In a recent study published in the journal Applied Materials Today, researchers from China developed a facile method to fabricate smart multi-responsive shape and color memory materials using poly(ε-caprolactone) (PCL)-based dynamic polymer network, which was programmed in specific shapes at the macroscale and microscale level.
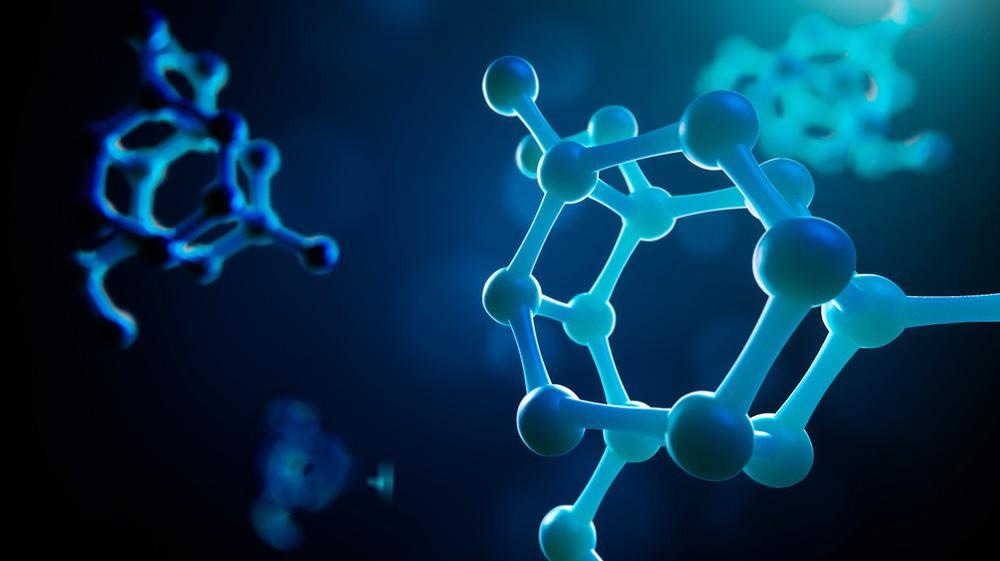
Study: Multiscale shape-memory effects in a dynamic polymer network for synchronous changes in color and shape. Study: Egorov Artem/Shutterstock.com
The researchers used low-cost compact disks (CDs) as a photonic grating nanostructure and imprinted it on the PCL-based network via nanoimprinting and plastic reconfiguration to achieve both temporary and permanent shaping.
As both macroscale and microscale shapes were controlled by a single molecular stimulus, on the application of heat, they recovered simultaneously. The recovery of microscale shapes also led to the recovery of the color of the polymer network. This shape and color memory polymer network can be used for information encryption and transfer platforms.
Shape and Color Memory Materials
Shape memory materials (SMMs) are a class of smart materials, which return to their initial shapes when exposed to external stimuli such as heat, light, electric charge, magnetic field, and many more. Thus, it would be appropriate to say that they can memorize their initial shapes. They are commonly used in bionic soft actuators and robots capable of responsive behaviors. Generally, it is easy to achieve a single responsive behavior in a smart material, but the development of multi-responsive materials without inter-response interference is challenging.
The color-changing and memory materials have advanced artificial photonic structures that draw their inspirations from nature, such as chameleon and octopus’ skin, butterfly wings, beetle exoskeletons, and bird feathers with ordered periodic structures in the wavelength range of visible light.
Commonly used methods to fabricate photonic structures include nanoimprinting lithography, colloidal particle self-assembly, chemical deposition, electrochemical etching, and cholesteric liquid crystal self-assembly. Shape memory polymer (SMP) networks having photonic nanostructures demonstrate both shape memory and color memory by reversing the periodicity of photonic nanostructures when exposed to solvent, thermal stimulation, or force.
About the Study
In this study, researchers imprinted the photonic grating nanostructure of a blank CD onto the PCL-based polymer network via nanoimprinting and plastic reconfiguration. First, they synthesized PCL-diol by the typical bulk ring-opening polymerization of ε-CL using 1,4-butanediol (BDO) as an initiator and stannous octoate (Sn(Oct)2) as the catalyst.
More from AZoM: The Role of Pellet Extruders in 3D Printing
After that, PCL-diacrylate (PCLDA) was synthesized by solution casting of the mixture of PCL-diol, anhydrous 1,2- dichloroethane, 2-isocyanatoethyl acrylate, and dibutyltin dilaurate (DBTDL). The final NW-PCLDA- trimethylolpropane tris(3-mercapto propionate) (TMPMP)- diazabicyclo [4.3.0] non-5-ene (DBN) (NPT x-D) polymer network was synthesized by mold drying mentioned chemicals.
Subsequently, blank CDs were used as diffraction pattern templates to obtain a photonic structure master. Polydimethylsiloxane (PDMS) prepolymer and a crosslinker were mixed and poured onto the prepared master followed by curing at 80 °C for 2 hours and peeled off to obtain patterned PDMS mold. Finally, the photonic structures were programmed on to NPTx-D polymer network using a prepared patterned PDMS template in a hot compression mold.
Observations
Differential scanning calorimetry (DSC) results showed the PCLDA linear polymer indicated expected crystalline and melting behavior between the crystallization temperature and melting temperature at 29.6 and 52.0 °C, respectively. Moreover, the crystallization temperature and crystallization enthalpy of the NPTx-D networks decreased with an increase in the content of the TMPMP crosslinker as it severely interrupted the regularity of the PCL segment and inhibited the crystallization.
The neutralized organic base, DNB, induced thermal plasticity in the crosslinking network. The isostrain stress-relaxation test revealed that all NPTx-D samples showed faster stress-relaxation speeds at higher thermal plastic temperatures, and the stress relaxation performance was highly dependent on the degree of crosslinking and amount of TMPMP crosslinker.
The dynamic mechanical analysis (DMA) showed the storage modulus decreased sharply when the temperature crossed the melting temperature of the PCL segment. However, the storage modulus decreased slightly when the temperature was increased further due to the exchange of dynamic bonds.
The materials were easy to be imprinted at the temperatures of temporary and permanent grating at 70 and 130 °C, respectively. The crystallinity of the PCL segment acted as a single switching point for both macroscale level and microscale level shape and color memory behavior of the NPTx-D polymer network. Also, the plasticity was effectively suppressed under the conditions used for the elastic shape-memory experiments.
Conclusions
To summarize, the researchers developed a synchronous shape and color memory polymer network using PCL polymer and imprinted the shape-color memory pattern onto the NPTx-D polymer network using simple low-cost CDs containing photonic grating nanostructures. They found that the crystallinity of the PCL segment acted as a single switching point for both the shape and color memory behavior of the polymer network. This new polymer shape-color memory polymer can be used for information encryption and transfer platforms.
Reference
Xu, Z., Li, L., Du, L., Wang, L., Shi, L, Yang, K., Wang, Y., Multiscale shape-memory effects in a dynamic polymer network for synchronous changes in color and shape, Applied Materials Today, 2021, 101276, ISSN 2352-9407, https://www.sciencedirect.com/science/article/pii/S2352940721003395?via%3Dihub
Disclaimer: The views expressed here are those of the author expressed in their private capacity and do not necessarily represent the views of AZoM.com Limited T/A AZoNetwork the owner and operator of this website. This disclaimer forms part of the Terms and conditions of use of this website.