In today's industries, quality inspection in semiconductor manufacturing is critical. Many traditional fault detection and diagnosis techniques have been developed to determine the existence of trends or patterns in the process or whether a specific process variable behaves normally.
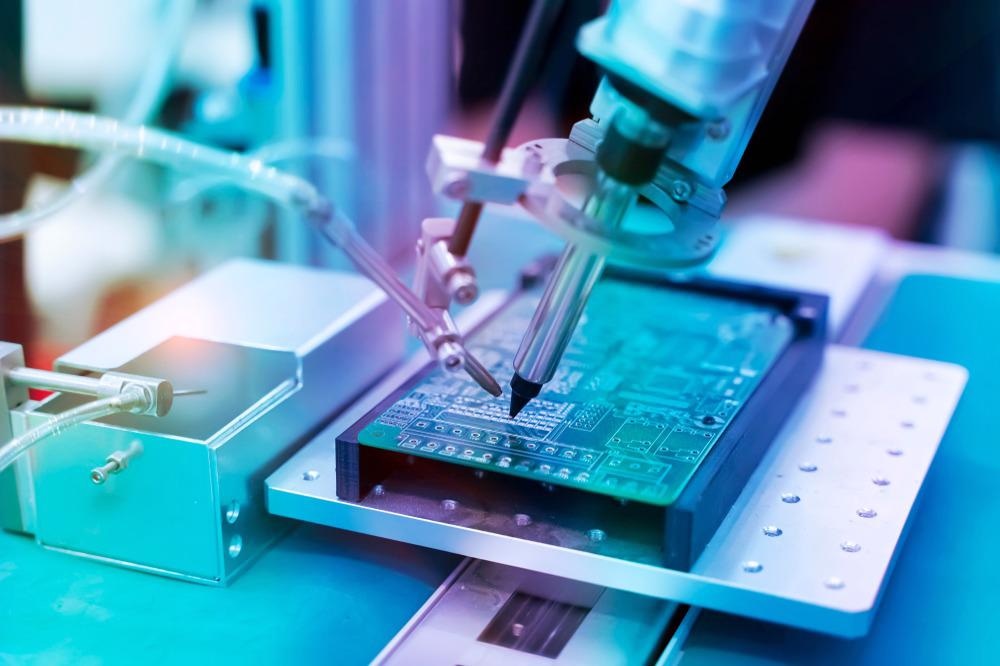
Image Credit: asharkyu/Shutterstock.com
Due to underlying process dynamics, these fault detection methods may occasionally fail to produce several hidden process characteristics or detect faults in processes.
In this article, we will discuss fault detection, the semiconductor etching process, and some novel approaches to overcome current challenges in effective fault detection methodologies.
What is Fault Detection in Engineering?
Detecting, isolating, and recovering faults is a branch of control engineering concerned with monitoring a system, determining when a fault has occurred, pinpointing the nature and location of the defect, and then taking appropriate action to prevent future failures or unfavorable accidents. In high-cost and safety-sensitive processes, fault detection is vital as early detection prevents the occurrence of unforeseen circumstances.
Fault detection and diagnosis have piqued the interest of many industrial sectors to improve many process performances.
Semiconductors and their Uses
Semiconductors are materials that can behave both as a conductor and an insulator depending on the situation in which it is needed. Generally, as the temperature rises, the resistivity of the material decreases.
Semiconductors are used in the production of diodes, transistors, and integrated circuits, among other electronic devices where they are intended to manage and control current flow. Semiconductors are used extensively due to their reliability, power efficiency, low cost, and compactness. Examples of semiconductors include silicon and gallium arsenide.
What is Etching and Why is Semiconductor Etching Important?
Etching is a chemical or electrolytic process that is applied after metallographic grinding and polishing to increase the contrast on surfaces, making it possible to see the microstructure or macrostructure. It can also be used to remove the thin, highly deformed layer formed during grinding and polishing processes.
Etching, as used in semiconductor device fabrication, is used to describe any technology that selectively removes material from a thin film on a substrate, with or without preexisting structures on its surface to create a pattern of that material on the substrate.
Etching is generally grouped into wet and dry etching. Dry and wet etching procedures are both effective for removing surface materials and creating patterns on surfaces. Wet etching differs from dry etching in that it employs liquid chemicals or etching agents, whereas dry etching employs plasmas or etching gases.
Some types of semiconductor etching processes are isotropic radical etching, reactive ion etching, physical sputtering, and ion milling.
