The development of eco-friendly and energy-efficient technology is one of the most pressing needs of this century. Moreover, energy consumption on Earth is predicted to dramatically increase in the future, leading to a higher demand for novel energy supplies that must be safe, clean, and sustainable. A paper in the journal Energy Storage Materials considers the use of organic electrode materials.

Study: Artificial intelligence driven in-silico discovery of novel organic lithium-ion battery cathodes. Image Credit: nevodka / Shutterstock
Against this backdrop, organic electrode materials (OEMs) combine key sustainability and versatility properties with the potential to realize the next generation of truly green battery technologies. Organics offer a combination of attractive features such as being low cost and lightweight and having versatile synthesis methods, customizable properties, and production from renewable sources.
Therefore, the proper design of novel organic materials with enhanced properties is highly crucial for sustainable development. However, for OEMs to become a competitive alternative, challenging issues related to energy density, rate capability, and cycling stability must be overcome.
This study details the development of an efficient and elegant workflow combining density functional theory (DFT) and machine learning to accelerate the discovery of novel organic electroactive materials.
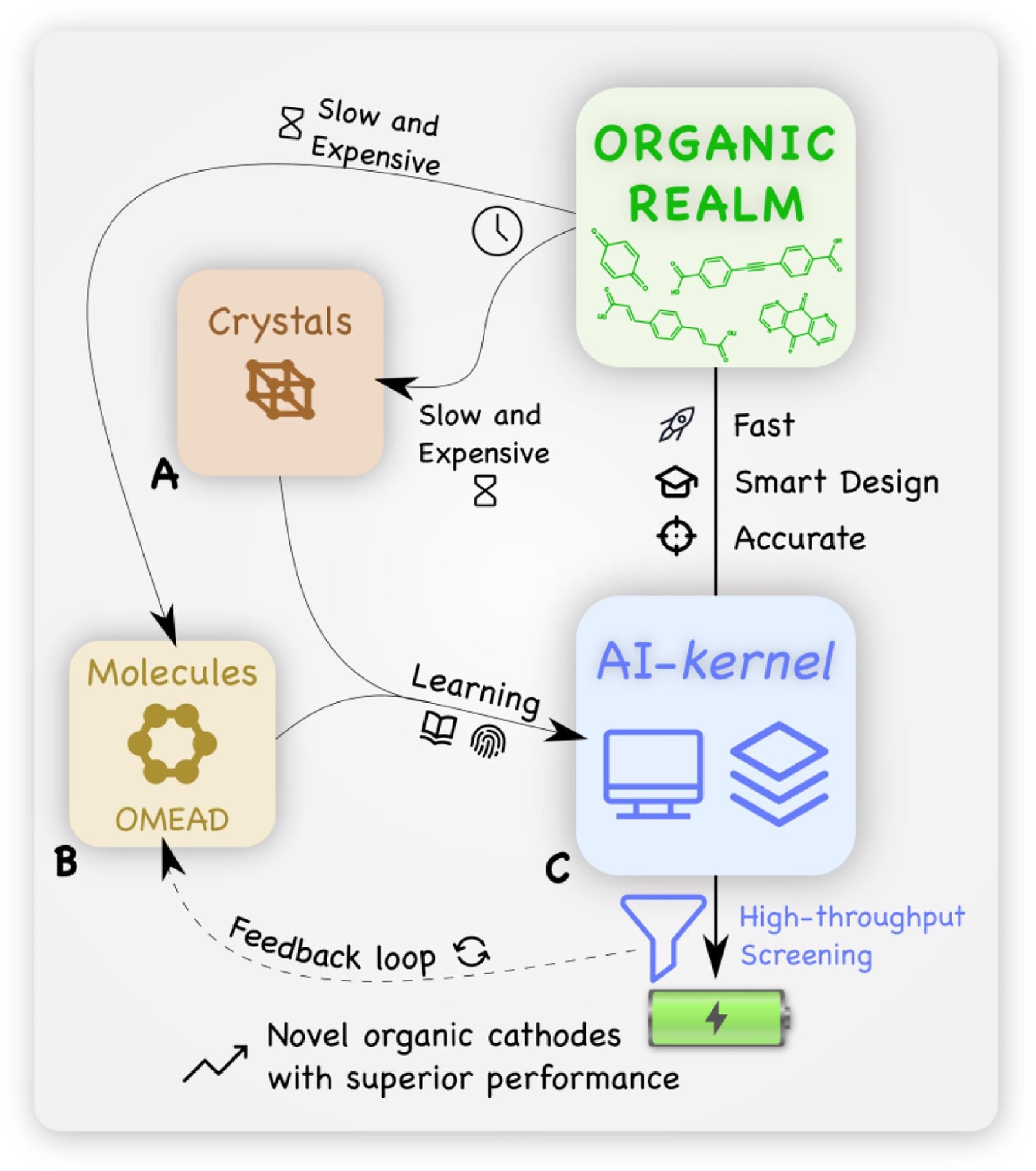
Flowchart illustrating the whole workflow of the developed framework and how the AI-kernel enables fast access to the world of organic materials after the learning step. OMEAD stands for “Organic Materials for Energy Applications Database.” Image Credit: Carvalho et al., 2021.
