.jpg)
In this interview Professor Harald Pasch talks about The Importance of a Field-Flow Fractionation System When Characterising Polymer Self-Assemblies.
For the benefit of our readers could you explain what polymer self-assemblies are?
The ability of block copolymers to self-assemble into various nanostructures, such as micelles or vesicles, when dissolved in a selective solvent is based on the specific amphiphilic character of the block copolymer. Amphiphilic block copolymers combine a polar, hydrophilic polymer sequence (block) with a non-polar, lipophilic polymer sequence. Such block copolymers can be synthesized via different controlled polymerization processes as shown in Fig. 1a. In a selective solvent i.e. a solvent that is a thermodynamically good solvent for one block but a poor solvent/precipitant for the other, the ‘insoluble’ block aggregates and forms a core while the ‘soluble’ block forms a corona that interacts with the solvent and stabilizes the polymer self-assembly. To stabilize the core, in some cases cross-linking reactions are used. Depending on the arrangement of the block copolymer molecules in the self-assembly, different architectures can be formed as shown in Fig. 1b.
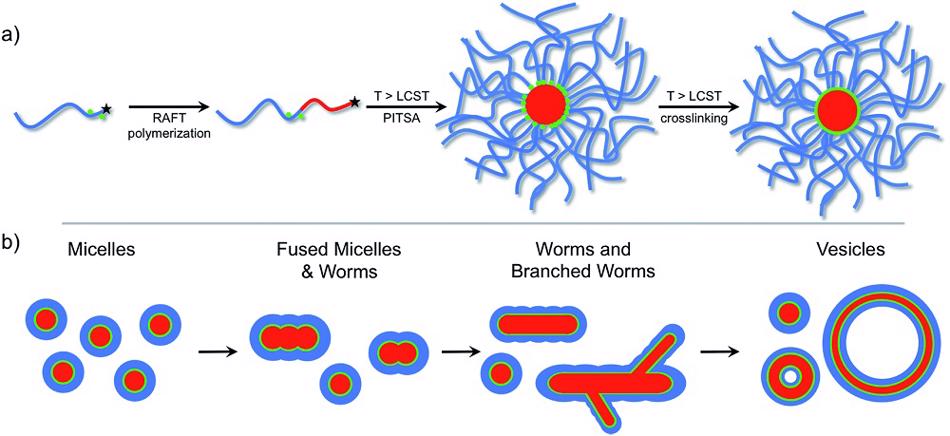
Figure 1. (a) Synthesis and self-assembly of an amphiphilic block copolymer. (b) Progression of self-assembly morphology as a function of block copolymer composition (Image adapted from C.A. Figg et al., Chemical Science 2015, 6, 1230-1236).
Why are polymer self-assemblies important?
Self-assembly of nanoscale structures has provided a powerful path to self-assemblies with specific functions. As explained before, block copolymers are a versatile class of self-assembling materials characterized by chemically distinct polymer blocks that are covalently bonded. Polymer compositions can be chosen to control the micelle size and compatibility with specific substances including drugs. Therefore, polymer self-assemblies are attractive drug carriers that, depending on their size, shape and composition can be subjected to the human body to transport drugs to specific locations (e.g. tumor cells) where they release the drug. The challenges here are the difficulty of reproducing or controlling the size of the self-assemblies, preparing predictable size distributions, and ensure the stability of self-assemblies with high drug loads. Other applications include magnetic drug delivery where magnetoresponsive and superparamagnetic nanostructures with highly anisotropic shapes (chain-like) can be manipulated using magnetic fields. The encapsulation capabilities and the biocompatibility of micelles and vesicles together with the large diversity of other morphologies (cylindrical micelles, worms, nanorods) makes them interesting vehicles for in vivo cell absorption and the ability to encapsulate molecules in both their hydrophobic vesicle membrane and their hydrophilic interiors, providing the possibility of dual component delivery. Other applications include colloid stabilization and micro- and nanoreactor processes.
What methods are available to characterise polymer self-assemblies?
In order to make full use of their potential, polymer self-assemblies must be characterised extensively in terms of size, aggregation number, morphology, corona composition and molar mass as these parameters can significantly influence the function and behaviour in different applications. Current bulk characterization techniques such as electron microscopy (SEM and TEM), atomic force microscopy (AFM) as well as dynamic (DLS) and static light scattering (SLS) are frequently used to determine particle sizes and particle size distributions. However, these techniques cannot be used to determine molar masses, chemical compositions or aggregation numbers. Furthermore, although average corona compositions and number average molar masses can be probed by NMR and fluorescence spectroscopy, these techniques cannot determine the dispersity of these parameters. More importantly, the suitability of current bulk characterization techniques is limited for samples exhibiting complex or multiple size, molar mass or composition distributions.
Why did you choose field-flow fractionation for characterising polymer self-assemblies?
To obtain polymer property distributions (most importantly molar mass distributions), selective fractionation methods must be used. The gold standard for molar mass determination is size exclusion chromatography (SEC) as it not only provides reliable and accurate information but it also has a well-developed theoretical foundation from which to predict and explain the retention behaviour of polymers. However, SEC separates polymers according to their size in solution and not according to their molar mass. The correlation between hydrodynamic size and molar mass is obtained through SEC calibration or the use of molar mass sensitive detectors. SEC also has limitations when it comes to complex or high molar mass samples (such as block copolymers, polyelectrolytes and polymer self-assemblies) as these can undergo shear degradation or even adsorption onto the stationary phase.
