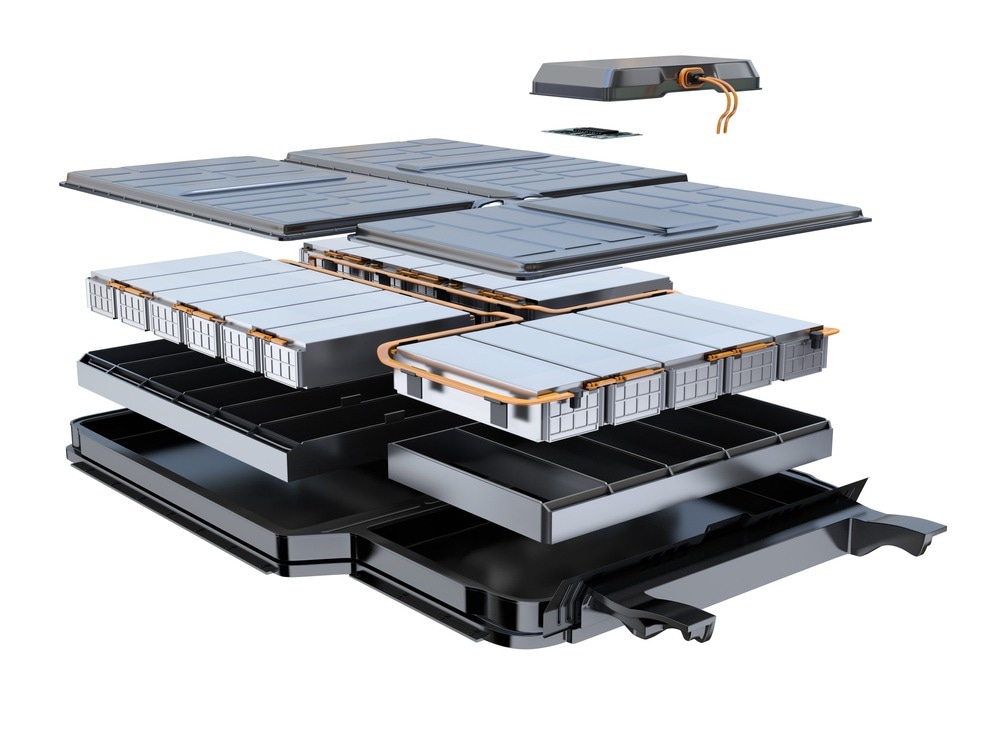
Study: Machine-Learning-Driven Advanced Characterization of Battery Electrodes. Image Credit: Chesky/Shutterstock.com
Background
Numerous material characteristics with geographically and temporally variable chemistry, morphology, and crystallography are related to the performance of lithium (Li)-ion batteries. Since Li-ion batteries were initially made available commercially, they have seen significant advances as a result of controlling these attributes to obtain favorable cell performance.
Advanced characterization techniques are frequently required to confirm the management of material properties, yet there are still many things that analytical equipment cannot practically or physically measure. Artificial intelligence (AI) techniques to improve, classify, integrate, forecast, or create data artificially have advanced significantly during the past ten years.
Many commercial data-analysis software programs have begun to include AI approaches, for instance, to improve correlation data across multi-modal techniques and spatial resolution or recognize and quantify patterns in the data. Each year, new methods for using AI to improve data are demonstrated, and many of these methods are soon used in commercial applications. An especially pertinent illustration is the fact that datasets are being generated using AI approaches with more information than any one characterization technique is capable of, thereby going beyond the capabilities of the hardware. Despite the progress made so far, there is still plenty of room to use AI techniques to improve our characterization abilities, giving researchers the ability to measure and consequently manage battery material qualities that are otherwise impossible to get.
About the Study
In this study, the authors discussed the performance constraints of lithium-ion battery electrodes and how they require a solid understanding of materials characterization. They also elucidated the significant progress in laboratory-based characterization techniques and the structure-function relationship of electrodes.
The team observed that by utilizing data gathered from a variety of methodologies, AI demonstrated considerable potential for achieving representative, 3D, multi-modal datasets. A summary of current developments in lab-based characterization methods was provided for Li-ion electrodes. They discussed how AI methodologies might integrate and improve these methods, significantly advancing the understanding of electrodes.
The researchers provided an overview of the relevant multi-length-scale battery material properties, discussed the drawbacks of lab-based materials characterization methods, and also discussed how recent advances in AI could be applied to get over these drawbacks. From this perspective, the systemic constraints of microscopy techniques could be overcome by combining multi-modal data with generative adversarial networks (GANs). Further, a scenario was sketched out in which AI techniques allow data to be combined into a single, multi-modal dataset that covered a variety of length scales.
Observations
To use transmission electron microscopy (TEM) methods, samples must typically be thinner than 100 nm or less than 10 nm for atomic resolution. The sample slice must then be micro-manipulated for mounting and imaging. Another example of a sample preparation limitation was the requirement for nano-X-ray computed tomography (XCT) samples to be only slightly broader than the field of view, or about 0.1 mm, to achieve the best attenuation. It was impossible to perform correlative metrology utilizing several procedures and length scales or to establish statistical confidence in the methodology due to the difficulties with sample preparation.
The time required to cut small samples significantly decreased after the recent introduction of laser milling in electron microscopes. Systematic barriers that prohibited the simultaneous gathering of the many performance-influencing material attributes could be overcome by AI-enhanced characterization. The creation of greater resolution and larger dimensionality datasets was made possible by CNN-based approaches.
Additionally, information gathered from all methods of characterization could be combined to create unified representative volumes of electrode microstructure. These unified volumes could support thorough multi-physics simulations and high-throughput optimization when combined with electrochemical data.
Although Li-ion electrodes were employed as a specific case example, the general methodology was broadly relevant to different fields of energy materials research. High-quality data would be essential to the success of the new paradigm for characterization that these capabilities presented. Datasets that could be read or understood by humans were not necessary for neural networks. The full potential of data-driven ML was not realized when the networks were constrained to output data in formats like 3D volumes representing various material qualities so that physical processes could be stimulated on them.
Conclusions
In conclusion, this study elucidated that more abstract models could be created using data from the entire process, from creation to demise, if the imaging data was integrated with other data streams. These models would omit the limitations necessary to produce findings that could be interpreted by humans as well as the assumptions and simplifications included in the models.
The authors mentioned that to achieve this goal, careful consideration and planning must be given to pertinent variables as well as the gathering, organizing, and processing of data. They believe that a deeper knowledge of the numerous physical heterogeneities in the materials is necessary for future advancements.
More from AZoM: How are Bioplastics Made?
References
Finegan, D. P., Squires, I., Dahari, A., et al. Machine-Learning-Driven Advanced Characterization of Battery Electrodes. ACS Energy Letters 7, 4368-4378 (2022).
https://pubs.acs.org/doi/10.1021/acsenergylett.2c01996
Disclaimer: The views expressed here are those of the author expressed in their private capacity and do not necessarily represent the views of AZoM.com Limited T/A AZoNetwork the owner and operator of this website. This disclaimer forms part of the Terms and conditions of use of this website.