New breakthroughs have been made by a research group headed by Tao Sun, an associate professor of materials science and engineering at the University of Virginia. It has the potential to expand additive manufacturing in aerospace and other industries that depend on powerful metal parts.
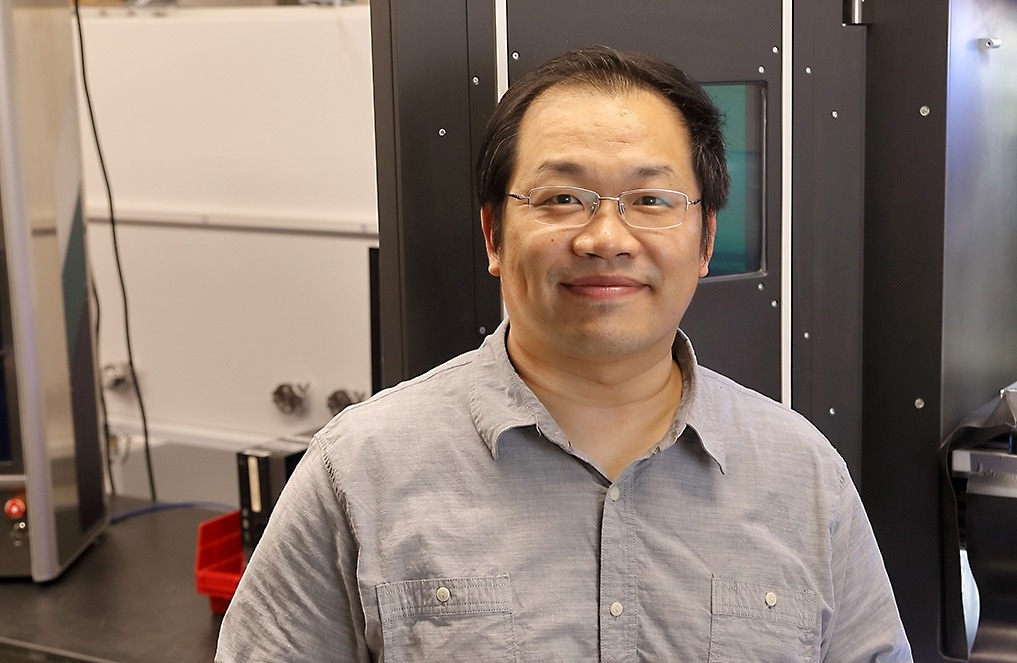
UVA associate professor Tao Sun in his lab at the University of Virginia. Image Credit: Tom Cogill for UVA Engineering.
Their peer-reviewed paper was reported on January 6th, 2023, in Science Magazine.
It meets the problem of detecting the formation of keyhole pores, considered to be one of the significant defects in a common additive manufacturing method known as laser powder bed fusion or LPBF.
Having been introduced in the 1990s, LPBF utilizes lasers and metal powder to 3D-print metal parts. However, porosity defects remain a difficulty for fatigue-sensitive applications like aircraft wings. Few porosities have been linked to deep and narrow vapor depressions, known as keyholes.
The keyhole’s formation and size are known as a function of scanning velocity and laser power, as well as the capacity of the materials to absorb laser energy. If the keyhole walls tend to be stable, it improves the encircling material’s laser absorption and also helps in improving laser manufacturing efficiency.
But if the walls seem to be wobbly or collapse, the material solidifies next to the keyhole, thereby trapping the air pocket present inside the newly developed material layer. This makes the material highly brittle and more probable to break under environmental stress.
Sun together with his group, such as Materials Science and Engineering Professor Anthony Rollett from Carnegie Mellon University and Mechanical Engineering Professor Lianyi Chen from the University of Wisconsin-Madison, came up with a method to detect the exact moment when a keyhole pore gets developed during the printing process.
By integrating operando synchrotron x-ray imaging, near-infrared imaging, and machine learning, our approach can capture the unique thermal signature associated with keyhole pore generation with sub-millisecond temporal resolution and 100% prediction rate.
Tao Sun, Associate Professor, Materials Science and Engineering, The University of Virginia
In expanding their real-time keyhole detection technique, the scientists also progressed the way an advanced tool—named operando synchrotron X-Ray imaging—could be utilized. Using machine learning, they further discovered two modes of keyhole oscillation.
“Our findings not only advance additive manufacturing research, but they can also practically serve to expand the commercial use of LPBF for metal parts manufacturing,” stated Rollett. Rollett is also the co-director of the Nextmanufacturing Center at CMU.
Porosity in metal parts remains a major hurdle for wider adoption of LPBF technique in some industries. Keyhole porosity is the most challenging defect type when it comes to real-time detection using lab-scale sensors because it occurs stochastically beneath the surface.
Tao Sun, Associate Professor, Materials Science and Engineering, The University of Virginia
Sun added, “Our approach provides a viable solution for high-fidelity, high-resolution detection of keyhole pore generation that can be readily applied in many additive manufacturing scenarios.”
The group’s research has been financially supported by the Department o0066 Energy’s Kansas City National Security Campus managed by Honeywell FM&T.
Journal Reference:
Ren, Z., et al. (2022) Machine learning–aided real-time detection of keyhole pore generation in laser powder bed fusion. Science. doi.org/10.1126/science.add4667.