To address the limitations inherent to column-based fractionation techniques, channel-based fractionation techniques, such as Field-Flow Fractionation (FFF), have become important alternative fractionation platforms to separate and characterise not only polymers but also other complex analytes such as polymer self-assemblies. One of the most prominent sub-techniques of FFF for polymer characterization is Thermal Field-Flow Fractionation (ThFFF) as it can separate polymers and polymer self-assemblies based on not only their size in solution (or molar mass) but also according to their chemical composition, in a single analysis. Over the last few years, both the development of ThFFF theory and the application of the technique to characterise complex analytes has shown tremendous growth and as a result has started to gain increased attention from the polymer community.
What does the field-flow fractionation system that you use comprise of?
We are working with two systems, an asymmetric flow FFF (AF4) and a ThFFF system. As these systems in many respects are similar to column-based fractionation systems, a range of detectors that are also used for column chromatography, can be attached to AF4 and ThFFF. The FFF platform has a modular architecture and, therefore, depending on the analytical problem different FFF devices can be combined with different detectors. The setup of our current ThFFF system is presented in Fig. 2. It comprises the ThFFF fractionation device and five information-rich detectors that are online coupled to the device. The detectors can be used in different combinations to provide selective molecular structure information. Chemical compositions can be determined using dual concentration detection (dRI and UV) while hydrodynamic radii and radii of gyration can be analysed by dRI-MALS-DLS. Information on molecular conformation are accessible via Mark-Houwink and conformation plots that require concentration, MALS and dVis detection.
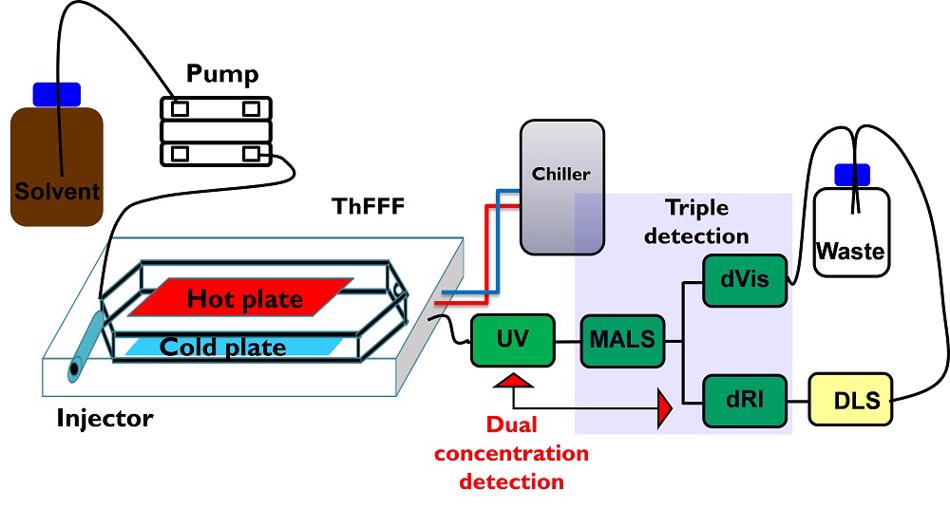
Figure 2. Schematic presentation of the current setup for ThFFF using five different information-rich detectors: ultraviolet (UV), differential refractometer (dRI), multiangle light scattering (MALS), dynamic light scattering (DLS), differential viscosimeter (dVis).
Why did you choose Postnova for this field-flow fractionation equipment?
In the analytical instrumentation market, there are only very few companies that have FFF instrumentation in their product portfolio. One of them is Postnova that specialized on FFF in general and different FFF sub-techniques in particular. On top, Postnova offers a large range of detectors that can be used for FFF and column-based fractionations alike. Since, in our group, we focus on advanced channel- and column-based fractionations it is very useful to use the same components for different types of fractionation. An additional advantage is that software communication between the different components is very easy because the programs are based on the same platform.
How do you see your field of research developing in the near future?
Compared to column chromatography, FFF will always be a niche technology. However, as FFF is complementary to column chromatography, there is an increasing number of advanced applications where FFF has shown superior performance. An increasing number of laboratories are using FFF complementary or alternatively to column chromatography, in particular when it comes to large, high molar mass and sensitive analytes. Another plus of FFF is its flexibility and versatility as different external fields (flow, thermal, sedimentation, electrical) can be used.
What would you like to see in new polymer characterisation systems?
In addition to developments in new and improved instrumentation, I would like to see analytical scientists to be more ‘adventurous’. With a huge number of different fractionation devices and advanced detectors at hand, method combinations should be used more frequently. Similar to comprehensive multidimensional chromatography, multidimensional FFF would be an interesting development. Even the combination of column- and channel-based fractionations in one device would be an interesting approach. It is the analytical problem that counts. And finally for fractionation – if the column does not work try a channel.
About Professor Harald Pasch
.jpg)
Harald Pasch is a Distinguished Professor at the Department of Chemistry and Polymer Science, University of Stellenbosch, South Africa. His research focuses on the development of advanced analytical methods for complex polymers. Pasch holds the SASOL Research Chair in Advanced Analytical Polymer Science. He is the author/co-author of more than 300 scientific papers and has promoted more than 50 postgraduate students (MSc and PhD). In 2013 he published the textbook ‘Multidimensional HPLC of Polymers’. His latest book ‘Advanced Separation Techniques for Polyolefins’ appeared in September 2014.
Disclaimer: The views expressed here are those of the interviewee and do not necessarily represent the views of AZoM.com Limited (T/A) AZoNetwork, the owner and operator of this website. This disclaimer forms part of the Terms and Conditions of use of this website.