Method
The framework is divided into three key steps. Firstly, the crystal structures for a limited set of 28 electrode candidates and their corresponding lithiated phases were resolved by combining DFT and an evolutionary algorithm.
Secondly, a database containing structural information and properties of 26,218 organic molecules extracted from high-level DFT calculations was developed. Most of the organic moieties recently proposed for energy conversion and storage applications have been included.
Thirdly, models were developed based on machine learning methodologies to considerably accelerate the evaluation of the electrochemical properties of the OEMs. By combining data from the first and second steps, an efficient AI-kernel with good statistical fidelity was designed, which relies only on the knowledge of molecular structure as input to predict the battery open-circuit voltages, completely by-passing the time demanding ab-initio calculations.
Results and Discussion
The crystal structure for the molecules was predicted for their respective first two lithiated phases, and the average lithiation voltage (VOC) for a two-step reaction was calculated. Several molecules in this dataset are based on dicarboxylates because they initially form stable crystals. In addition, the dicarboxylate-based building blocks may be further customized by different mechanisms, thus offering tunable thermodynamic properties.
A common signature of these crystals is the formation of a salt layer intercalated by their organic counterpart. The Li-ions in this layer are usually surrounded by four carboxylate oxygens, which form tetrahedron coordination. This feature adds considerably to the general stability of these types of organic electrodes, a favorable property for lithium-ion batteries (LIBs).
A neural model was built by benchmarking different combinations of fingerprints and network architectures to generate the most effective model. The neural networks for all the Coulomb Matrix (CM) and Many-Body Tensor Representation (MBTR) combinations were coded on the TensorFlow framework, while the Simplified Molecular-Input Line-Entry System (SMILES) was developed on top of PyTorch.
The mean absolute error (MAE) was chosen as the training criteria for the networks when analyzing the general performance of the different fingerprints and architectures. The training was performed in a portion of the Organic Materials for Energy Applications Database (OMEAD) molecular database with 18,528 samples, while 2290 were reserved for testing purposes.
The SMILES representation achieved similar performance as the one for the MBTR—a fingerprint considerably more powerful and capable of encoding more structural information. Thanks to its conceptual elegance and simplicity, the SMILES architecture was the final choice.
With the neural model trained, the AI kernel was settled. The next step was to apply the framework in production to explore the organic universe and identify new potential electrodes for LIBs through a high-throughput screening approach.
To select potential candidates, a simple voltage filter was applied to identify cathodic compounds with VOC higher than 2.9 V (vs Li/Li+) and anodic compounds with VOC between 0.0 V and 0.5 V (vs Li/Li+).
The overall result presents a good agreement between DFT and AI, reasserting the model’s performance. Small deviations are chiefly due to outliers mostly from molecules that went through major structural changes over the redox process in the DFT calculations.
The realization of such improved materials could place organic-based batteries in a desirable position as a next-generation technology for energy-demanding applications where the combination of high gravimetric energy density and battery sustainability is crucial.
Conclusion
The AI-kernel discussed in this study has enabled a high-throughput screening of a huge library of organic molecules, resulting in the discovery of 459 novel potential OEMs, with the candidates offering the potential to achieve theoretical energy densities beyond 1000 W h kg−1.
Moreover, the machinery accurately identified common molecular functionalities that result in such higher-voltage electrodes and pinpointed an interesting donor-accepter-like effect that could power the future design of cathode-active OEMs.
Journal Reference:
Carvalho, R. P., et al. (2021) Artificial intelligence driven in-silico discovery of novel organic lithium-ion battery cathodes. Energy Storage Materials. https://www.sciencedirect.com/science/article/pii/S240582972100489X