A self-organized network of nanowires resembles a set of “Pick Up Sticks” and simulates how synapses work in the brain. In this experiment, a network was trained to retrieve learned and memorized dynamic online data.
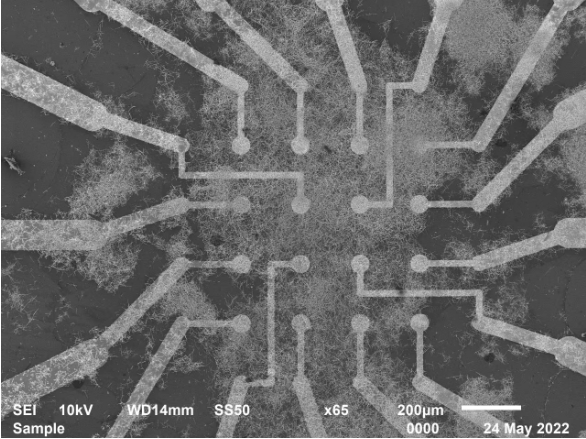
Electrodes interact with the nanowire neural network at the heart of the chip. Image Credit: University of Sydney
It has been successfully demonstrated for the first time that a physical neural network can learn and remember “on the fly,” in a manner that is modeled after and similar to the functioning of brain neurons.
The outcome creates a path for the development of low-energy, effective machine intelligence for increasingly difficult, real-world learning and memory tasks.
The research, which was published in Nature Communications, was done in conjunction with researchers from the University of California, Los Angeles, and the University of Sydney.
The findings demonstrate how brain-inspired learning and memory functions using nanowire networks can be harnessed to process dynamic, streaming data.
Ruomin Zhu, Study Lead Author and PhD Student, University of Sydney
Nanowire networks consist of minuscule wires with a diameter of just billionths of a meter. The wires self-organize into patterns that resemble the children’s game “Pick Up Sticks,” simulating neural networks similar to those found in the brain. Certain information processing tasks can be carried out with the aid of these networks.
Simple algorithms that react to variations in electronic resistance at junctions where the nanowires overlap are used to accomplish memory and learning tasks. This feature, called “resistive memory switching,” is produced when electrical inputs experience conductivity changes, much like synapses in the brain.
Inspired by the way the human brain processes information, researchers in this study used the network to detect and retain sequences of electrical pulses related to images.
Professor Zdenka Kuncic, who oversees the research, compared the memory test to having to recall a phone number. Additionally, the network was utilized to retrieve images from the MNIST library of handwritten digits, a collection of 70,000 tiny greyscale images used in machine learning, to complete a benchmark image identification position.
Our previous research established the ability of nanowire networks to remember simple tasks. This work has extended these findings by showing tasks can be performed using dynamic data accessed online.
Zdenka Kuncic, Professor, Faculty of Science, University of Sydney
Kuncic added, “This is a significant step forward as achieving an online learning capability is challenging when dealing with large amounts of data that can be continuously changing. A standard approach would be to store data in memory and then train a machine-learning model using that stored information. But this would chew up too much energy for widespread application. Our novel approach allows the nanowire neural network to learn and remember ‘on the fly’, sample by sample, extracting data online, thus avoiding heavy memory and energy usage.”
According to Mr. Zhu, there are further benefits to information processing online.
Zhu added, “If the data is being streamed continuously, such as it would be from a sensor for instance, machine learning that relied on artificial neural networks would need to have the ability to adapt in real-time, which they are currently not optimized for.”
In this study, the nanowire neural network demonstrated a machine learning capability benchmark, properly detecting test images with a score of 93.4%. Recalling up to eight-digit sequences was required for the memory task. Data was fed into the network for both tasks to show how memory improves online learning and to show the network’s capability for online learning.
Adam Stieg, James Gimzewski, and Zdenka Kuncic work at Emergentia Inc. Research scholarships are provided by the University of Sydney to Ruomin Zhu and Alon Loeffler. Zdenka Kuncic acknowledges the Australian-American Fulbright Commission for its assistance.
Journal Reference
Zhu, R., et al. (2023) Online dynamical learning and sequence memory with neuromorphic nanowire networks. Nature Communications. doi:10.1038/s41467-023-42470-5
Article Revisions
- Jan 6 2025 - Meta Description has been updated to fall inline with Google recommendations and a malformed link has been repaired.