Fault Detection Methodologies During the Etching Process
We will discuss some cases where attempts have been made to improve fault detection systems.
As previously mentioned, detecting faulty processes, identifying anomalies as they occur, and quickly classifying the root cause of the fault is critical for maximizing equipment utilization in today's semiconductor manufacturing; however, tool data acquired from production equipment contains a wealth of information that is frequently difficult to analyze due to its sheer volume and complexity.
In an attempt to make better use of this data, Hong et al., in a study published in IEEE Transactions on Semiconductor Manufacturing, proposed a modular neural network model as a method that can be used for fault detection in plasma etching.
The Dempster-Shafer theory of evidential reasoning was used to demonstrate successful fault detection at the subsystem level with zero missed alarms, and the classification for determining the root cause of the fault was presented in the chamber leak fault simulation. The researchers realized that combining various related information and incorporating engineering expert knowledge could result in a successful fault detection system.
In another case, to increase production yields and reduce wafer yield losses due to misprocessing, more efficient fault detection during the plasma process is desired. Process faults can be caused by incorrect equipment conditions, and performance drifts of parts or components of complex semiconductor fabrication equipment are often not recognized but can eventually lead to changes in the plasma conditions.
In a very recent study published in the journal Electronics, the researchers propose using optical emission spectroscopy data to improve the stability and accuracy of process fault detection.
The extended isolation forest approach was used to detect anomalies in optical emission spectroscopy data under a controlled experimental setup of arbitrarily induced fault scenarios, and it outperformed the conventional isolation forest method regarding accuracy and speed.
The researchers also used optical emission spectroscopy data to generate features related to electron temperature and discovered that combining electron temperature features with equipment status variable identification and optical emission spectroscopy data improved the prediction accuracy of process/equipment fault detection by up to 0.84 %.
A Deep Learning Methodology for Fault Detection
Researchers have also leveraged recent advances in technology to improve fault detection processes. In another study published in the journal IEEE Transactions on Semiconductor Manufacturing, the researchers propose a deep learning-based domain adaptation method for fault diagnosis in semiconductor manufacturing.
Intelligent data-driven condition monitoring methods have been successfully developed and applied in industrial applications in recent years. Despite the promising performance of condition monitoring, existing methods generally assume that the training and testing data are from the same distribution.
In practice, because of variations in the manufacturing process, the collected data are typically subject to different distributions in different operating conditions, which significantly degrades the performance of data-driven methods.
The researchers attempt to solve this problem by proposing a method that offered an effective and generalized data-driven fault diagnosis approach for quality inspection based on experimental results on a real-world semiconductor manufacturing dataset.
In a nutshell, fault detection is increasingly important in semiconductor manufacturing. Traditional approaches for fault detection face challenges in detecting all fault characteristics. Researchers are encouraged to leverage advances in technology to develop sophisticated algorithms that can detect patterns more efficiently and produce scalable and generalizable fault diagnosis approaches.
More from AZoM: A Review of Semiconducting Direct Thermoelectric Gas Sensor Techniques
References and Further Reading
Abbasi, I. (2022). Etch Technology Used in Semiconductor Manufacturing [Online]. AZoM.com. Available at: https://www.azom.com/article.aspx?ArticleID=21487
Azamfar, M., Li, X. and Lee, J. (2020). Deep learning-based domain adaptation method for fault diagnosis in semiconductor manufacturing. IEEE Transactions on Semiconductor Manufacturing, 33(3), pp.445-453. https://ieeexplore.ieee.org/document/9096390
Hong, S.J., Lim, W.Y., Cheong, T. and May, G.S. (2011). Fault detection and classification in plasma etch equipment for semiconductor manufacturing. IEEE Transactions on Semiconductor Manufacturing, 25(1), pp.83-93. https://ieeexplore.ieee.org/document/6074955
Kwon, H. and Hong, S.J. (2022). Use of Optical Emission Spectroscopy Data for Fault Detection of Mass Flow Controller in Plasma Etch Equipment. Electronics, 11(2), p.253. https://doi.org/10.3390/electronics11020253
Park, Y.J., Fan, S.K.S. and Hsu, C.Y. (2020). A review on fault detection and process diagnostics in industrial processes. Processes, 8(9), p.1123. https://doi.org/10.3390/pr809112
Disclaimer: The views expressed here are those of the author expressed in their private capacity and do not necessarily represent the views of AZoM.com Limited T/A AZoNetwork the owner and operator of this website. This disclaimer forms part of the Terms and conditions of use of this website.